If you've ever found yourself at your desk at 9 PM, drowning in interview transcripts with a cold cup of coffee, you know the pain of traditional qualitative analysis. Between re-reading transcripts, taking notes, re-playing videos, a single hour-long interview can consume 1.5-2 hours for analysis – while your stakeholders inevitably ask for "quick findings" from the study.
The landscape of interview analysis is changing dramatically in the last few years. AI tools are transforming how we extract meaning from qualitative research, cutting analysis time by up to 80%. Of course, you need to know how to use AI correctly to ensure that the quality of insights doesn’t suffer.
Whether you're a user researcher conducting discovery interviews, a market researcher tracking emerging trends, or a CX professional analyzing customer feedback, this guide will show you how to leverage AI to automatically identify patterns, generate compelling visualizations, and scale your research practice without sacrificing rigor.
What is AI interview analysis?
AI interview analysis uses advanced algorithms to automatically review and interpret interview data, such as transcripts or audio recordings, allowing researchers to extract insights more quickly and accurately. This process enables teams to identify key patterns and themes without delays in manual analysis.

AI-driven tools enhance qualitative data analysis by reducing human biases, ensuring more consistent results. By automating tasks like thematic analysis and transcript review, AI significantly streamlines the research process. As AI continues to transform user experience (UX) research, it offers a more efficient approach to analyzing complex data, making it a valuable tool in fields like user interview synthesis, AI thematic analysis, and AI text analysis.
How to do AI interview analysis: step-by-step guide
Interview analysis has traditionally been a time-consuming and labor-intensive process, often prone to human bias. With AI tools, the process becomes significantly faster and more accurate.
Here’s a step-by-step guide:
1. Prepare your interview data
Before you can begin any type of interview analysis, the first step is to organize and prepare your data. This typically means gathering and formatting your interview transcripts, as well as audio or video files.
- Text Data: If you’re working with text-based data, ensure that the transcript is clean and formatted correctly. Remove any irrelevant content like filler words and correct errors in the transcript. The cleaner your data, the more accurate your analysis will be.
- Audio/Video Files: If you have audio or video interviews, you’ll need to transcribe these into text. AI transcription services can help here by converting speech into text automatically. Some AI interview analysis tools like Looppanel include transcription features—you can go from recording to AI analysis in one place.
2. Choose the right AI interview analysis tool
Choosing the right AI-powered tool is critical for efficient and meaningful analysis. The correct tool can save you hours of manual labor while improving the accuracy of your insights.
- Text vs. audio/video need some text
- For text: Look for tools with strong pattern recognition and theme extraction.
- For audio/video: Choose platforms with accurate transcription and speaker detection.
In either case, test platforms you’re considering with data that you’re very familiar with to make sure that you can gut-check results first. Tools like Looppanel handle both formats seamlessly, automatically transcribing and analyzing your recordings.
- Key features to consider
When evaluating AI interview analysis tools, focus on key features that align with your research needs:
- Automated theme detection
- Sentiment analysis to understand emotional responses
- Real-time collaboration for team analysis
- Custom tagging capabilities
- Export options for sharing insights
Explore the Top 5 Tools for AI Thematic Analysis in this article.
- Cost and integration factors
The cost of AI interview analysis tools varies based on the features offered. Ensure that the tool you choose fits within your budget but also delivers robust features. Beyond the obvious pricing structures, also account for hidden costs that can significantly impact your budget. These include storage fees for maintaining historical data, charges for exporting analyses, costs for additional user seats, API access fees, and potential training and onboarding expenses.
Also, ensure that your selected tool integrates well with video platforms like Google Meet or Zoom.
3. Analysis process - step-by-step guide
Once you've prepared your data and selected your AI tool, it’s time to dive into the question of how to analyze interview data. Let’s use Looppanel as an example to walk through this process.
Let’s say you’re running interviews for a soda brand. Your job is to understand what their customers love and hate about the soda product.
- Upload & transcribe your data
.png)
Begin your research journey by either conducting live interviews directly within Looppanel or uploading your existing recordings.
Let’s say you’re speaking to customers on Zoom, Google Meet, or Teams, you can have Looppanel jump in to record those sessions, or upload the files afterwards.
Looppanel starts working immediately, transcribing your audio or video in real-time with over 90%+ accuracy. Unlike other tools that require third-party transcription services, Looppanel has built-in transcription capabilities, streamlining your workflow and saving valuable time.
