Ever tried to organize your Netflix watchlist? Hundreds of shows and movies, each with different genres, ratings, and viewing histories. Now imagine doing that with research data - thousands of interview responses, observations, and field notes. That's where framework analysis steps in. Like Netflix's recommendation algorithm making sense of viewing patterns, framework analysis helps researchers organize and interpret complex qualitative data systematically.
In this guide, we'll show you how to transform overwhelming data into clear, actionable insights - whether you're studying patient experiences in healthcare or analyzing workplace culture.
No more drowning in data. Let's dive in.
What is framework analysis in qualitative research?
Framework analysis is research method that systematically sorts, organizes, and interprets textual information. It was specifically created to address the needs of applied policy research, offering a systematic and transparent approach to managing and analyzing qualitative data. Since its development, it has been widely adopted across multiple fields, including healthcare, education, and market research.
What are the five steps of framework analysis?
Framework analysis is performed in five stages to provide a clear, systematic approach to qualitative data. Each steps build upon the previous one by offering researchers a structured pathway to transform raw data into insights while maintaining transparency…
Step 1: Familiarization with data
This foundational stage involves immersing yourself in the data through deep engagement with all available materials. Researchers spend time reading and re-reading transcripts, carefully reviewing field notes and contextual information, and when available, listening to audio recordings to capture nuances that might be missed in text alone.
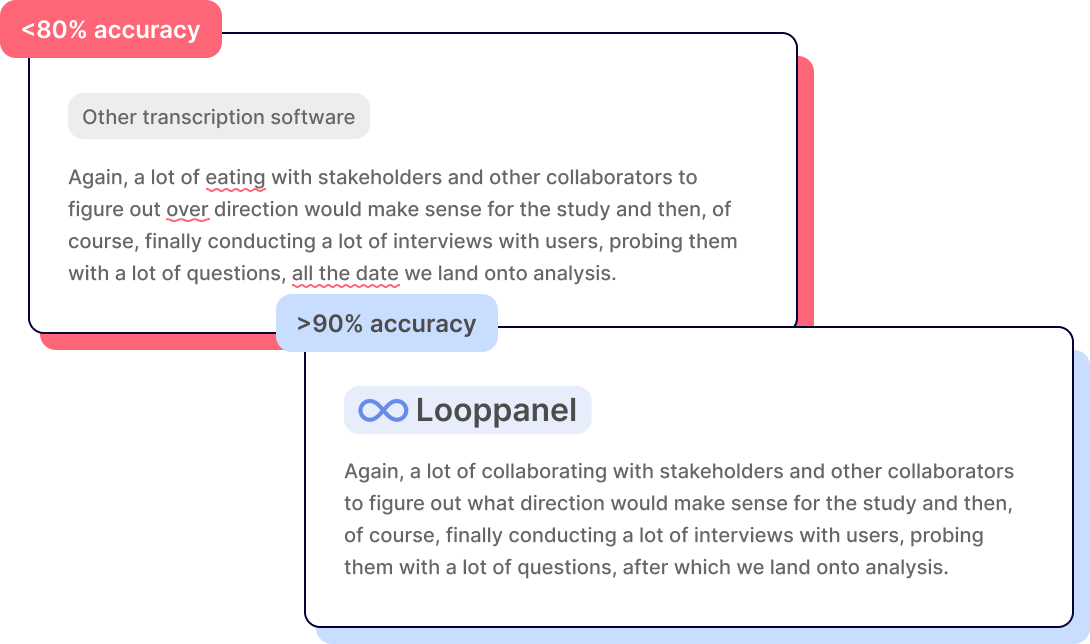
During this process, researchers begin making initial notes about key ideas and recurrent themes they observe, while also documenting their early analytical observations. Here, transcription services from modern tools like Looppanel can help convert audio to text efficiently. This hands-on immersion helps develop a thorough understanding of the data landscape, setting the foundation for more detailed analysis to come.
