Only 3% of organizations have matured UX research to drive efficient business decisions.
This data is shocking, right?
UX researchers might have fine-tuned the data collection method, but the analysis remains questionable. How to approach it, which research analysis method will yield the most effective result, or how to ensure the traditional ways of research analysis are efficient, clear and actionable, remains a major issue for most of them.
It’s a common challenge everyone faces. The solution? This guide!
We'll walk you through how AI is transforming UX research analysis methods and overtaking traditional methods with speed and precision. Whether it is the analysis of qualitative interviews or survey data, this approach will empower you to confidently tackle any research scenario and drive maximum results out of your research.
What is user research analysis?
"Regardless of whether you’re dealing with a numeric dataset or a verbal interview, you’re always on the lookout for patterns and themes that can tell you something meaningful about the user, the product, or both,"
- Career Foundry
That’s exactly what user research analysis is. It is the process of organizing and interpreting raw data to find insights that will guide efficient decisions. It's transforming scattered information into a clear narrative of your users, their needs, and their experiences by identifying recurring themes and meaningful trends.
What are the traditional methods of UX research analysis?
Traditional UX research methods rely on manual processes for the interpretation of both quantitative and qualitative data. Quantitative research, involving statistical analysis, A/B testing, and surveys, relies on the researcher's observation of numbers, percentages, and trends to define patterns of user behavior.
Similarly, in qualitative UX research, techniques like thematic coding of interviews, focus groups, and usability testing rely on manual identification of recurring themes, sentiments, and pain points. While these methods have been effective for small-scale studies, they usually require a lot of time and effort, therefore making it hard to analyze big datasets or find deeper, more complex insights.
Master the art of UX research analysis by turning user feedback into actionable insights with these 10 simple steps!
To analyze user experience effectively, you can choose from quantitative, qualitative, or mixed user research analysis methods. Here's a concise overview:
Quantitative Methods
- Surveys: Collect large-scale user feedback to measure sentiment and trends.
- Analytics: Use tools like Google Analytics to track user behavior (e.g., flows, clicks).
- A/B Testing: Test variations to optimize conversions and engagement.
- Heatmaps: Visualize areas of high or low user interaction.
- Clickstream Analysis: Analyze user navigation paths and drop-off points.
Qualitative Methods
- User Interviews: Dive deep into user needs and motivations via one-on-one conversations.
- Focus Groups: Gather diverse insights through group discussions.
- Usability Testing: Observe users interacting with a product to identify pain points.
- Ethnographic Research: Study user behavior in their natural environment.
- Diary Studies: Capture real-time experiences over time.
- Card Sorting: Discover intuitive navigation structures based on user input.
- User Journey Mapping: Visualize touchpoints and pain points in user experiences.
Mixed Methods
- Sequential Design: Start with qualitative insights, then validate with quantitative data.
- Convergent Design: Collect and integrate qualitative and quantitative data simultaneously.
- Embedded Design: Combine both data types within a single study (e.g., usability tests).
- Triangulation: Use multiple methods to cross-validate findings.
- Complementary Design: Pair qualitative depth with quantitative breadth for holistic insights.
The evolution of UX research methods with AI
Even though traditional UX research analysis methods were foundational and everyone’s go-to choice, they usually fell short of dealing with the demands of modern data.
Manual sifting through interview transcripts or survey results was time-consuming and subjective. Hence, these methods were not efficient in finding patterns with large datasets. These limitations meant delayed insights and lost opportunities to act on user feedback.
This is where AI comes in—revolutionizing UX research by addressing these gaps in traditional methods. AI-driven tools are not just faster, they're smarter, offering capabilities that were unimaginable a few years ago.