- Auto-tag your data
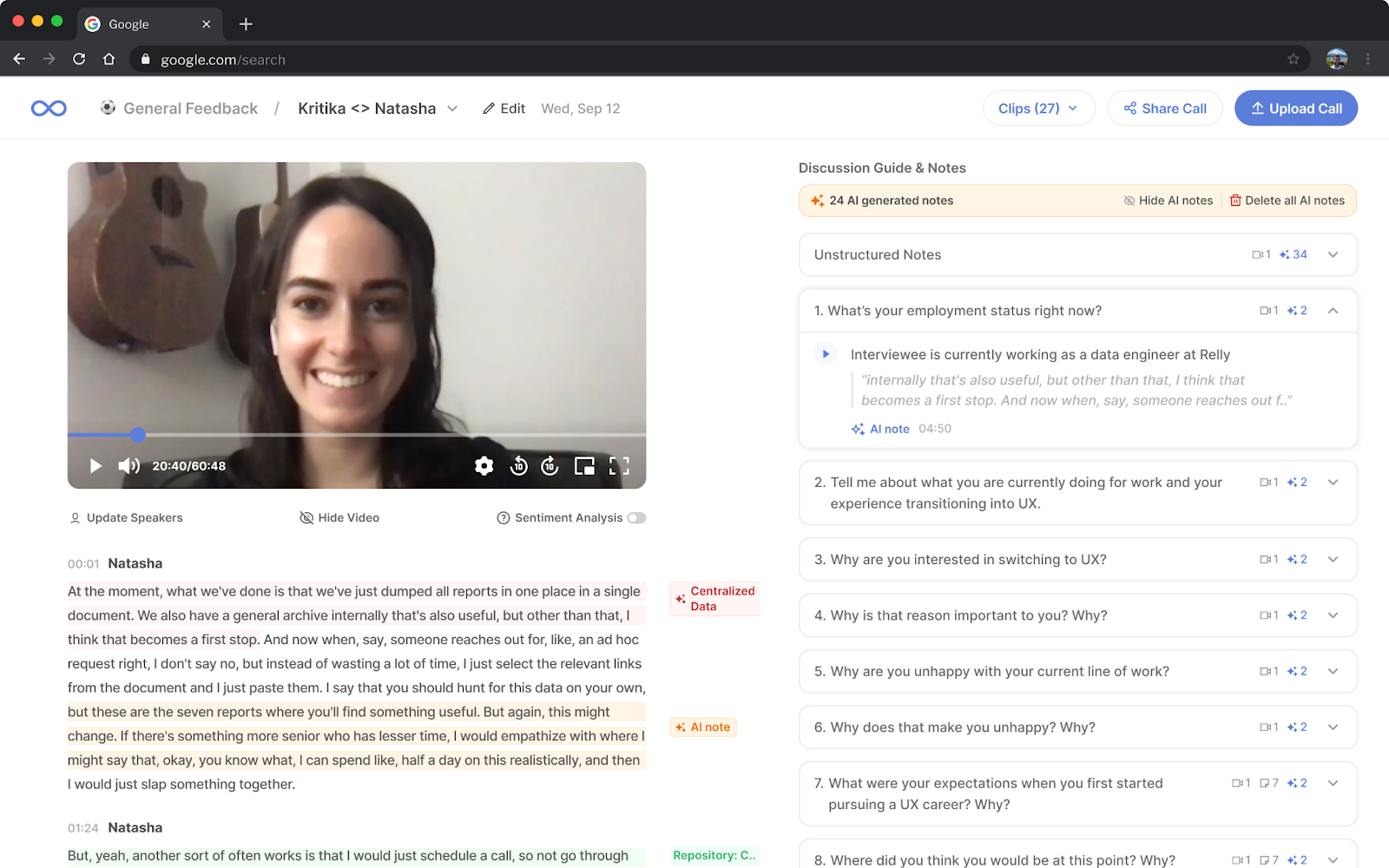
Once your data is transcribed, the next step is to begin coding.
Looppanel works alongside you as a virtual research assistant. It helps you extract answers your participant provided, make notes, and thematically tag your data.
Thematic tagging is run across the project to identify themes from multiple interviews. Let’s say your participants really hated some features of ColoSoda. AI tags may look something like this:
- Product complaint: Too sweet
- Product complaint: Bottle is difficult to hold
- Product complaint: Not available in a can
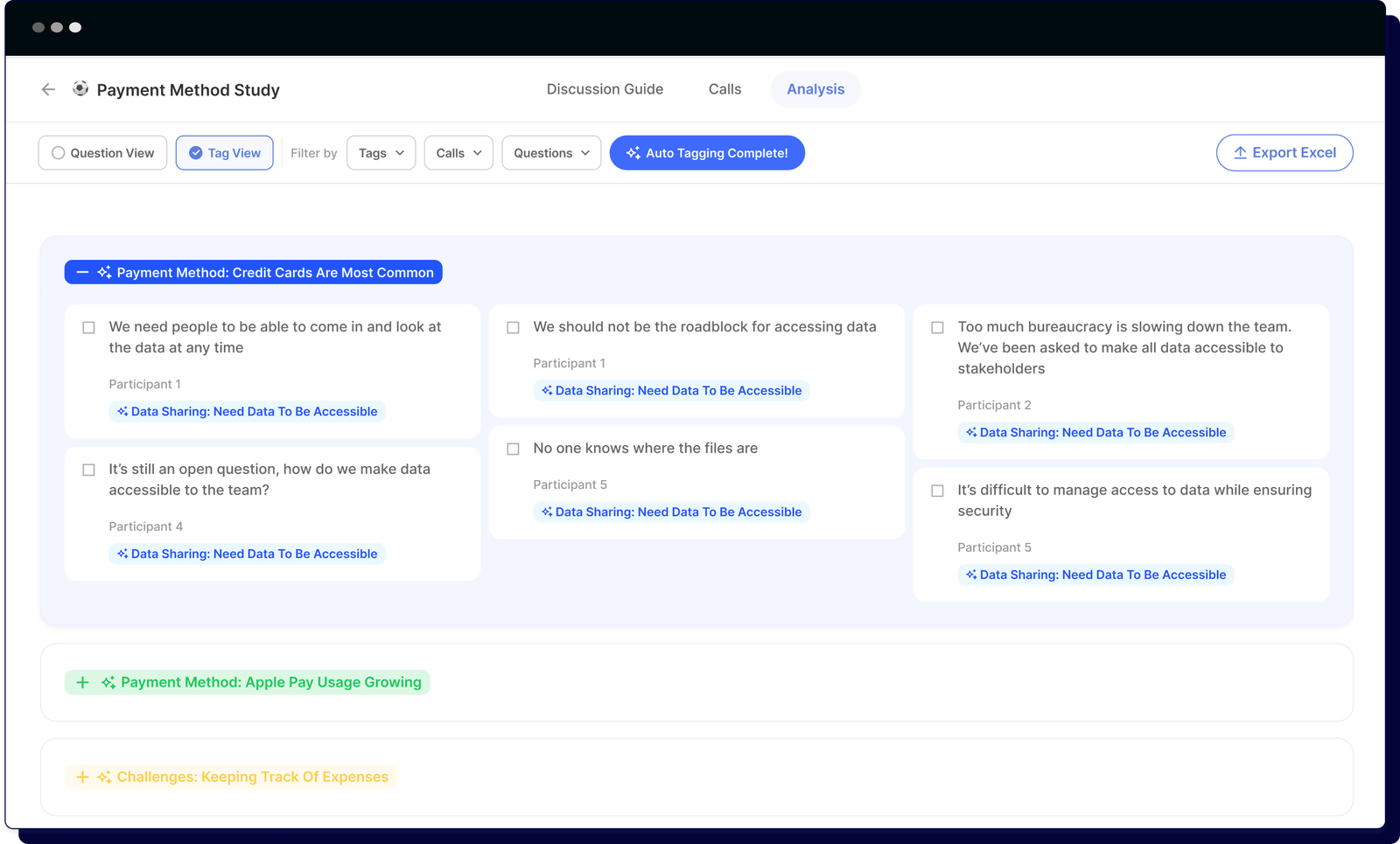
- Review and analyze
While AI is amazing, it’s not perfect. You want to spend some time checking the notes, automatic codes, and adding / editing them for your final analysis.