Step 2: Identifying the thematic framework
Once you understand the data with AI transcription, developing a thematic framework is next. It involves defining the categories or themes that connect with your research questions. It begins with reviewing your initial notes and identifying broad categories of themes. These serve as the foundation for organizing and refining the data as new patterns emerge.
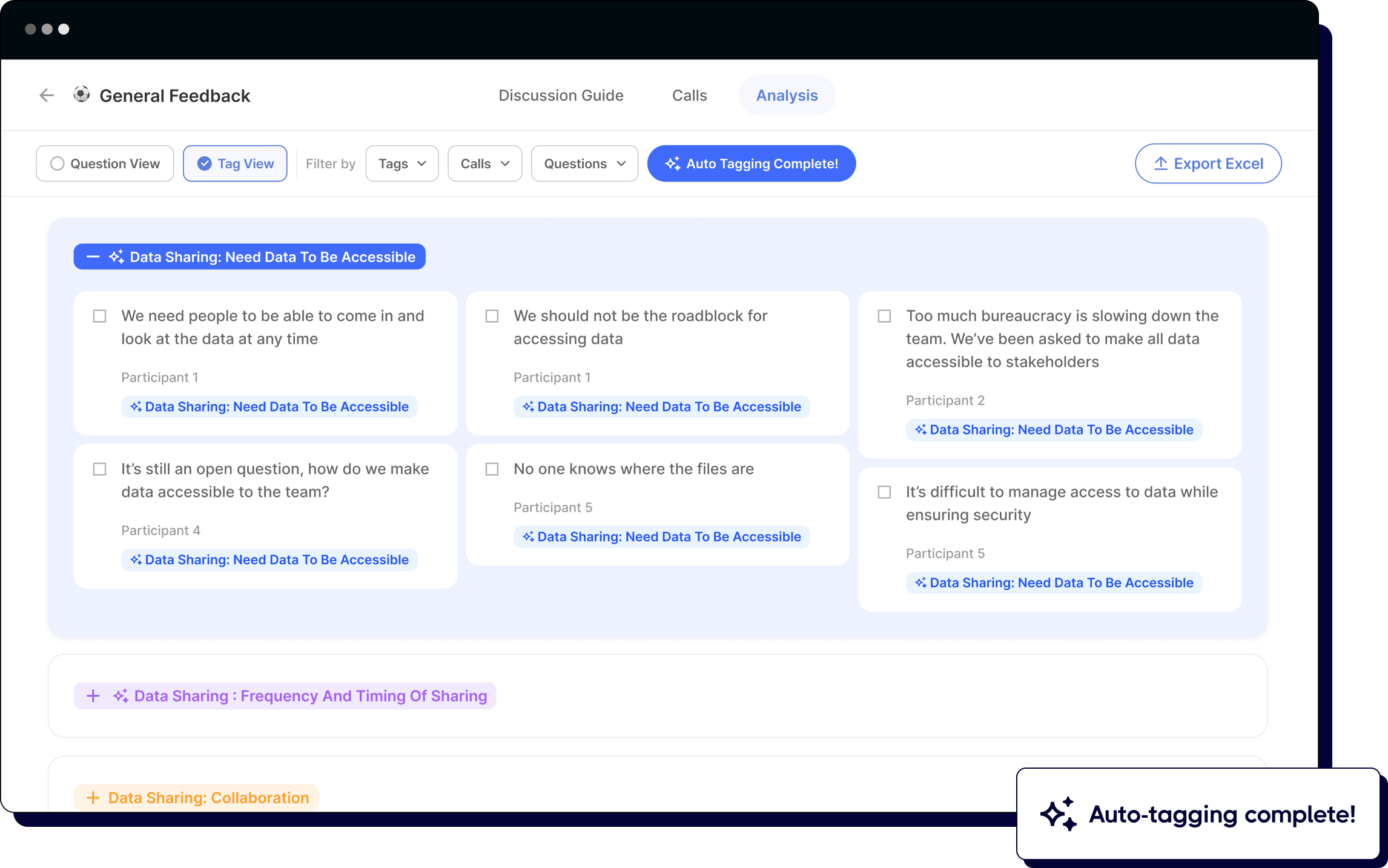
This process can be meticulous and requires careful attention to detail to ensure no themes are overlooked. Looppnael’s AI thematic tagging simplifies this task by automating the identifying and grouping of the themes from the data. This thematic analysis saves time and effort and helps to review and adjust themes with the nuances of the research.