5 benefits of AI UX research analysis methods
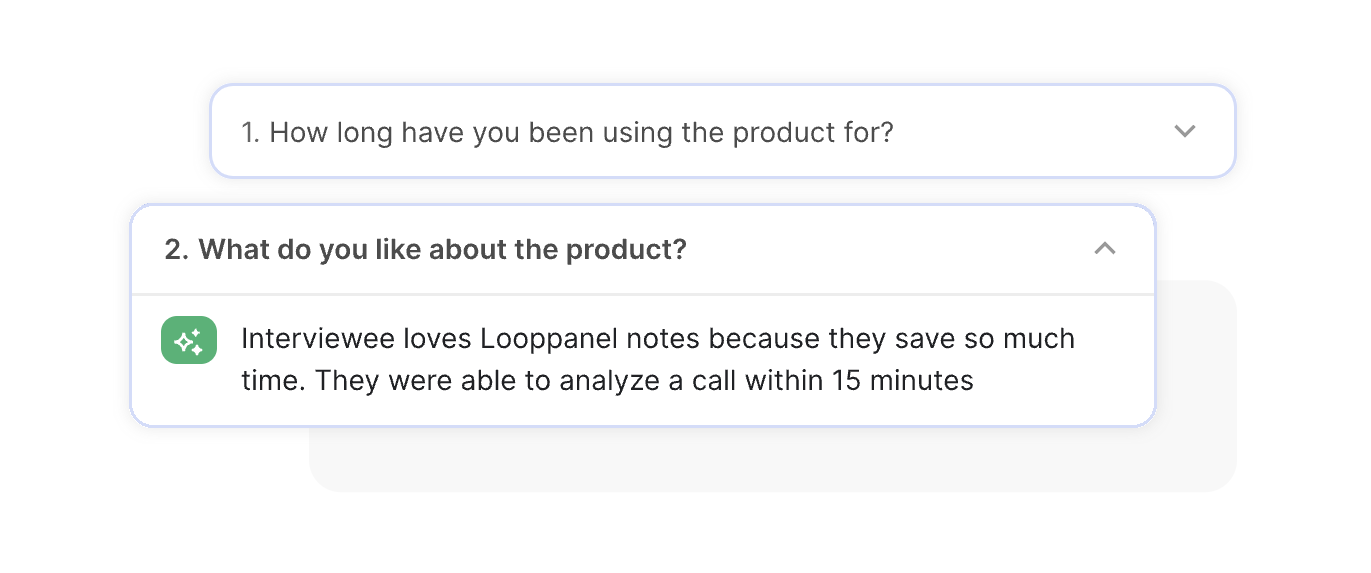
Speed and efficiency: AI with precision and speed, performs time-consuming tasks such as transcription and theme detection in an efficient manner, delivering results in hours rather than weeks.
- Improved accuracy: Algorithms remove human bias and errors, ensuring more accurate insights from raw data.
- Deeper insights: AI tools can help analyze large datasets in order to find hidden patterns, sentiment trends, and predictive behaviors that may be overlooked by traditional methods.
- Scalability: Whether it's 10 interviews or 1,000 survey responses, AI handles data at scale effortlessly, allowing researchers to focus on strategy.
- Actionable outputs: AI organizes findings into clear, stakeholder-ready formats, bridging the gap between analysis and decision-making.
Streamlining UX research with AI-powered tool - Looppanel
Analysis of research data can be a tricky and time-consuming activity, no matter the nature of the data. But with the right AI-powered tool, you can facilitate this step with much ease and precision.
You can use AI tools like Looppanel to help you transcribe UX research data and help with automatic note-tagging. Looppanel takes the burden off your shoulders and analyzes data with utmost precision in the market to ensure no important insight is overlooked and you get the maximum results out of your data. The key features include:
- Over 90%+ accurate transcripts: It provides highly accurate transcripts in 17 languages, which ensures a solid base for all further AI features like smart search, tagging, and note-taking.
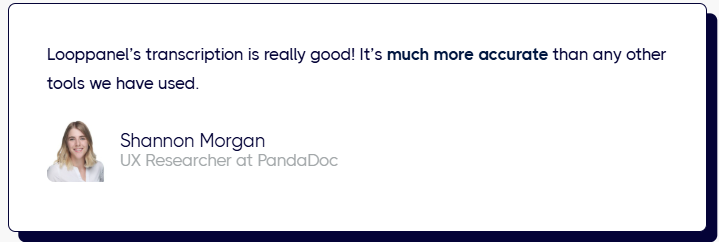
- Automatic note-taking: Looppanel automatically generates clear, organized notes from the data uploaded, reducing time spent on reviewing recordings and transcripts by 80%.