In Looppanel, you could review all the notes tagged with a theme, delete or rename ones you disagree with and create new codes as required. While manual review is still required, because AI helped you with the first round of tagging, you can now complete tagging and analysis 80% faster.
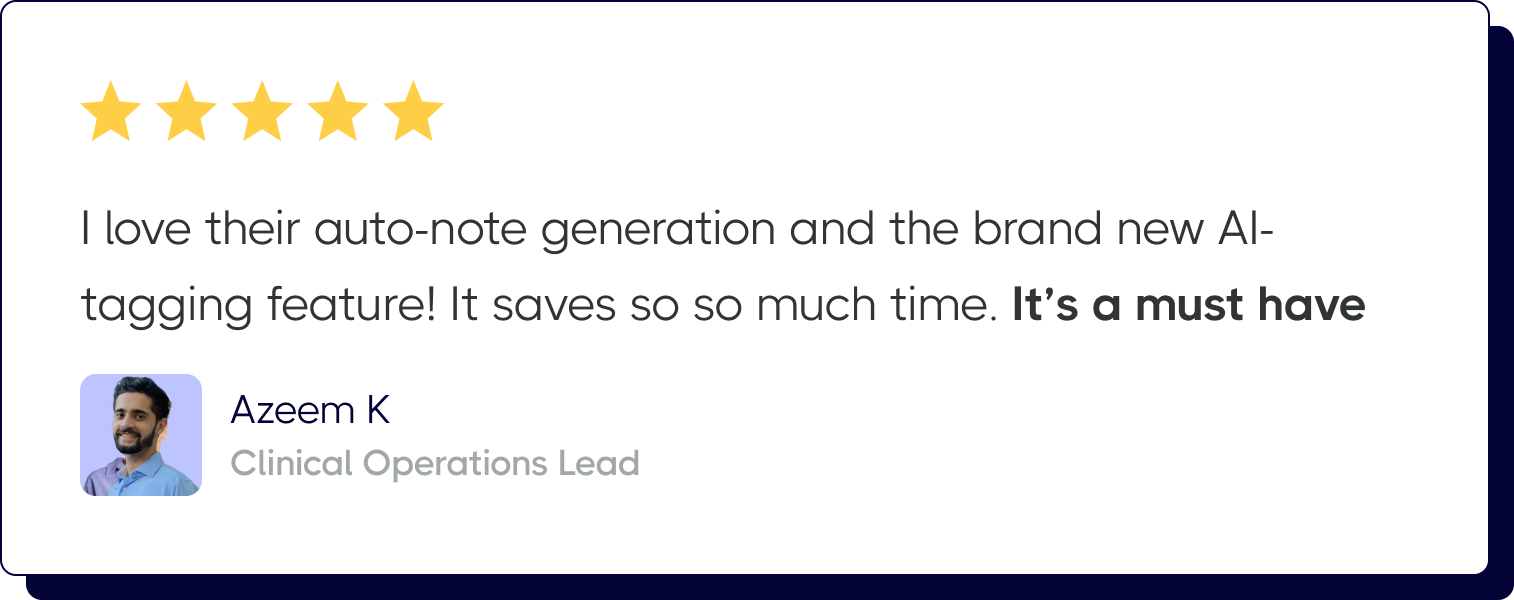
- AI-search for custom questions / quotes

If you’re looking to answer specific questions or find quotes across 5 or 10 or 100 interviews, it can take a while to get through. Having an AI-powered search is a powerful way to ask questions and quickly surface answers and data to your question.
For example, when analyzing our ColoSoda data, you may have a follow-up question for people who didn’t like the bottle shape. Perhaps it’s, “What did people dislike about our bottles?”
Looppanel would give you an AI-summarized answer to this question, as well as all the quotes and data related to it below. This way you can dig deeper into specific parts of your data as well.