Step 3: Indexing the data
You assign data points to the thematic framework you’ve developed in the previous step to ensure all the relevant information is linked to the specific themes or sub-themes. This meticulous process asks you to organize all the data systematically and identify patterns and connections across the dataset. Careful indexing ensures that no critical insights are missed.
Step 4: Charting
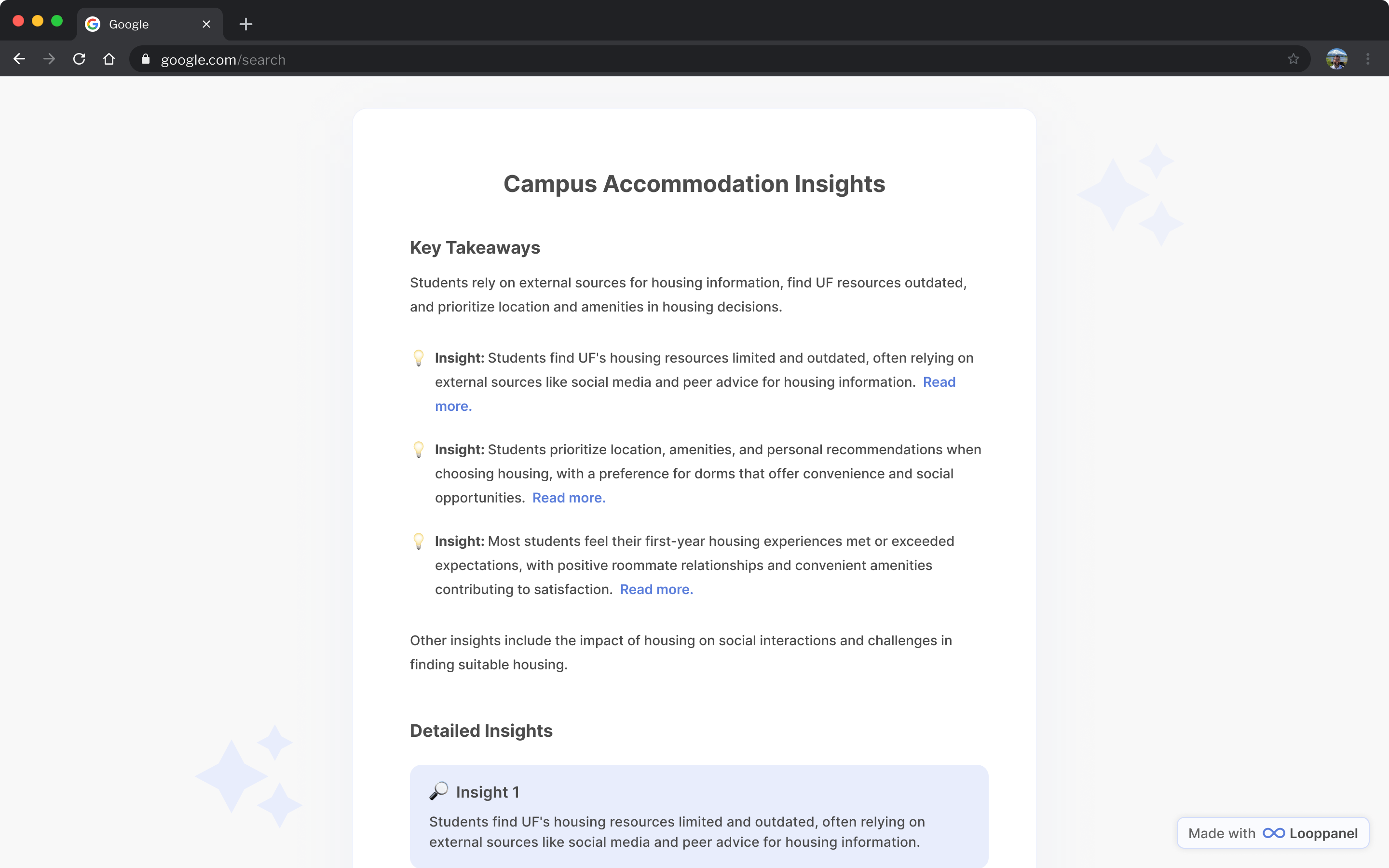
In this step, the data must be arranged in a structured format. This allows you to compare themes across participants, datasets, and contexts. Researchers can create detailed matrices where themes become columns and cases (like individual participants) become rows. This visual organization helps identify patterns while maintaining the rich context of individual experiences.
