Unlike quantitative data, which can be analyzed easily by the use of statistics, qualitative data needs a little more effort in analysis and sifting through human emotions to understand the underlying patterns. Therefore, when people mention qualitative analysis, the very first thing that comes to everyone’s mind is thematic analysis!
It dives deeper into the data collected through surveys, interviews, or focus groups, and understands the core meaning behind what participants actually mean. This approach helps researchers make accurate and effective decisions that drive real change. This guide covers the different types of thematic analysis and how to choose the approach that best fits your needs.
TL;DR
- A method for finding patterns in qualitative data from interviews, focus groups, and surveys.
- Main approaches: Inductive (themes emerge naturally) vs. Deductive (guided by existing theories); Semantic (surface meanings) vs. Latent (underlying meanings).
- Choice depends on goals: exploratory research = inductive; hypothesis testing = deductive; customer feedback = semantic; complex experiences = latent.
- Best for large volumes of qualitative data where you need to organize information into meaningful patterns.
- Modern AI tools can automate much of the analysis process, reducing time from days to minutes
What is thematic analysis?
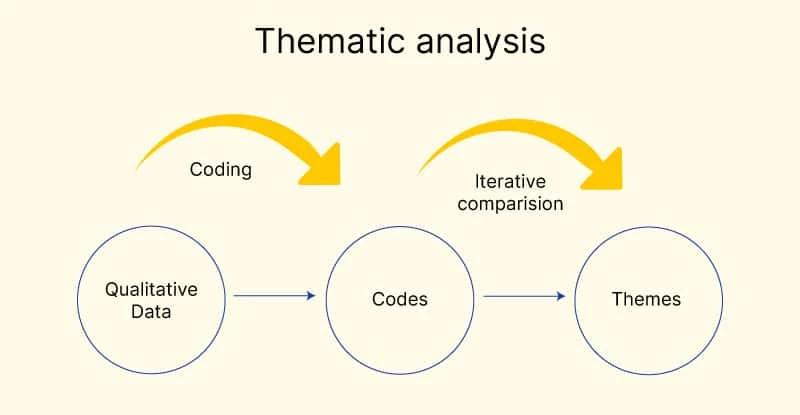
Thematic analysis is a widely used method for qualitative data analysis. It involves identifying, analyzing, and reporting themes or patterns found within a data set. This approach is often used with data from interviews, focus groups, or user research surveys to better understand people's thoughts, experiences, and behaviors. Thematic analysis allows researchers to identify and interpret recurring patterns to turn raw information into meaningful insights.
What are the types of thematic analysis?
Different types of thematic analysis are designed to make qualitative research more systematic and insightful. These are as follows:
1. Inductive thematic analysis
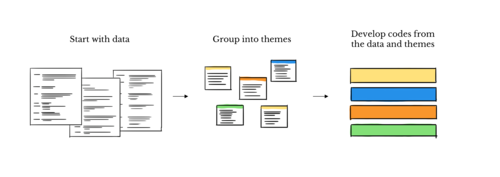
In this qualitative UX research method, analytical themes emerge organically from the data. You don't pre-set or base them around a certain premise, rather, you synthesize themes from the data themselves.
Example: A study on client satisfaction may find an emergence of themes like "ease of use" or "price satisfaction" with no predefined categories.
2. Deductive thematic Analysis

Unlike inductive thematic analysis, deductive analysis starts with a theory or framework. The researcher applies the existing concepts to identify the theme and test the hypothesis instead of allowing themes just to come out.
Example: A UX researcher may apply specific usability principles to check whether user feedback matches these predefined expectations.