- Collaborate and organize
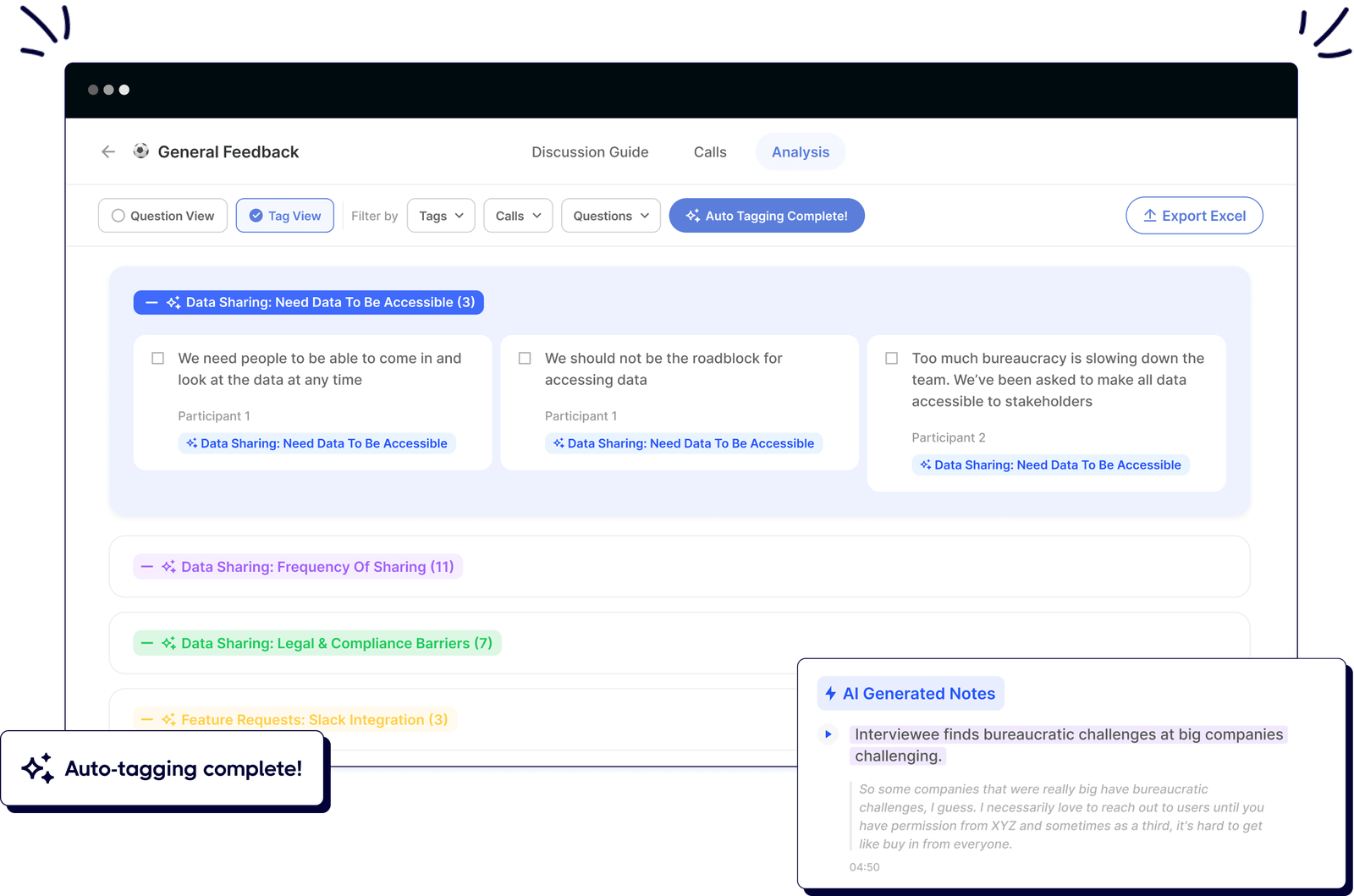
Invite team members to view and analyze interviews together. Sharing the insights from your research or the raw tagged-data view can help get multiple perspectives and align on your final insights.
- Share and export
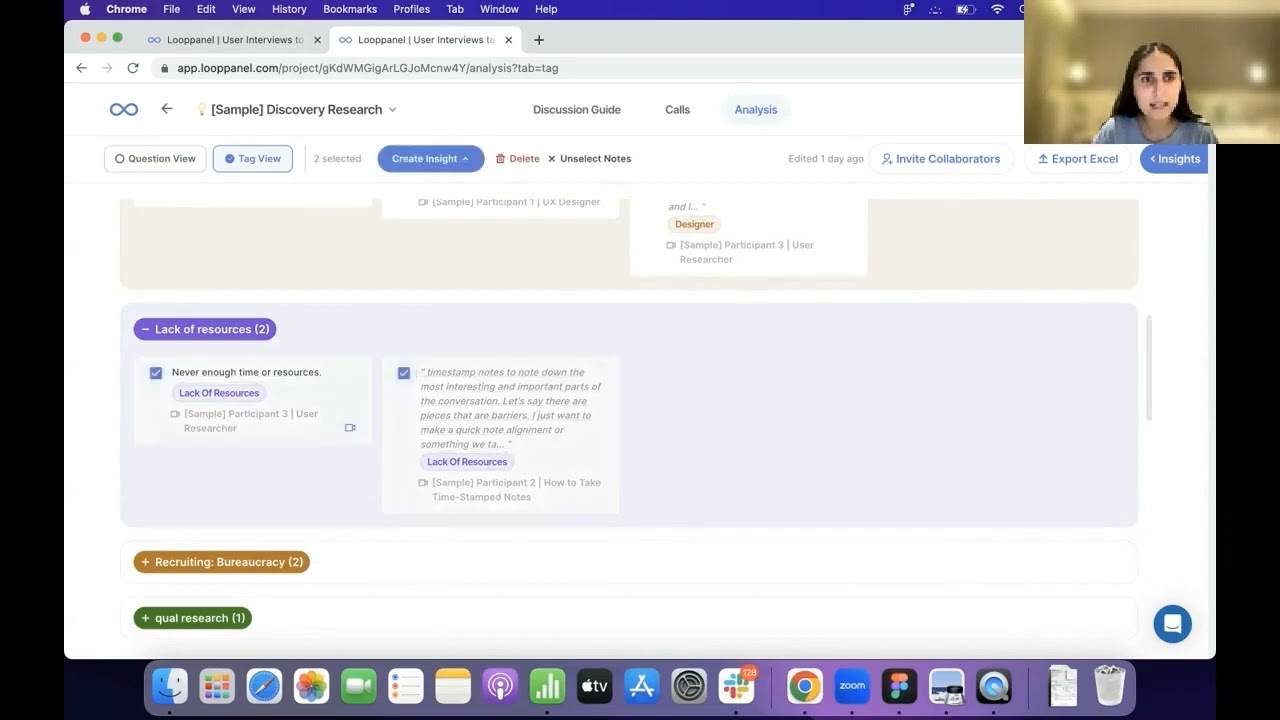
Make your research findings accessible and actionable by creating highlight reels of key interview moments or a sharable report.
On Looppanel, you can select multiple notes and create shareable insights and reports.You can easily embed video clips directly into tools like Notion or Jira, and generate comprehensive reports for stakeholder presentations that showcase the most valuable findings from your research.
Top 5 AI interview analysis tools
Analyzing interview data used to be an exhausting and time-consuming task. But thanks to advancements in artificial intelligence, researchers now have tools that can streamline this process, saving time while providing deeper insights.
