Affinity mapping has long been a trusted method for sorting through complex ideas and data. It helps teams identify patterns and set actionable goals. Yet, the process isn't without its challenges.
Often, the sheer volume of information and the subjectivity in grouping can overwhelm even the most organized teams. This is where the power of AI steps in. AI for affinity mapping streamlines sorting and categorizing data with precision and speed. Using AI, teams can overcome all the common hurdles and efficiently perform affinity mapping.
In this blog, we'll learn how to use AI for affinity mapping, its benefits and some practical tips to integrate this technology into your strategy for 2024.
What is the use of affinity diagram?
An affinity diagram is a tool that organizes large amounts of data into related groups after brainstorming sessions. It helps teams visualize the relationship between different ideas and issues. They can tackle complex problems by breaking them into more manageable parts through this process.
Let's understand how this tool functions and its practical applications:
- Clarity in complexity: Often used in project management and team meetings, affinity diagrams help clarify thoughts that appear chaotic. They arrange scattered ideas into coherent groups to make it easier to understand and act upon.
- Enhancing decision making: Teams can visually categorize ideas or problems, prioritize issues, and see where to focus their efforts. This will lead to more informed decision-making processes.
- Boosting collaboration: Affinity diagrams require team involvement and promote a collaborative environment where every member contributes. This collaboration ensures diverse perspectives are considered.
- Identifying patterns and relationships: As information is grouped, patterns that were not initially apparent may emerge. This can lead to discovering the root causes of problems and how different issues are interconnected.
- Streamlining project management: In project management, affinity diagrams can sort out project tasks, assign roles based on issue categories, and organize timelines more effectively.
- Customer feedback organization: For businesses, customer feedback is essential. An affinity diagram helps categorize this feedback into themes and makes it easier to see which areas need attention and improvement.
How is AI used in mapping?
AI in affinity mapping is changing how we approach mapping tasks. It helps organize and interpret complex data sets more efficiently. Here's a closer look at how AI enhances this process and why it's becoming a must-have tool for businesses and teams.
- Automates data organization: AI for affinity mapping automates the initial sorting of data, which is traditionally done manually. It uses algorithms to scan vast amounts of information and categorize them based on predefined criteria or learned patterns. This means what used to take hours can now be done in minutes.
- Enhanced pattern recognition: AI can identify patterns that might not be obvious to the human eye. AI for affinity mapping analyzes data to find correlations and associations between different ideas or issues. It presents these connections in an easy-to-understand format. This capability helps in strategic planning and problem-solving.
- Interactive and adaptive learning: As AI systems are exposed to more data, they learn and adapt, which improves their categorization algorithms over time. This aspect of machine learning means that the more you use AI for affinity mapping, the better it gets at understanding your specific needs and nuances.
- Practical applications: From marketing strategies to product development and customer feedback analysis, AI-powered affinity mapping is used across various fields to enhance decision-making and streamline processes.
The process of AI-driven affinity mapping
AI-driven affinity mapping process streamlines and augments the traditional method by utilizing machine learning algorithms to automate and refine the categorization process. Here's a detailed look at how this process unfolds:
Firstly, we start with the data collection phase. In an AI-driven system, data can be gathered from various sources like surveys, customer feedback forms, brainstorming sessions, and online data repositories. The diverse nature of data sources ensures that the AI has a broad base from which to learn and categorize.
Once data is collected, the AI for affinity mapping begins by preprocessing this data. This involves cleaning the data by removing duplicates, correcting errors, and standardizing formats to ensure consistency. The AI then employs natural language processing (NLP) techniques to analyze and understand the context and nuances of the text-based data.
The next step involves the actual mapping. AI algorithms classify the data into thematic clusters based on similarities in the content. This is achieved through techniques like semantic analysis, where the AI assesses the meaning behind the words and clustering algorithms that group similar items. The ability of AI to recognize patterns that are not immediately obvious to human analysts is one of the primary benefits of AI-driven affinity mapping.
Furthermore, the AI continually learns and adapts as it processes and sorts data. Machine learning models adjust their parameters based on new data and feedback, making the system more efficient and accurate over time.
Once the data is organized into clusters, these are then visually represented in an affinity diagram. The diagram typically displays themes as clusters of related ideas and makes it easier for teams to analyze the data and derive actionable insights. This visual representation helps identify relationships between different data clusters.
How to do affinity mapping?
To conduct a traditional affinity mapping session, start by collecting all relevant data points from brainstorming sessions, user feedback, or any other sources of information.