Step 5: Mapping and integration
This last step involves transforming organized data into actionable insights. It involves understanding the relationships between themes, explaining patterns, and drawing conclusions that align with your research objectives. AI-driven insights and editable summaries by Looppanel make this interpretation faster and more precise. It also allows you to add or edit summaries and share them directly with stakeholders.
What are examples of frameworks in qualitative research?
Framework analysis is the most used across multiple fields to address real-world challenges. Some of the examples of its application include:
1. Healthcare research
Framework analysis is used to understand patient experiences, such as barriers to accessing healthcare or evaluating the effectiveness of treatment plans. Researchers can analyze interviews with patients and healthcare providers to understand recurring themes like communication gaps.
2. Education
This method helps in analyzing feedback from students and teachers. Researchers can study qualitative data from surveys to understand factors affecting student engagement or the impact of teaching patterns.
3. Market research
Businesses use this analysis to understand customer behavior and the shift in it. Focus group discussion and customer feedback are analysed to understand the trends, preferences and pain points.
4. Public health campaigns
It is used to assess the effectiveness of a health awareness campaign by analyzing qualitative feedback from participants, identifying areas for improvement, and understanding future strategies.
5. Social policy development
Policymakers use this analysis to understand public opinions on social issues like housing, employment or climate change. This provides insights that guide decision-making and policy formulation.
What is the difference between thematic analysis and framework analysis?
Thematic and framework analysis differ in approach and purpose.
Framework analysis:
- Uses a matrix-based structure to systematically organize data
- Can incorporate both pre-defined and emergent themes
- Particularly suited for managing large datasets in team research
- Maintains clear links between original data and interpretations
- Emphasizes transparency and systematic approach
Thematic analysis:
- More flexible in its approach to organizing data
- Can be purely inductive or incorporate theoretical frameworks
- Particularly suited for exploring meanings and experiences
- Can be adapted to various epistemological approaches
- Emphasizes pattern recognition and theme development
Both methods:
- Can be used for applied or theoretical research
- Require rigorous engagement with data
- Can incorporate both predetermined and emergent themes
- Need systematic documentation of analytical decisions
Challenges and solutions of framework analysis
Like any other research method, framework analysis has its own challenges. Below are the challenges and tips to solve them:
1. Time-consuming process: Framework analysis requires systematic indexing and charting of vast amounts of qualitative data, which can be extremely time-consuming. Researchers often spend weeks working through interview transcripts, field notes, and observational data, particularly when dealing with large-scale studies involving multiple participants.
Solution: Qualitative data analysis tools can help streamline the organization and retrieval of data. Also, researchers should allocate realistic timeframes for each analysis stage. Breaking the analysis into manageable chunks, setting clear milestones, and using systematic documentation can help manage the time investment effectively.
2. Framework rigidity: Traditional approaches to framework analysis can sometimes feel restrictive, especially when unexpected themes or patterns emerge during the study. This can make it challenging to incorporate new insights that don't fit neatly into the predetermined framework.
Solution: Researchers should build regular review points into their analysis process to assess and refine their framework. This includes scheduling periodic framework evaluations, documenting modifications, and maintaining flexibility to add or adjust categories as new patterns emerge in the data.
