One of the most frequent pain points for qualitative researchers?
The time it takes to manually code, analyze and synthesize huge amounts of data from calls, focus groups, interviews, or even open ended surveys.
It’s often described as…
Tedious. Time-consuming. Drowning. Manual. Overwhelming.
This is why we need AI qualitative data analysis.
Even though we love the puzzle of de-coding people’s behavior and needs, sometimes it just feels like too much information to make sense of in a couple of hours or days. Especially with tight deadlines and demanding stakeholders, researchers often don’t have hours (or days) to spend re-reading transcripts, sorting through scrappy notes, and coding or tagging data in Miro boards and excel sheets.
Luckily, we’re on the verge of an exciting wave in technology. According to the 2023 State of User Research Report, 20% of researchers are currently using AI for research, with another 38% planning to join them in the future. Key use cases include AI qualitative data analysis, AI-supported discussion guide generation, re-writing reports with AI, and ideation with AI.
While AI is not perfect, it is an amazing assistant to help you extract, organize and qualitatively code or theme your data so you can find insights faster.
Skeptical? Just try this AI-powered research assistant (free for 15 days!), and get back to us.
In this article, we’ll look at:
If you’re manually analyzing data, you’re using Post-Its and whiteboards or a digital version of the same like Miro or Mural. The manual process involves extracting relevant data, tagging or grouping it and then reviewing insights at the end.
If you’re analyzing user interviews, usability tests or focus groups for example, you can re-listen to your calls to make notes, or rely on ones taken during the interview. Usually folks add their notes to an excel sheet or Miro board and start looking for patterns across calls there.
For an in-depth walk through of how you can run qualitative data analysis manually, check out these pieces:
- How to analyze user interviews
- Many modes of research synthesis
- Affinity mapping: An ultimate guide
Pros of Manual Qualitative Data Analysis
A lot of researchers like to do manual qualitative data analysis, despite the time and labor it requires. Here are a few reasons why.
1. You have complete control over the data: Some researchers believe that you need to fully immerse yourself in the data for real inspiration and insights. Manual research analysis is perfect for that. By reading and organizing all the data segments yourself, you understand the contours of the research better, as well as your users.
2. It’s easy to learn and execute: You don’t have to spend time figuring out a fancy new tool. All the labeling and notes can be conquered with Post-its, paper or a simple whiteboard tool.
3. You can make it collaborative: On a whiteboard or Miro board, you can bring in teammates or clients to analyze data for you—discussing each data point and labeling or grouping it based on consensus. This allows you to get buy-in from stakeholders and take their perspective into account when discovering insights.
Cons of Manual Qualitative Data Analysis
While Thematic Analysis is really powerful, as we know—it has 4 major drawbacks:
1. It’s very time-consuming: If your research has a deadline in place, manual coding is a lot to take on. For academic projects with longer timelines, there should be room for it. But if you’re working at a company or agency—you have a few days or weeks to turn insights around.
2. It’s insanely difficult to scale: You know that feeling when you've had 10 hour-long interviews you need to go back though? Or over 100 pages of transcripts? It’s painfully exhausting to review and re-review all this data. For extensive datasets, manual qualitative tagging becomes laborious and inefficient. As the volume of data increases, so does the time required for coding.
3. It requires work and re-work: Just imagine reading all that data and transcripts and manually remembering to code and categorize everything. You begin with one set of codes—let’s say different issues based on customer feedback. Halfway through the reading, you realize that a new feedback point has recurred enough to be labeled separately. You’ll now have to revisit all your data again and make changes to your coding.
4. So. much. data. Since qualitative data is unstructured, you can very easy get overwhelmed and be drowning in recordings, transcripts, notes before you know it. Think about it—you’re trying to figure out common patterns from 500 open-ended survey responses, 200 pages of user interview transcripts, and the note-taker’s observations from watching users interact with the new feature. It’s a lot.