Spread these points on sticky notes or a digital platform where they can be easily moved and grouped. Begin to sort these notes into groups based on their natural relationships or emerging themes. It's a collaborative effort, usually requiring team members to discuss and negotiate the placement of ideas actively.
Once the groups are formed, they are labelled with a headline that captures the essence of the theme. This visual data arrangement helps teams see the bigger picture and make better decisions.
Learn more about How to Use an Affinity Diagram Template in our detailed guide.
How to create an affinity map on Figma?
Creating an affinity map on Figma, a popular digital design tool, involves several steps. Here's how you can set up an affinity map:
- Prepare your Figma workspace: Open a new file in Figma and set up a large canvas. Since affinity mapping can sometimes involve many notes, ensure your workspace is sufficiently expansive.
- Import data: You can manually add data points using text boxes or import data from other sources. Use plugins like 'Sticky Notes' to create notes directly in Figma, which mimic the physical sticky notes commonly used in affinity mapping.
- Create clusters: Start grouping related notes. You can change the colours of sticky notes to represent different themes or categories. Drag and align these notes close to each other to form clusters.
- Label clusters: Once a cluster is formed, create a heading for each group. Use a larger, bold text at the top of each cluster to define the group's theme or insight.
- Refine and rearrange: Some themes might need refinement or splitting into sub-themes as you work through the clustering process. Continuously iterate on the arrangement until the groups accurately reflect the underlying patterns and relationships.
- Collaborate and share: One of Figma's strengths is its ability to facilitate real-time collaboration. Invite team members to view or edit the map and provide feedback or add additional data points.
- Finalize and export: Once your affinity map is complete, you can finalize the design and export it as a PDF or image file for presentation or further analysis.
What are the best practices for creating affinity diagrams?
Whether you're using traditional methods or leveraging AI tools like ChatGPT for affinity mapping, here are some best practices to ensure your diagrams deliver the most value:
- Gather comprehensive data: The foundation of any good affinity diagram is the data it represents. Ensure you collect a broad and diverse set of data points to understand the topic's scope fully. This includes feedback, ideas, observations, and issues from various stakeholders.
- Use an AI tool: Incorporate AI for affinity mapping to handle large data sets efficiently. AI tools can quickly sort through vast amounts of information, identify patterns, and suggest groupings based on data similarities which might not be immediately apparent.
- Engage diverse perspectives: When manually sorting data into groups, involve team members from different departments or backgrounds. This diversity can lead to more comprehensive groupings and innovative solutions to complex problems.
- Iterate and refine: Affinity mapping is not a one-and-done process. It requires iterative refinement. As you start to see patterns emerge, reevaluate and adjust your groups to reflect the relationships between data points better.
- Utilize tools like ChatGPT: When using AI like ChatGPT for affinity mapping, tailor the AI's focus based on specific project needs. ChatGPT can assist in generating and categorizing ideas. It will ensure that the mapping is thorough and aligned with your objectives.
- Visual presentation: Once your data is grouped, ensure your affinity diagram is clear and visually engaging. Use colours or icons to differentiate between themes, and ensure each group is clearly labelled and easily understood.
Advantages of AI in affinity mapping
With the help of affinity mapping tools like ChatGPT, organizations are seeing significant improvements in efficiency and accuracy. Here are some of the standout advantages:
- Enhanced speed and efficiency: AI accelerates the data sorting process, a traditionally time-consuming task. It automates the initial stages of grouping data and allows teams to focus on analysis and decision-making rather than getting bogged down by the mechanics of data organization. This swift processing translates into faster project turnaround times and increased productivity.
- Improved accuracy and pattern recognition: AI systems, including ChatGPT, are equipped with advanced algorithms capable of identifying patterns that might elude human analysts. This precision in detecting nuances and correlations within large data sets ensures that the insights derived are based on comprehensive and accurate information.
- Scalability: Using AI for affinity mapping facilitates handling larger datasets that would be overwhelming for manual processes. This scalability makes AI-driven affinity mapping particularly valuable for growing companies that need to process increasing amounts of data without compromising the quality of insights.
- Collaborative and dynamic: Tools like ChatGPT for affinity mapping are designed for collaboration. They allow team members to interact with the AI and provide feedback from which the system can learn to refine its outputs. This dynamic interaction improves the mapping results over time and encourages a collaborative environment where insights are continuously enhanced.