1. Looppanel

Looppanel is an AI-powered tool specifically designed for user and market research, enabling businesses and researchers to analyze interview data with speed and accuracy. Its intuitive interface and powerful analysis features make it the go-to choice for those looking to get actionable insights quickly. Whether you’re working with text, audio, or video interview data, Looppanel’s seamless integration ensures a smooth experience.
Key features:
- Multi-format support: Analyze text, audio, and video interview data.
- Sentiment analysis: Identify the emotional tone of responses to gauge sentiment.
- AI Thematic analysis: Automatically categorize responses into themes for better organization.
- Automatic notes: Automatically extract and write notes for your participant responses, organized by interview question so it’s easy to see how each participant responded to one specific topic.
- AI-powered search: Find all the data related to a specific topic or term within a couple of seconds.
Ideal for:
- UX research: Quickly identify pain points or user needs.
- Customer insights: Uncover customer feelings and attitudes towards your brand.
- Market research: Automate the analysis of large interview datasets.
Pricing: Starts at $30/month with a free 15-day trial.
2. Dovetail
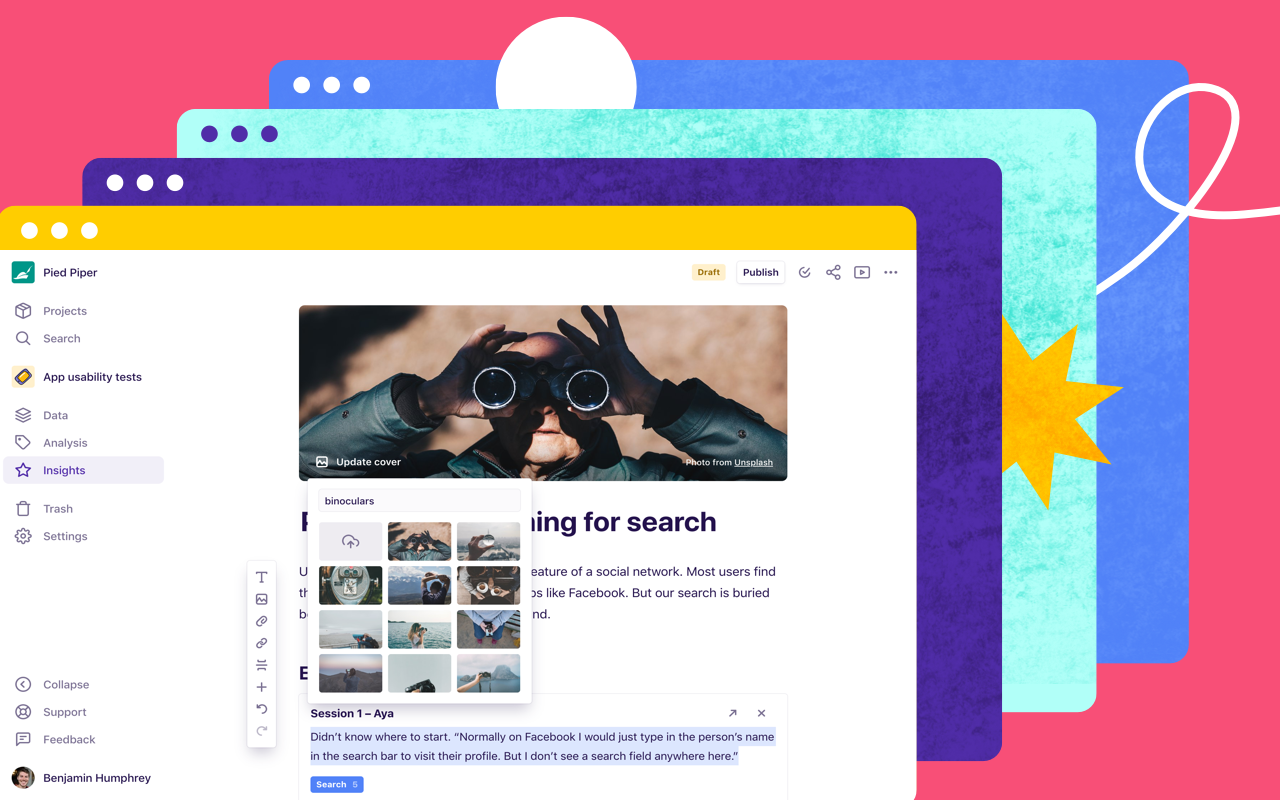
Dovetail brings AI-driven research capabilities to your team, offering robust features for analyzing interviews and organizing insights. With its clean interface and collaborative tools, Dovetail helps teams across departments interpret qualitative data and share findings efficiently.
Key features:
- Collaborative analysis: Easily share findings with your team for collaborative decision-making.
- Automated insights: Use AI to extract insights from interviews and group similar responses.
- Customizable tags: Tag data based on themes or key topics for easier organization.
- Reports and dashboards: Visualize your data with insightful graphs and charts.
Ideal for:
- UX Teams: Ideal for teams looking for a collaborative tool for qualitative research.
- Product Managers: Useful for those wanting to organize user feedback and prioritize feature requests.
Pricing: Dovetail starts at $29 per month for professional users.