5. Bias: If it’s just you coding—bias can enter easily. With manual qualitative analysis, the themes that appear will be based on your subjective interpretation. Having AI to partner with can give you a different perspective to consider.
With the advent of Artificial Intelligence (AI), particularly through Natural Language Processing (NLP) and Generative AI, research analysis is going through a massive shift.
Sure, it’s not smarter than a person (yet). It cannot reason, and won’t be taking over any researcher jobs anytime soon. But it can incredibly speed up research, and help you skip the tedious parts.
This means that it can help researchers do their job 5x faster, by automating the manual, time-consuming, tedious parts. Researchers already swear by tools like Looppanel for making it 10x easier to go from data to research insights.
So how can we use AI for qualitative data analysis? Let's dig into 5 key use cases:
1. Replace your note-taker (I’m not kidding)
Finding a good note-taker for every call is tough.
It’s expensive, time-consuming, often just not even available. Luckily, note-taking is the kind of task AI is actually really good at.
AI qualitative data analysis tools can capture key parts of your call while keeping you in the driver’s seat. It’ll automatically highlight where questions were answered, summarize notes in a Q&A or theme based format.
Instead of spending days re-reading transcripts, you can review the notes, make sure they capture what you care about, and add additional context or tags as needed.
With a little help from AI-assisted note-taking, researchers can speed up the entire process, reduce dependence on team members and make sure they don’t miss anything from their interviews.
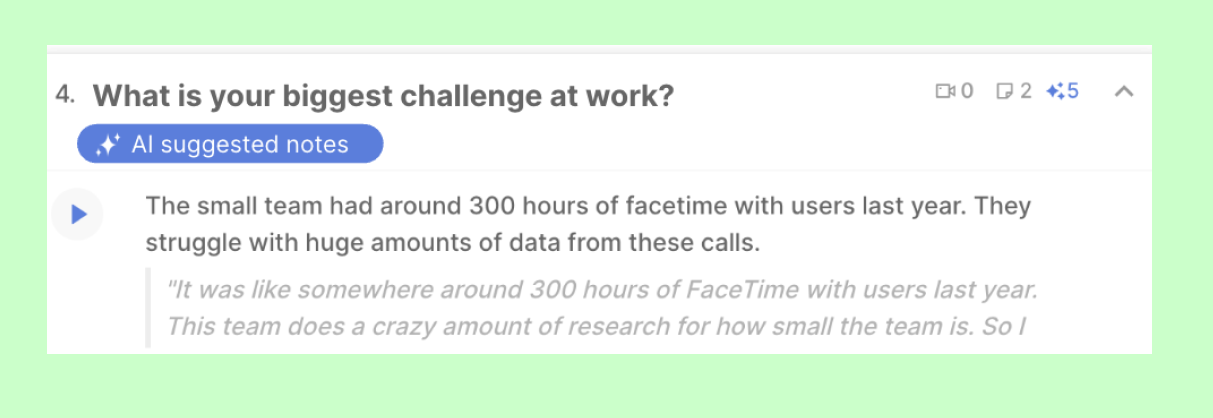
Looppanel’s AI-powered note-taker for example joins digital interviews on Google Meet, Zoom or MS Teams seamlessly to record, transcribe and take notes, leaving you free to focus on the conversation at hand.
After your call ends, you can review notes by the question they answer, review the transcript section they’re created from, or create shareable video clips to send to your team.
2. Generate super accurate transcripts in minutes
Quality of AI transcription across accents has improved substantially. The days of manually listening to and transcribing calls are over (at least in English!)
Stop wasting time correcting transcripts or paying for expensive human transcription—hop on the AI-assisted transcription bandwagon to see what’s possible.
While the quality of transcripts is superior in English, tools like Looppanel offer transcription in multiple languages like Hindi, German, French, Spanish, Portuguese, Dutch, and Italian.

3. Automatically tag your data
Instead of reading through all the pages of the transcript to identify key themes and codes, AI thematic analysis tools can do the first pass of grouping relevant data for you.