3. Semantic thematic analysis
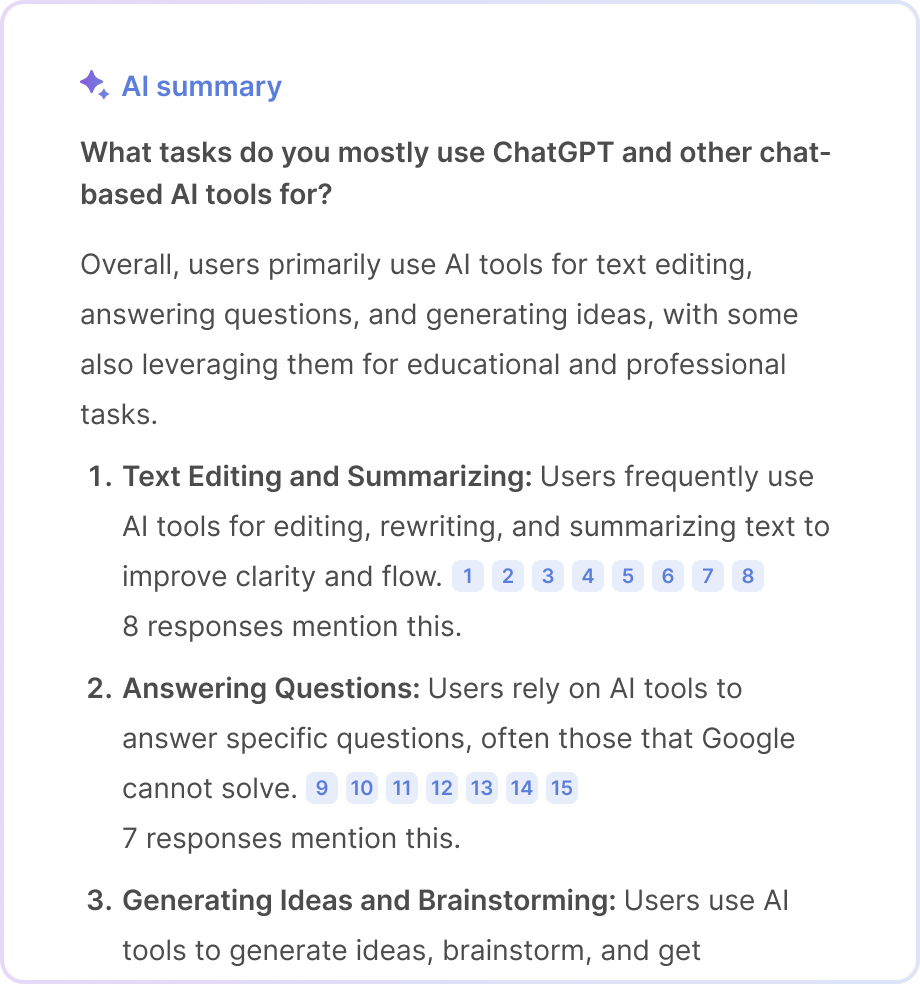
Semantic thematic analysis identifies themes based on the explicit and surface-level meanings in the data. Rather than interpreting hidden or implied meanings, this approach focuses on what is directly stated. It’s particularly effective for organizing straightforward feedback into actionable categories, making it easier to summarize and present findings.
Example: Customer reviews could be categorized into such themes as "fast shipping" or "quality issues."
4. Latent thematic analysis
Latent thematic analysis goes below the surface to find the greater degree of meanings or assumptions contained in the participants' responses. It’s more focused on what is implied rather than what is explicitly stated.
Example: The internal feedback provided by employees may indicate the latent themes around "feeling undervalued" while surface-level comments are presented about workplace perks.
Thematic analysis: choosing the right approach
The right process of thematic analysis depends on a combination of your research goals, the nature of your data, and the insights you hope to find. Each has its strengths, so it comes down to what you want and in what context. Let's break it down:
1. Research goals
Your primary objective determines whether to take an inductive or deductive path. Exploratory research benefits from an inductive approach, where patterns emerge organically from the data without preconceived frameworks. This works especially well when investigating new phenomena or seeking unexpected insights. Studies testing specific hypotheses or building on existing theories fare better with a deductive approach, using predetermined themes to guide analysis and validate theoretical frameworks.
2. Depth of analysis
The choice between semantic and latent analysis depends on your required analytical depth. Semantic analysis focuses on surface-level meanings and works well for straightforward feedback like customer reviews or survey responses. It helps identify clear patterns and explicit themes.
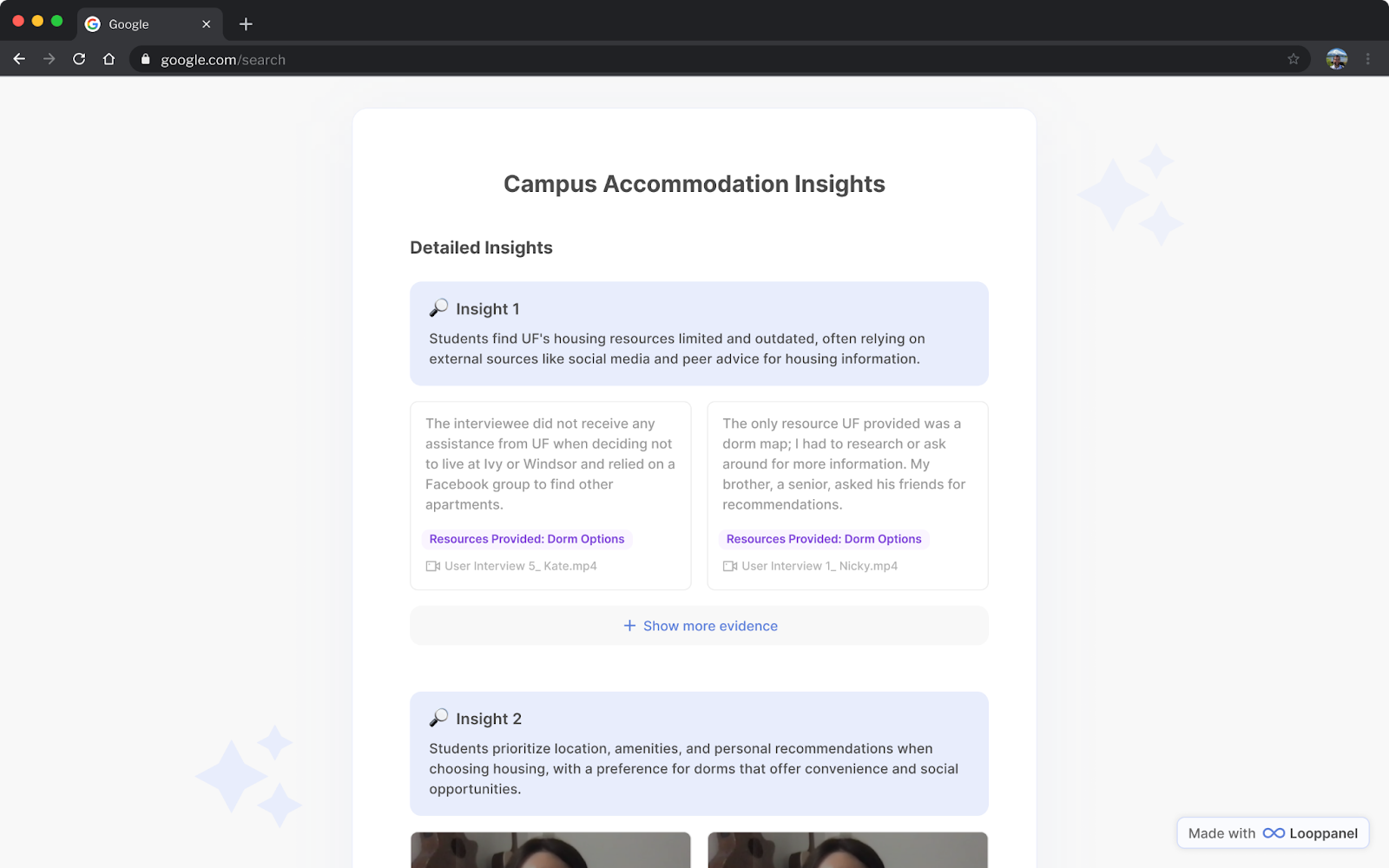
Latent analysis digs deeper, uncovering underlying assumptions and implicit meanings. This approach reveals subtle patterns and hidden biases, making it invaluable for research exploring complex social phenomena or participant experiences.
3. Practical factors
Resource availability and data characteristics significantly influence your analytical choices. Methods like reliability coding require multiple coders and substantial time investment but enhance analytical rigor.