Implementing AI for affinity mapping in your organization
You need to pick a good AI tool to implement AI for affinity mapping in your organization. Here are three best picks:
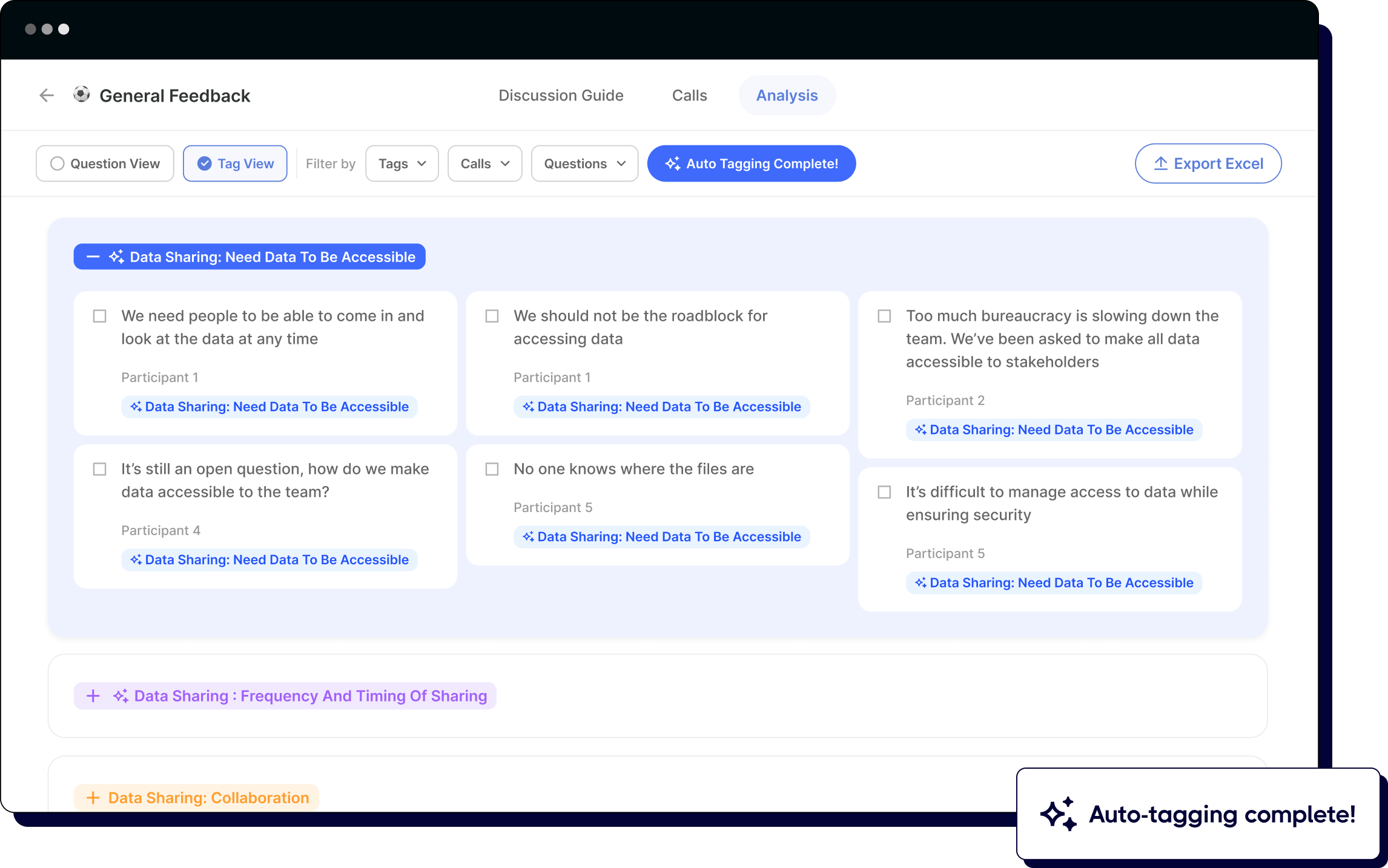
Looppanel sets itself apart in AI-driven affinity mapping by offering precision tools explicitly tailored for in-depth user research and analysis. Unlike basic AI tools that merely sort data, Looppanel's AI is designed to understand the context behind the data. This enables it to provide more nuanced insights and recommendations that are tailor-made for the specific contours of user research.
Key unique features of Looppanel
- Real-time transcription and tagging: Captures live discussions and automatically tags content.