Of course, you want to still review the data, check that the themes tally with your intuition and context, but boy it’s so much easier dealing with small groups of data, versus 1000 random sticky notes (breaks into sweat at the thought 😅).
Some AI thematic analysis tools have started to offer AI-assisted tagging, which can cluster tags automatically for review. Although this technology is still evolving, it holds immense promise in streamlining data analysis processes.
4. Organize your data by question
Not only do great AI qualitative data analysis tools extract data for you, but they can group them by your interview questions for easy review.
Tools like Looppanel auto-organize notes based on your discussion guide or interview questions. Just toggle the topic you’re interested in exploring, and voila! The notes and highlights related to it are in one place.
Since answers are automatically extracted for each interview, you can easily view answers across calls as well.
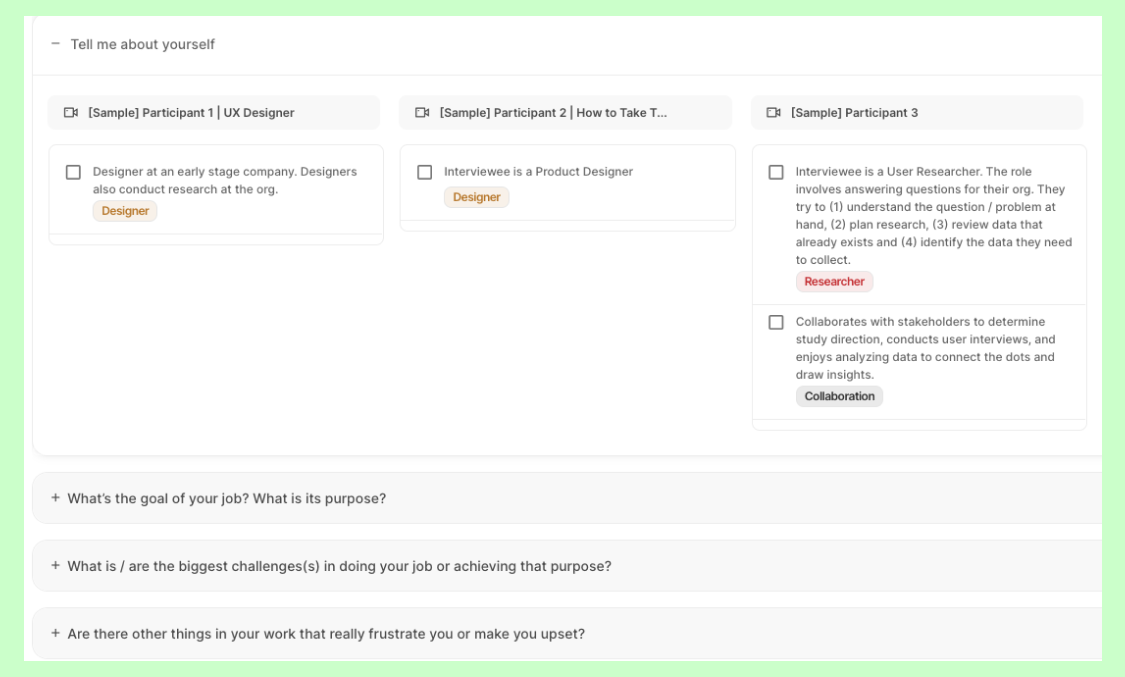
5. Gut check on bias
Subjective bias is a big risk with qualitative analysis. Standardizing AI qualitative coding, and providing a different perspective can be helpful. It’s like a super-smart, third party research assistant to cross-check all your interpretations, and offer a second opinion.
Of course, you want to be careful of any bias the AI may introduce as well! That's why it's important that you're not using a black-box AI that you feed data into and get an answer out of in one step. This output is very hard to cross-check without doing the analysis yourself. Use a software that helps you do the analysis faster and allows you to cross-check its output at every stage for the best outcome.
There's a range of tools to try out for AI qualitative data analysis—some are purpose-built for qualitative research, while others are general purpose tools you can use for analysis.