The nature of your data also guides the approach – customer feedback often suits semantic analysis for quick categorization, while rich interview transcripts benefit from latent analysis to uncover deeper experiential insights. Balance methodological ideals against project constraints to develop an approach that delivers both rigor and feasibility.
Check out the top 5 tools for AI thematic analysis in 2024 to leverage AI for thematic analysis and gain deeper insights.
When to use thematic analysis?
Thematic analysis is particularly beneficial when working with large volumes of qualitative data. It helps organize and categorize information into manageable themes, making it easier to analyze and interpret.
Here are key scenarios where thematic analysis is ideal:
- If you’re starting, thematic analysis offers a straightforward, accessible way to analyze qualitative data without requiring advanced methodologies.
- You can also leverage AI for thematic analysis, whether working with transcripts, surveys, or focus group discussions, as it is ideal for finding recurring patterns and insights effectively.
- You can use the flexibility in thematic analysis to collaborate with participants in validating themes or just ensuring that their perspectives are well captured.
.png)
Conclusion
Thematic analysis converts raw qualitative data into something tangible and useful for the researcher to quickly identify patterns and trends. This approach helps to simplify the qualitative data analysis process, making it easier to interpret complex data.
Looppanel significantly enhances thematic analysis with its AI-powered features by automating time-consuming tasks. It saves you hours or even days of processing work using AI thematic tagging.
In just minutes, Looppanel’s smart AI can tag 80% of data that would typically take a day to process, giving you a head start in your research. All tags are traceable to the original source for transparency, and the human-in-the-loop approach makes editing a breeze when needed.
Book a demo today to see how Looppanel’s advanced features can transform your research workflow.
Frequently asked questions (FAQs)
1. What are the 6 types of thematic analysis in qualitative research?
The six types of thematic analysis include inductive, deductive, semantic, latent, codebook, and reflexive, each serving different purposes in analyzing qualitative data.
2. What are the three thematic analysis types?
The three common types of thematic analysis are inductive, deductive, and semantic, focusing on emerging themes, pre-existing frameworks, and surface-level meanings respectively.
3. What are inductive and deductive thematic analysis?
Inductive thematic analysis allows themes to emerge naturally from data, while deductive thematic analysis is guided by pre-existing theories or frameworks to identify specific themes in the data.