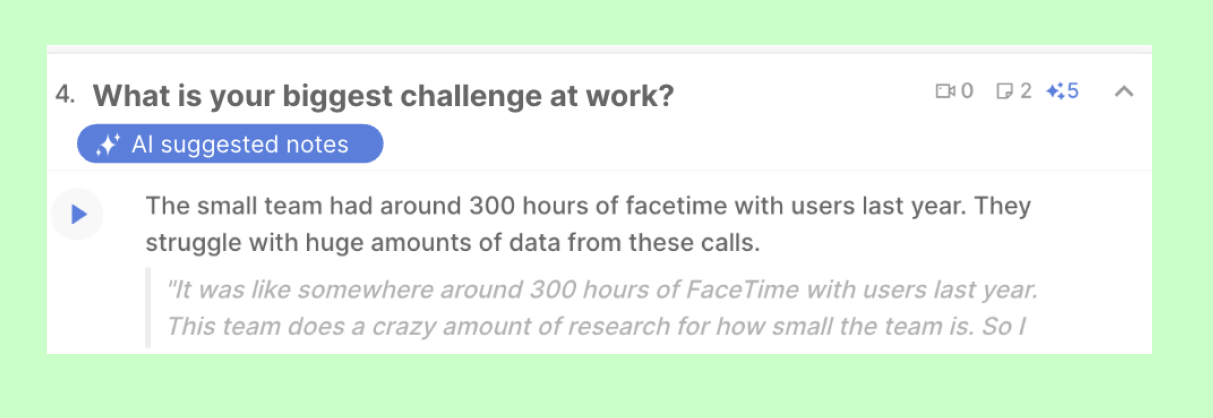
- AI thematic tagging: Automatically tag data into relevant themes, saving hours of manual effort in categorizing research findings and providing a high-level overview of emerging patterns.
- AI executive summary: Creates a concise executive summary from tagged data, including key insights and action points that can be shared with stakeholders immediately to improve reporting efficiency.
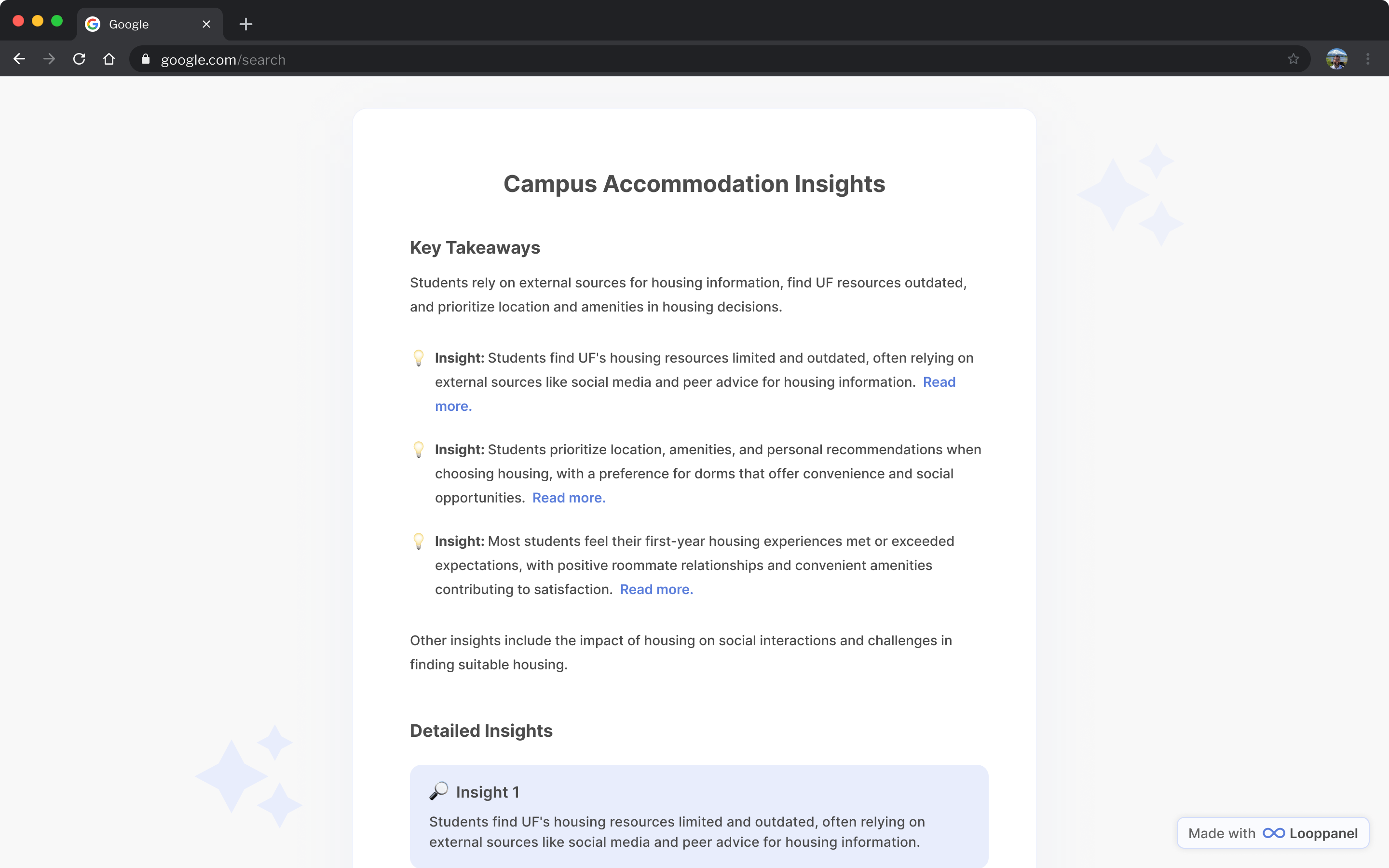
- AI smart search: Smart search goes beyond simple keyword matching in delivering answers with insights, citations, and raw data. It allows researchers to find what they need in seconds.
.png)
Related read: 11 analysis tools for user research and qualitative data
Best practices for integrating AI into your research workflow
AI can significantly improve the efficiency and accuracy of your UX research analysis, but it requires careful planning to implement.
You can follow these best practices to ensure that AI seamlessly complements your UX research workflow to drive better outcomes:
- Define clear objectives: Before incorporating AI, set clear research goals to ensure AI tools align with your specific needs, whether it's data analysis, pattern recognition, or enhancing user insights.
- Combine AI with human expertise: While AI is powerful, combining these results with a UX researcher’s expertise, results in better-informed, more nuanced conclusions.
- Update data sets regularly: Keep your data fresh and diverse. AI thrives on rich, up-to-date data, so make sure you're feeding it current user behaviors and feedback to keep it accurate.