Here are top 3 picks with step-by-step guides on how you can leverage them for AI qualitative data analysis.
1. Looppanel
Looppanel is built like an AI-powered research assistant—it'll automate all the tedious, manual parts of the job you don't have time for. Key AI features include:
- Really good transcripts (across accents)
- Automatic notes organized by your interview questions
- Automatic tagging of data
- Google-like search across your workspace
If you're looking for a product that's easy to use and packages all the benefits of AI within your research workflow, look no further. Just sign up, paste in your discussion guide and upload your calls—Looppanel will do the rest!
Free Trial: Yes
Pricing: Starting $30 / month
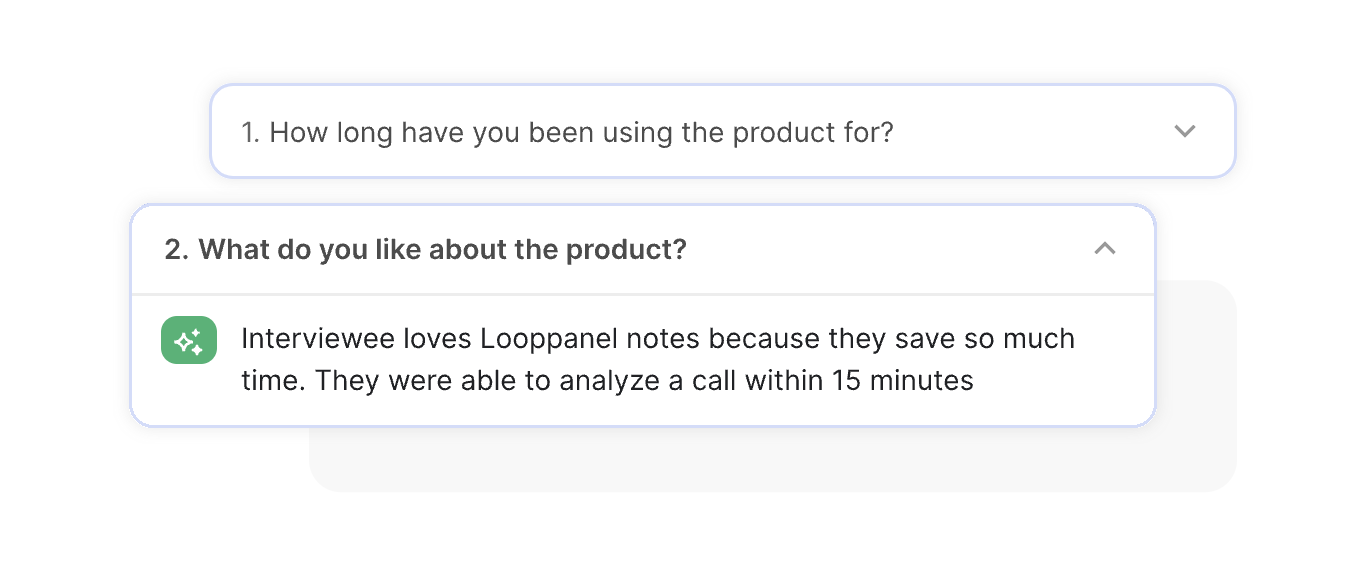
2. ChatGPT
ChatGPT is a very powerful AI model, but it's not built for research which means you have to adapt your analysis process to ChatGPT's limitations.
Can ChatGPT do qualitative data analysis?
TLDR: It can, but it takes some work.
For example, ChatGPT has a limit on the amount of text it can take in at a time—to use GPT for analysis, you'll need to break your transcript into smaller chunks to feed it to the product (not fun). It's otherwise incredibly helpful for work like content creeation, email marketing and coding websites, as done in digital marketing by link building agency.
Here are some limitations to keep in mind:
- Free versions of these models are often poorer in quality than paid ones.