- Interactive analysis board: Users can interact with their data through a dynamic dashboard and navigate through large data sets.
- AI-enhanced summary reports: Generates concise reports from complex data sets using advanced AI.
- Collaborative workspace: Enabling users to collaborate on data analysis in real time.
Pricing
Looppanel offers pricing plans catering to different user needs, from $27 per month for individual researchers to custom pricing for large enterprises requiring advanced features and support.
Looppanel G2 review
Looppanel has a strong reputation among its users, as evidenced by a high rating of 4.6 out of 5 on G2.

Wondering how to use ChatGPT for affinity mapping? Let us help you!
Using ChatGPT for affinity mapping brings a unique twist to organizing complex data. ChatGPT leverages its advanced natural language processing capabilities to quickly sift through vast amounts of text, categorize themes, and identify relationships. This allows teams to focus on deeper analysis rather than initial data organization.
To use ChatGPT for affinity mapping, simply input your raw data, and it will propose thematic clusters to help streamline the process and enhance collaborative brainstorming sessions.
Here are two sample prompts if you need some inspiration getting started with ChatGPT:
Prompt for initial data sorting: "ChatGPT, I have compiled a list of customer feedback comments from our latest product release. Could you help me sort these into thematic clusters based on common issues and suggestions?"
Prompt for identifying relationships: "ChatGPT, based on the attached brainstorming notes from our last team meeting, can you identify and organize the key ideas into groups that reflect their relationships and potential impact on our project development?"
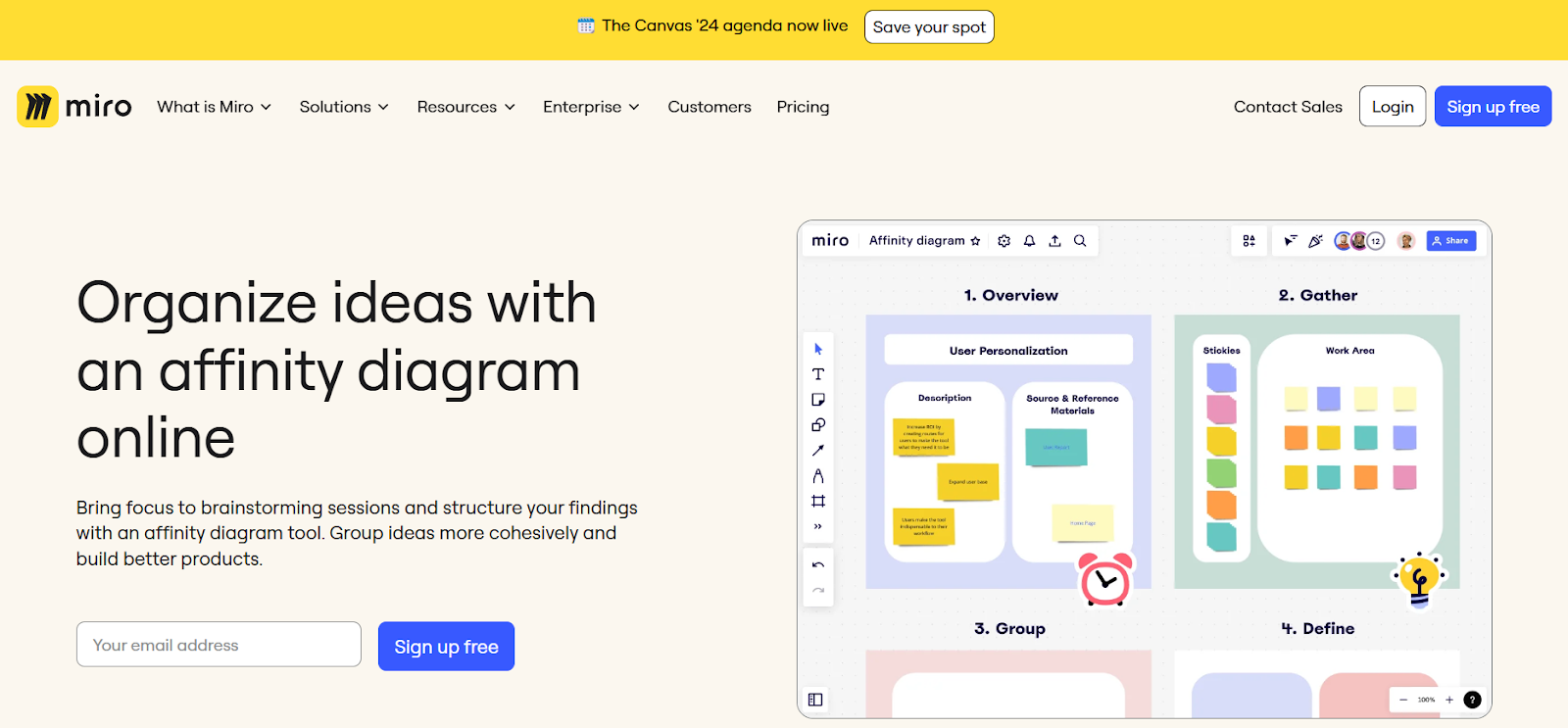
Miro is a great tool for affinity mapping that transforms how teams brainstorm, organize, and refine their creative processes online. The platform offers ready-made templates for various brainstorming techniques, including affinity diagrams. With this, your ideas can be grouped, prioritized, and transformed into structured plans.