3. ChatGPT
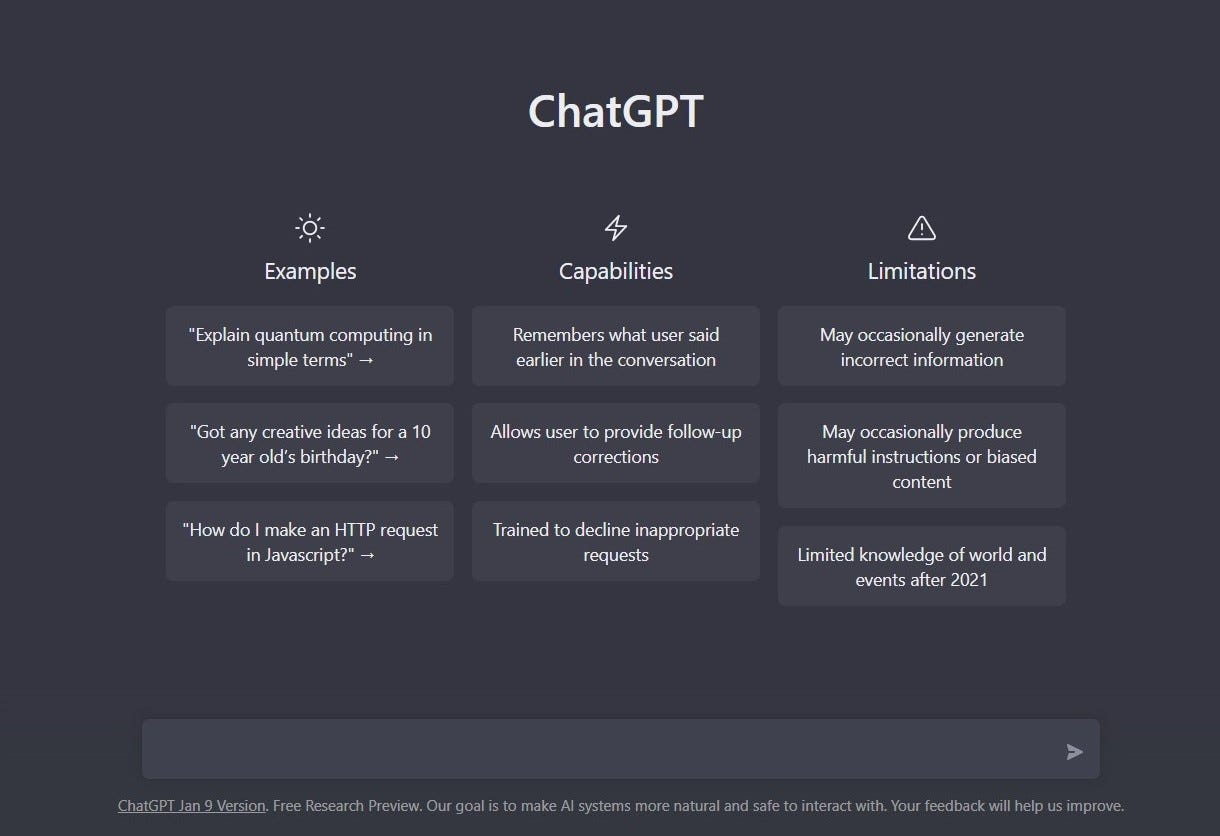
While ChatGPT is primarily known as a conversational AI, it’s also an effective tool for interview analysis. By uploading interview transcripts into ChatGPT, you can ask the AI to summarize responses, extract key themes, or even perform sentiment analysis. It's a flexible option that can be adapted to suit different research needs.
Key features:
- Flexible data input: Works with text-based interview data, offering quick insights.
- Sentiment analysis: Analyze the emotional tone of responses from interviewees.
- Summarization: Generate concise summaries of lengthy interviews.
- Customization: Tailor the analysis based on your research questions.
Quick note, while ChatGPT is great, there are privacy and accuracy concerns when using it. It can hallucinate (aka make stuff up) and also use your data for training its models (security risk) unless you’re careful.
Ideal for:
- Small Research Teams: A great option for teams or individuals who need a flexible and low-cost analysis tool.
- Academic Researchers: Perfect for summarizing large datasets and extracting key themes.
Pricing: ChatGPT offers free basic access, with ChatGPT plus plans starting at $20/per month available for more advanced features and larger datasets.
4. ATLAS.ti
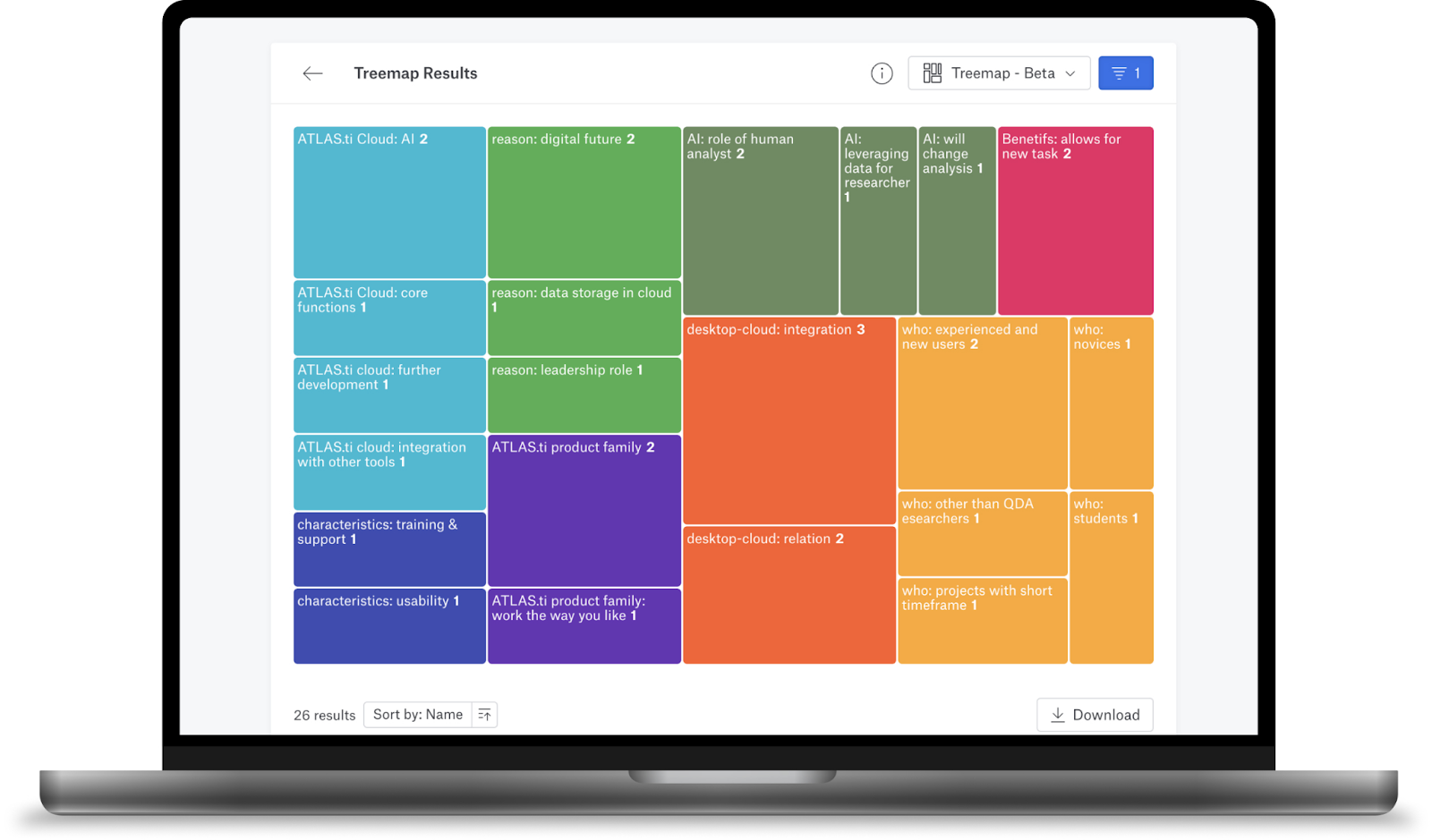
ATLAS.ti is a comprehensive qualitative data analysis tool that leverages AI to analyze and interpret interviews, videos, and audio files. Known for its deep coding capabilities and powerful search functions, ATLAS.ti is often the tool of choice for academic researchers and teams requiring complex qualitative data analysis.