- There are often limits to how much information general purpose AI chatbots can consume. If analyzing data, you may need to break your transcripts into smaller chunks to be able to analyze them (warning: this can be painful!).
- You'll need to spend time iterating on the prompts depending on the output you want (here's a list of 14 ChatGPT prompts to get started with!).
- They're not built for the research workflow, so any other actions you want to take (e.g., create a clip, tag a note), you'll have to do elsewhere.
What's the best way to use ChatGPT for AI qualitative data analysis?
4 steps:
- Get a really high quality transcript (or clean one up so it's 95%+ accurate).
- Break the conversation into small chunks (~2,500 characters each). You do this for two reasons: (1) ChatGPT has a prompt limit of4,096 characters, (2) ChatGPT hallucinates and it's helpful to be able to trace where a note / summary came from.
- Create a prompt for your use case (e.g., "Summarize what Participant 1 is saying in 3-5 bullet points"). Iterate on this based on the language, or output you want. Also add any important context on your company here.
- Feed ChatGPT each of your 2,500 word sections with the relevant prompt. Copy-paste the prompt sections and output text to a miro board or excel sheet for final analysis. Remember to check the output after every prompt! ChatGPT can hallucinate
Free Trial: Yes
Pricing: GPT 3.5 is free! The quality is far below GPT 4 though, which costs $20 / month
3. Atlas.ti
If you're working in an academic setting and need tons of customization to analyze your data, Atlas.ti is worth exploring.
The product is built more for manual analysis, relying heavily on coding transcripts by hand but has recently forayed into adding AI features to their toolstack.
Key AI features:
- AI chatbot that you can ask questions to. This will look through your transcripts for relevant data.
- AI qualitative coding the software automatically generates for you.
Free Trial: Yes
Pricing: Commercial license from $50 / month
4. MAXQDA
MAXQDA is the leading tool for qualitative and mixed methods research. While it's more complex than other options, it's perfect for academics and scientists requiring in-depth, detailed analysis.
Key AI features:
- MAXQDA’s AI Assist feature can converse with your data, prepare summaries and even find definitions for complex terms!
- It also offers quantitative analysis, visual mapping features and a comprehensive workspace.
Free Trial: Yes
Pricing: Custom plans depend on industry and usage requirements
Here’s what you need to avoid, if you are using AI-powered tools like Looppanel and ChatGPT for user research analysis and AI qualitative coding. Aka how NOT to use AI for qualitative data analysis!
1. AI is your assistant, it cannot replace you
AI supplements human expertise; it doesn't replace it entirely.
AI is your research assistant. It can help you code faster, but you still hold the context to interpret notes and clusters accurately.
You should check any AI-generated data and expect it to be a great starting point—not blindly take it as the final answer.
2. AI lacks context
AI tools completely lack worldly context, and don’t have access to a lot of data about your work. They will miss out on obvious points that you, with years of experience at your company, intuitively understand.
3. AI needs some supervision
You cannot feed an entire project’s worth of data to AI and expect fully formed final insights.
Well, you could—but you the odds that it could go sideways are high.
AI is not that smart (yet).
That’s why with Looppanel, we make sure AI output is always traceable and editable by you.
Because AI can make mistakes, you need to be able to check where this note came from, edit a cluster, or add your own takeaways manually.
4. Don’t expect AI to answer the whys
AI is good at spotting patterns in user behavior, like a detective sifting through clues. However, it struggles to grasp the "why" behind those behaviors. That's because human actions are influenced by a colorful mix of personal preferences, cultural norms, and situational factors. AI might tell you what users are doing but unraveling the why remains a puzzle it can't fully solve.
So, limit its role to just observing the user behavior and pointing out the patterns and themes within. You will have to do the analysis and making sense of the whys and how this affects your organization.
4. Feed it all the necessary data, as accurately as possible
Imagine AI as the brightest student in class, who answers your questions. But it doesn’t have the ability to answer anything outside the syllabus.