3. Bias in interpretation: Researchers may unconsciously allow their own perspectives, expectations, or previous experiences to influence how they interpret the data. This can lead to overlooking important themes or overemphasizing certain aspects of the data.
Solution: Implement multiple validation strategies such as peer review, team-based coding, and systematic documentation of analytical decisions. Regular team discussions about emerging findings and alternative interpretations can help surface and address potential biases.
4. Data management complexity: Organizing and managing large amounts of qualitative data can become overwhelming, particularly in studies with multiple data sources or longitudinal designs.
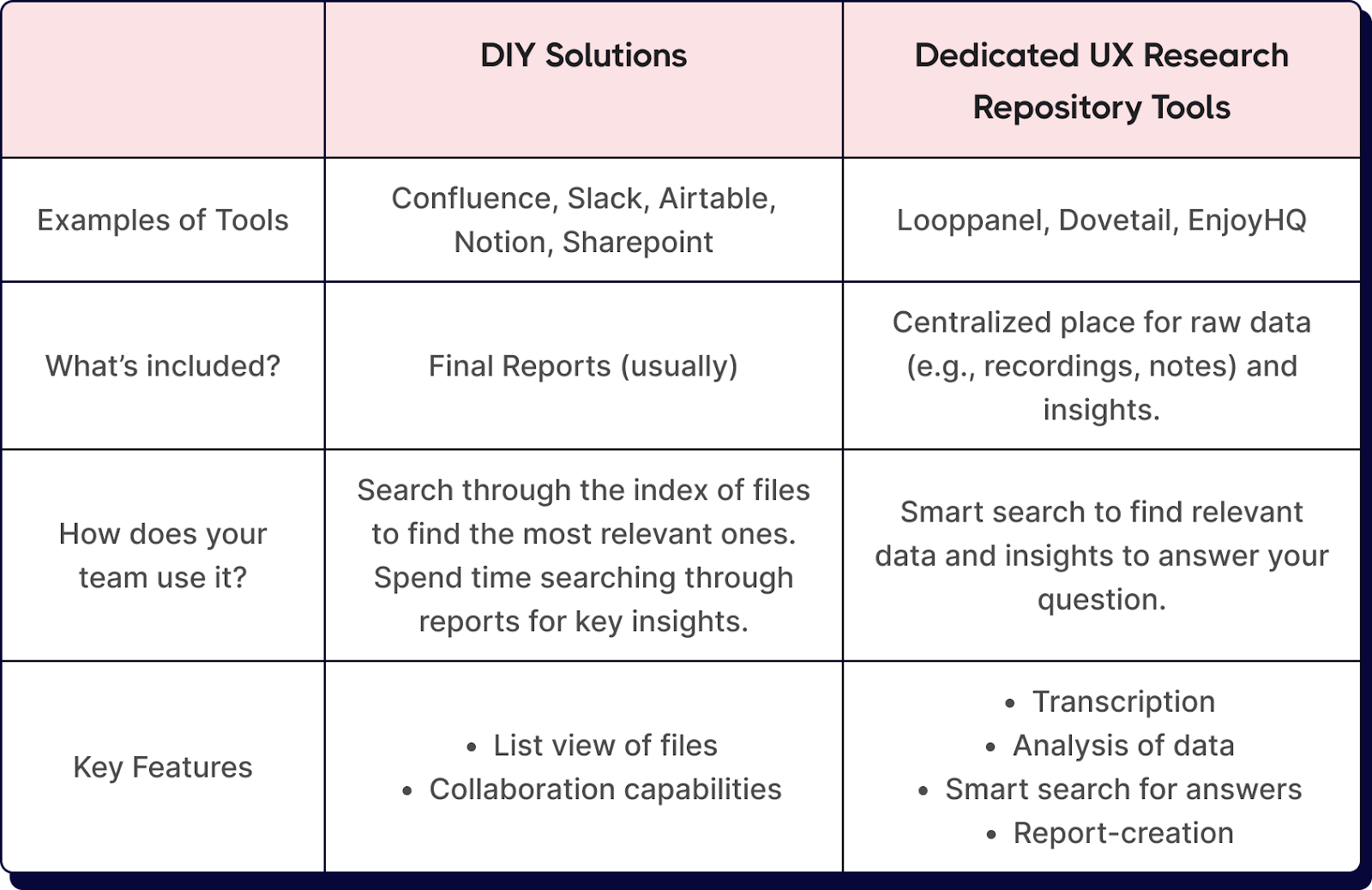
Solution: Establish clear data management protocols from the start, including consistent file naming conventions, systematic backup procedures, and clear documentation of analytical decisions. UX research repository tools can support this process while maintaining the integrity of the analysis.