- Ensure data privacy compliance: Always ensure that your use of AI tools falls within privacy regulations and protects user’s data to ensure trust and to keep you legally compliant.
- Iterate and experiment: While AI tools can bring new information and patterns to light, it is also equally necessary to iterate on the research process itself. Try different AI applications and see what works best for your team.
Conclusion
AI is reshaping the landscape of UX research, making it more efficient and impactful. Automation of tasks like transcription, note-taking, and theme identification saves time and ensures that the insights are more accurate and actionable, hence helping to deliver valuable results faster.
Looppanel takes it one step further with features like 90%+ accurate transcripts, sentiment analysis, and auto-tagging of themes, making the lives of UX researchers easier. Its intuitive tools save time like organizing your notes by interview questions and creating affinity maps, to make sure your insights are actionable. Try Looppanel today with a 15 day free tial or request a demo to see how Looppanel can elevate UX research!
Frequently asked questions (FAQs)
1. What are some challenges in traditional UX research analysis?
Traditional methods often involve time-consuming data organization and subjective interpretations, making it harder to derive actionable insights quickly and efficiently.
2. How can UX research analysis improve product decisions?
By uncovering user behavior patterns and needs, UX research analysis helps teams create user-centric designs and make informed product decisions.
3. What role does AI play in modern UX research?
AI enhances research by automating tasks like transcription and theme tagging, saving time and ensuring more precise data analysis.