Key features
- Intuitive drag-and-drop interface: Utilize a user-friendly interface with drag-and-drop items across the canvas.
- Integrated voting system: Engage team members in prioritizing ideas with an embedded voting system.
- Automatic linking and relationship lines: Automatically generate visual links between related ideas or groups.
- Search and tagging: Leverage search functionality and tagging to manage and navigate through complex datasets.
Miro pricing
Miro's pricing includes a free plan with basic features and three boards. The Starter plan costs $8 per member/month, the Business plan is $16, and the Enterprise plan offers custom pricing for advanced needs.
Miro G2 review
Miro boasts a strong G2 rating of 4.8 out of 5 stars. Users commend its user-friendly interface, versatile collaboration tools, and effective support for various business applications.
Redditors' opinion on AI tools for affinity mapping
The opinions on AI tools for affinity mapping range from cautious to optimistic. One view emphasizes the importance of team immersion and collective understanding, arguing that traditional methods are more effective for in-depth analysis and that AI might detract from team alignment. In contrast, another perspective sees AI as a valuable tool for pre-sorting data and streamlining initial stages, allowing researchers to focus on deeper analysis during team sessions.
A third, more balanced view acknowledges the efficiency gains from AI features in research analysis while also highlighting potential limitations. This perspective emphasizes the importance of tool quality and specificity, suggesting that while AI can augment the affinity mapping process, it should enhance rather than hinder the research workflow. Overall, the debate centers on balancing the benefits of AI-driven efficiency with the need for human insight and team collaboration in qualitative research.
FAQs
- How to make an affinity map?
To create an affinity map:
- Gather all your data points and write them on sticky notes or a digital tool.
- Cluster-related items together on a board or screen.
- Label each cluster with a summary that captures the essence of its content.
- Where can I create an affinity diagram?
You can create an affinity diagram on platforms like Miro, Figma, or Lucidchart, which offer collaborative digital workspaces. These tools provide templates and functionalities to easily group and label data.
- Does Affinity have AI?
Currently, Affinity software, known for graphic design and photo editing, does not include AI-driven tools specifically for creating affinity diagrams or automated data analysis. Affinity focuses more on creative processes rather than data sorting or AI functionalities.
- How to use AI for image recognition?
To use AI for image recognition, train a model with a labelled image dataset. This allows the AI to learn and identify patterns and features. Tools like TensorFlow, PyTorch, or pre-built APIs like Google Vision can be used to implement these models. They enable the AI to recognize and categorize new images based on the training it received.
- What is the difference between a mind map and an affinity diagram?
Mind mapping and affinity mapping serve different purposes. A mind map is a visual representation of thoughts and ideas branching from a central concept, used primarily for brainstorming and organizing thoughts. In contrast, an affinity diagram (or affinity map) groups large amounts of data into related themes or categories, often after data collection, to identify patterns and relationships.
- What is the difference between empathy mapping and affinity mapping?
Empathy mapping and affinity mapping are tools used to organize qualitative data but focus on different aspects. Empathy maps are designed for diving deep into an individual's experience, capturing what they say, think, do, and feel, which is crucial for user-centred design. Affinity mapping, however, sorts broader data into themes to analyze patterns across many responses, which helps identify common problems or ideas.
- What is the difference between card sorting and affinity mapping?
Card sorting and affinity mapping are both organizational techniques but are used differently. Card sorting is where users categorize information into groups based on their understanding, often used to inform website navigation and information architecture. Affinity mapping, on the other hand, is typically used by teams to organize ideas or data into thematic groups after brainstorming sessions.