5. Team coordination challenges: When multiple researchers are involved in the analysis, maintaining consistency in approach and interpretation can be difficult.
Solution: Regular team meetings to discuss coding decisions, emerging themes, and analytical challenges help ensure consistency. Create detailed analysis protocols and maintain clear communication channels for resolving questions or concerns.
Tips for conducting practical qualitative framework analysis
To help researchers navigate the above challenges successfully and ensure high-quality analysis, we've compiled a set of practical guidelines that can be implemented in any framework analysis project
1. Building your analytical framework
Develop your framework iteratively, starting with a test run on a small sample. Create crystal-clear definitions for each theme and include concrete examples. Keep your framework flexible enough to accommodate unexpected insights while maintaining its structural integrity.
2. Leverage technology
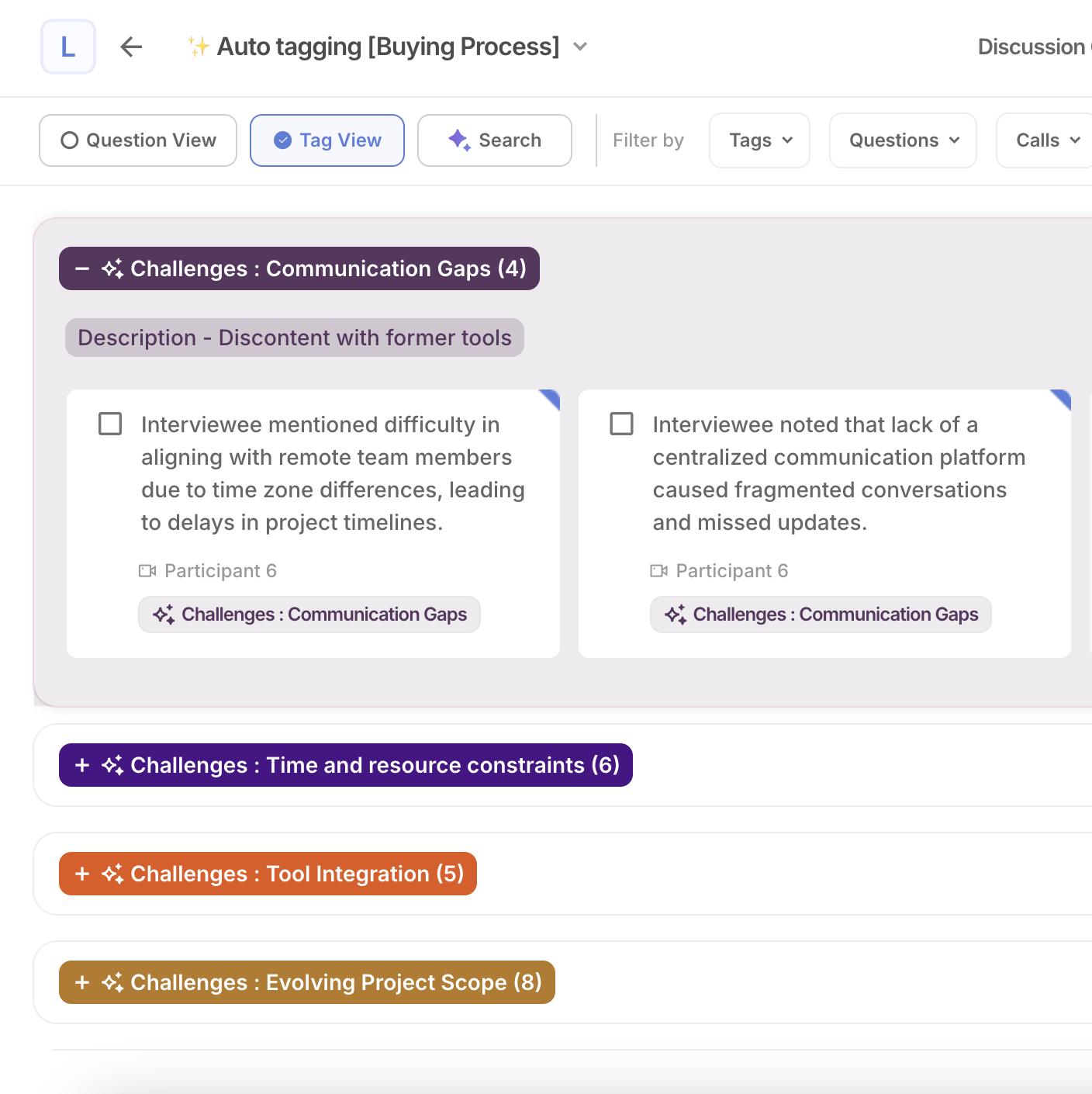
Qualitative data analysis software like Looppanel can help researchers with framework analysis. Looppanel is a research repository that keeps your data organized. It automatically records your user interviews, provides highly accurate transcripts and makes the process easier and manageable.
3. Manage the data effectively
Break down your analysis into digestible chunks to avoid feeling overwhelmed. Set realistic daily coding goals and use visual aids like color coding to help identify patterns. Create quick reference summary sheets for each data source to maintain easy access to key points.
4. Ensure quality and credibility
Regular quality checks are crucial for robust analysis. If working in a team, schedule consistent check-ins to discuss coding decisions. If working solo, consider seeking peer feedback at key stages. Actively look for contradicting evidence to challenge your assumptions.
5. Maintain the bigger picture
Don't get lost in the details. Schedule regular "zoom out" sessions to review your overall progress and emerging patterns. Create visual maps of connections between themes and keep returning to your research questions to maintain focus.
6. Seek feedback