Key features:
- Advanced coding: Automatically code interviews and data into categories.
- Visual tools: Use mind maps and networks to visualize relationships in your data.
- Cross-platform: Works across desktop, web, and mobile platforms.
- AI-powered search: Find patterns and themes in large datasets quickly.
Ideal for:
- Academic Researchers: Best suited for deep qualitative analysis of academic data. MaxQDA is clunky, has a big learning curve, and requires you to do most of the coding manually. It’s best suited for people with long-timelines for analysis. Academics also often have licences available from their universities.
Pricing:
Pricing starts at $23/month for basic researcher plans. Custom pricing available for enterprise users.
5. MAXQDA
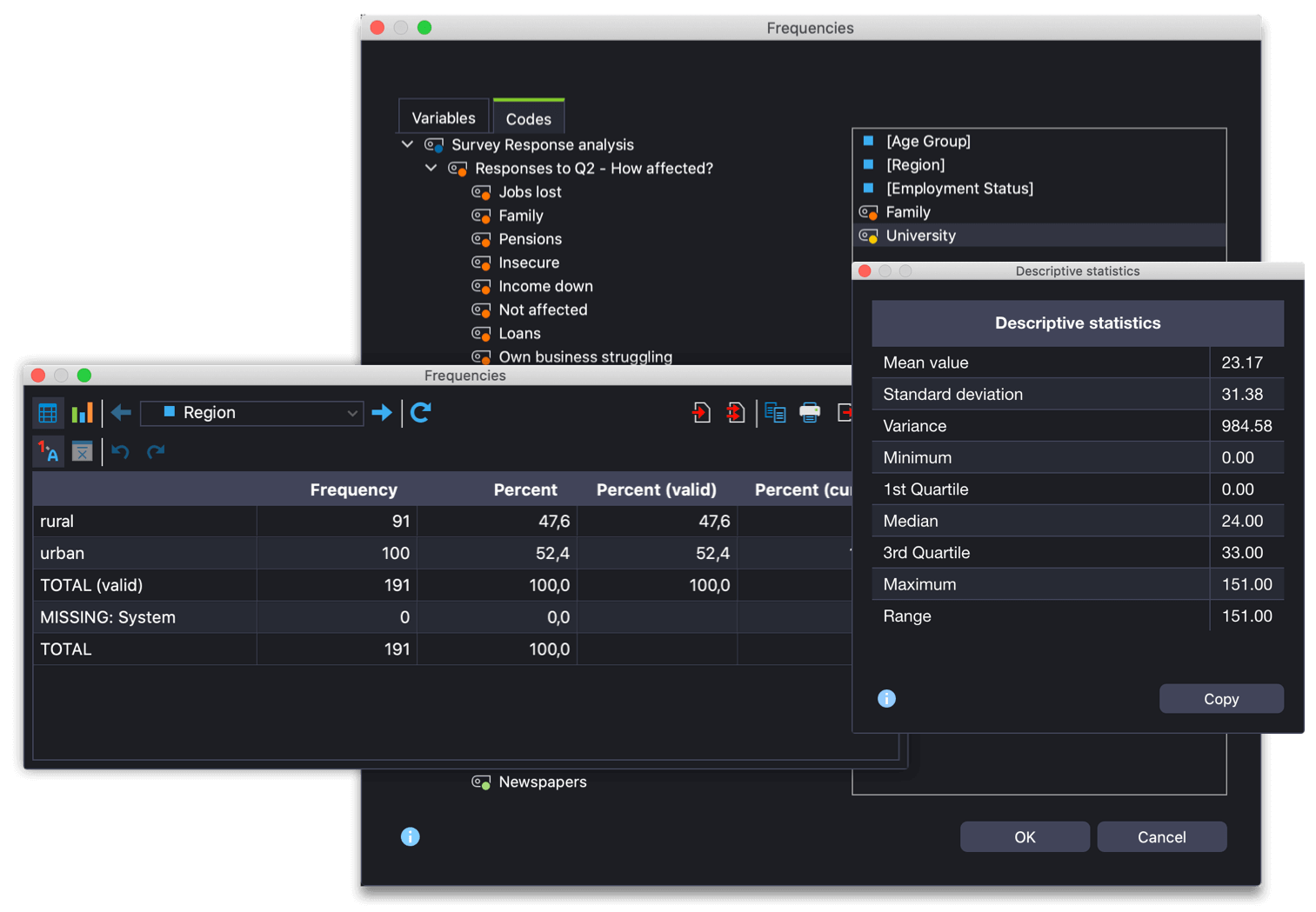
MAXQDA offers tools for analyzing qualitative data, including interview transcripts, audio, and video. With its strong visualization and thematic analysis capabilities, MAXQDA helps users explore large datasets and draw meaningful conclusions from qualitative research.