AI’s excellence depends on the data you give it. If your data is incomplete, inaccurate, or skewed, well, it's like giving it an incomplete set of notes for an exam. It might not provide the answers you're hoping for, simply because it doesn't have the full context.
5. Data security and compliance is a concern
When signing up to use AI qualitative data analysis tool, check the terms and conditions for their data security measures. Being brand new territory, legal and ethical regulations for AI tools are still catching up.
Do a quick check for the data security measures implemented, GDPR compliance etc.
For example, at Looppanel here are some of the security measures we take:
- 🔐 Data is encrypted and stored securely
- ❌ We only use summarization models that do not use your data for training purposes
- 🛡️ GDPR compliance for data privacy
If you have questions about Looppanel’s data security measures or what you should watch out for in terms of security, you can always reach out to us at support@looppanel.com.
FAQ
What is Qualitative Data Analysis (QDA)?
Qualitative data analysis is the process of analyzing qualitative data (usually interviews recordings / transcripts / notes, written feedback, etc.) to identify patterns, themes or insights. Qualitative data analysis often requires thematic analysis / qualitative coding (labeling your data points with themes) and affinity mapping (clustering data based on these themes to find patterns).
What are the 5 methods to analyze qualitative data?
There are 5 common methods of analyzing qualitative data: content analysis, narrative analysis, discourse analysis, thematic theory analysis, and grounded theory analysis. In this article, we'll mainly focus on thematic analysis—how you can do this manually, or with an AI qualitative data analysis tool.
What are some alternatives to Manual Qualitative Coding?
There are a couple of ways to fast-forward through manual qualitative coding.
You can always get a live note-taker (or be one yourself)! This is not the easiest, of course. You need another person on your team’s time, availability, and they need to be good at taking notes. Tough trifecta. If you’re at an agency, this could affect budgets on projects as well.
The easier route? AI qualitative data analysis software. There’s a new era of tech AI-powered tools out there, ready to help and do the grunt work you don’t want to. It’s like having a research assistant available 24/7.
Can AI do thematic analysis?
Yes, AI qualitative data analysis tools can perform thematic analysis, offering new possibilities for qualitative research. AI for qualitative data analysis can process large volumes of data, such as interview transcripts or open-ended survey responses, to identify recurring patterns or themes. These AI qualitative research systems use natural language processing and machine learning algorithms to recognize common words, phrases, and concepts across multiple data points.
While AI qualitative data analysis can quickly categorize and group similar ideas, it's important to note that it may miss nuanced or context-dependent themes that a human analyst might catch.
Can AI be used for data analysis?
Absolutely, AI qualitative research tools have become powerful assets for data analysis across various fields. AI for qualitative data analysis excels at processing and analyzing large datasets quickly and efficiently, identifying patterns and correlations that might be challenging for humans to detect.
In the realm of AI qualitative data analysis, these tools can assist with tasks such as text classification, sentiment analysis, and topic modeling. They can help researchers sort through vast amounts of unstructured data, such as social media posts, customer reviews, or interview transcripts, to extract meaningful insights.
While AI qualitative research tools can handle the heavy lifting of data processing and initial pattern recognition, it's crucial to remember that human expertise is still essential for interpreting results, understanding context, and drawing meaningful conclusions. The most effective approach often combines the processing power of AI for qualitative data analysis with human critical thinking and domain knowledge.
AI Qualitative Data Analysis with Looppanel
Ready for a super-smart AI assistant to help with qualitative analysis? We have the perfect one for you.
Looppanel is an AI-powered research repository tool that can:
- Transcribe calls in minutes with over 95% accuracy
- Take notes for you during interviews
- Auto-tag your data for affinity mapping
- Smart search (find everything you know about “why users churn”, even if you didn’t tag it)
You don’t have to believe me.
Try it out for FREE, and see how you feel!
This is part of a new Looppanel blog series on AI and UX Research. For more on AI Qualitative Research, read the rest of the series of articles on AI for UX Design & Research, and ChatGPT Prompts for faster research.