Collaborate with researchers to review your framework and analysis. Gather feedback to remove biases from the process and strengthen the analysis base.
Conclusion
Framework analysis stands as a robust and systematic approach to qualitative data analysis. When properly executed, this methodology enables researchers to move from raw data to meaningful insights through a transparent and documented process.
Its versatility makes it particularly valuable across diverse fields, from healthcare research examining patient experiences to educational studies analyzing learning outcomes, and from policy research informing decision-making to market research understanding consumer behavior.
Modern qualitative data analysis tools like Looppanel, can significantly streamline parts of the process by assisting with data organization, transcription, and initial coding, allowing researchers to focus more deeply on interpretation and analysis. Book a demo to explore Looppanel’s capabilities.
Frequently asked questions (FAQ)
1. How do you make an analysis framework?
To create a framework analysis, you must identify the research questions or objectives guiding the study. Once clear, you must select a relevant framework that connects with your study’s goals.
2. What are the four main parts of qualitative analysis?
The four main parts of qualitative analysis include gathering data through a survey and coding the data to identify themes and patterns. The last two parts include data interpretation for researchers to understand the context and themes and presentation of data for sharing the reports with stakeholders.
3. What are the five methods of qualitative data analysis?
- Thematic analysis focuses on identifying and analyzing the themes within qualitative data.
- Grounded theory is a systematic approach involving constructing theories from data rather than testing pre-existing hypotheses.
- Framework analysis is a structured approach in which data is sorted into a pre-determined framework.
- Narrative analysis examines the stories or personal accounts to understand individual sense of experience.
- Content analysis is a systemic approach to analyzing the context of text data, such as interviews or written documents, to identify patterns and trends.