Key features:
- Multimedia integration: Analyze interview transcripts, audio, and video files in one place.
- Automated coding: Use AI to automatically categorize data and identify recurring themes.
- Rich visualizations: Create charts and graphs to help visualize qualitative trends.
- Data import/export: Easily import or export data from other tools and formats.
Ideal for:
- Academic Researchers: Like MaxQDA, Atlas is also suited for deep qualitative analysis of academic data. It’s difficult to use, has a lot of features, and is very manual—this makes it a good fit for academics with long-term projects.. If you’re in academia, you may also have licences available from their universities.
Pricing: Basic plans starting at €45.
Best practices for AI interview analysis
When using AI for interview analysis, it's important to remember that while AI can handle large datasets and provide insights quickly, it’s not flawless. Following these best practices ensures you get the most accurate and meaningful insights from your research:
- Check codes created by AI and review / edit as required. Especially, watch for missed context and emotional nuances in AI analysis
- Treat AI as an assistant rather than a replacement for human expertise. Implement a human-in-the-loop approach to balance automation with expertise—check AI’s output along the way.
- Make sure you’re aware of security policies of tools. Tools like ChatGPT use customer data to train their models. This is a security risk, especially if you have privacy policies, NDAs, or IRB agreements in place with your participants. If you do decide to use ChatGPT, definitely complete a privacy request on their website to make sure your data isn’t being released onto the internet.
Future of AI interview analysis
The future of AI in interview analysis is incredibly promising. According to Fortune Business Insights, the global data analytics market is projected to reach $529.72 billion by 2030, growing at a compound annual growth rate (CAGR) of 27.3% from 2023 to 2030.
This highlights the increasing reliance on AI for extracting insights from interviews, enabling businesses to streamline research processes, reduce human bias, and uncover deeper patterns in data. As AI technology advances, its capabilities in qualitative research—particularly in analyzing interviews—are set to evolve, offering even more precise and insightful analysis in real-time.
<expect AI to complete change how we analyze interviews. In the future it’ll get better and smarter - quality will go up. There will always be a place for us to review + add human context, but a lot of manual, tedious, time-consuming stuff (E.g., manually tagging data, coming up with code books) will likely be largely automated>.
Use cases and applications of AI in interview analysis
AI interview analysis offers powerful benefits in extracting actionable insights from qualitative data, significantly reducing time and effort while increasing accuracy. By leveraging AI, teams can quickly process vast amounts of interview data, identify key trends, and uncover insights that might be missed in manual analysis.
1. User researchers
User researchers gather qualitative data through multiple research methods. Here's how AI analysis helps with each:
- 1:1 User Interviews: Process dozens of in-depth interviews to identify user needs, pain points, mental models, and behavioral patterns across different user segments. Extract key quotes and themes automatically.
- Moderated Testing: Analyze hours of usability test recordings to identify common task failures, UI confusion points, navigation patterns, and feature discoverability issues across different user types.
2. Market researchers
Market researchers collect qualitative data through multiple formats:
- Group Sessions: Process multiple focus group transcripts simultaneously to identify consensus points, disagreements, and emerging themes across different market segments.
- Individual Interviews: Analyze expert and stakeholder interviews to extract market insights, competitive intelligence, and industry trends. Map relationships between different insights automatically.
3. Customer experience teams
CX teams analyze feedback from various collection methods:
- Support Interactions: Analyze thousands of support call transcripts and chat logs to identify common issues, track problem resolution patterns, and surface emerging technical issues.
- Customer Interviews: Process in-depth customer feedback to understand satisfaction drivers, churn risks, and success factors. Extract actionable feedback for product and service improvements.
Outside of this, AI can be very useful for additional use cases, like:
- Survey Research: Code large-scale open-ended survey responses with AI to understand customer sentiment, brand perception, and purchase motivations across different demographics.
- Secondary Research: Process public comments, reviews, and social media discussions to track brand sentiment, identify emerging issues, and monitor competitive positioning.
- Passive Feedback: Synthesize feedback from multiple channels (surveys, forms, community discussions) using AI-text analysis tools to identify pain points and prioritize improvements.
Conclusion
AI interview analysis is revolutionizing how businesses process and extract insights from qualitative data. With the ability to quickly analyze large volumes of interview data, AI tools are making it easier to uncover patterns and trends that might otherwise be missed.
This shift not only improves efficiency but also ensures more accurate and actionable insights, empowering teams to make better decisions faster. As AI continues to evolve, the potential for smarter, more streamlined interview analysis grows. If you're ready to see the impact of AI on your interview data, book a demo with Looppanel and experience it firsthand.
Frequently asked questions (FAQs)
1. What are your thoughts on using AI to do thematic analysis?
AI can enhance thematic analysis by quickly identifying patterns in interview data. It speeds up the process, offering accuracy and efficiency, but human oversight is still important for nuanced interpretation.
2. What is the best way to analyze interviews?
The best way is to transcribe interviews and use AI tools to identify key themes and insights, saving time and improving accuracy during transcript analysis.
3. Which method is most commonly used to analyze an interview?
Thematic analysis is the most common method to analyze an interview, where AI tools can help quickly identify themes in large sets of interview data.
4. What type of analysis is used for interviews?
Interviews are often analyzed using methods like grounded theory, narrative analysis, or framework analysis. These approaches provide a structured way to interpret complex data, and AI tools can now assist by highlighting patterns more efficiently.
5. How to analyze user interview data?
Begin by organizing your transcript analysis, then apply coding techniques to identify patterns or insights. AI interview analysis tools can simplify this by automatically generating themes and providing visual summaries, saving time on manual interpretation.