Ever spent hours poring over interview transcripts, trying to make sense of all the data? If so, you're not alone. Analyzing transcripts is a crucial part of qualitative research, but it can be time-consuming and overwhelming. That's where AI tools for transcription analysis come in.
AI transcription analysis uses artificial intelligence to help researchers understand and analyze interview transcripts. It's changing the way researchers work with qualitative data, making the process faster and more efficient.
In this article, we'll look at different types of transcripts, what makes up a typical transcript, and how AI can transform your transcript analysis process. We'll also review some popular AI tools for analyzing transcripts and discuss their benefits.
Types of Interview Transcripts
Before we dive into AI analysis, let's look at the different types of interview transcripts you might work with:
- Verbatim transcripts: These capture every word, sound, and pause in the interview. That includes filler words, the "um"s and "uh"s, as well as non-verbal cues like laughter or sighs.
- Intelligent verbatim transcripts: These are cleaned-up versions of verbatim transcripts. They remove filler words and repetitions while keeping the essence of what was said.
- Edited transcripts: These are polished versions of the interview. They correct grammar, remove irrelevant parts, and sometimes rearrange content for better flow.
- Summarized transcripts: These provide a brief overview of the main points discussed in the interview, rather than a word-for-word account.
Each type of transcript serves a different purpose. The type you choose depends on your research goals and the level of detail you need.
Key Components of a Typical Interview Transcript
A well-structured interview transcript usually includes the following:
- Header: This contains basic information about the interview, like date and time, location, names of the interviewer and interviewee, and the purpose of the interview.
- Body: This is the main part of the transcript. It includes questions asked by the interviewer, responses from the interviewee and any follow-up questions or clarifications.
- Time stamps: These mark specific points in the interview. They help researchers find and reference specific parts of the conversation.
- Speaker labels: These show who is speaking at any given time.
- Non-verbal cues: These describe actions or emotions that aren't captured in words, like [laughs], [pauses], or [shows document].
Understanding these components helps when you're working with AI tools to analyze your transcripts.
How is AI used in interviewing?
AI is changing how we do interviews, making the whole process smoother and more insightful. For researchers, AI interview analysis tools are a game-changer. They help create better questions by looking at what worked well in past interviews. During the interview, AI can write down what's being said in real-time, so you can focus on talking instead of taking notes.
Some fancy AI interview analysis tools can even pick up on how people speak and their facial expressions in video calls. After the interview, AI can quickly turn the conversation into text and spot important themes. It can even suggest questions to ask in future interviews. Pretty cool, right?
How to use AI to analyse qualitative data?
Here's how it works: you feed your data (like interview transcripts or survey answers) into an AI tool. The AI then reads through it all and picks out patterns, themes, and feelings in the text.
The great thing about AI interview analysis is that it can spot things in large amounts of data that we might miss. It's also consistent, so it can help reduce bias in your analysis. But remember, AI is here to help, not replace researchers. You still need to use your brain to make sense of what the AI finds and put it in context.
Can AI summarize a transcript?
AI can be great to transcribe audio to text and summarize transcripts.
Is there an AI for transcription? Yes, AI transcription tools have come a long way in recent years. They can now turn audio or video recordings into written text with high accuracy. You can transcribe calls today using specialized transcription software like Otter and Rev, or employ the transcription feature in advanced research tools like Looppanel, Condens and Dovetail.
These research repository tools don't stop at transcription. They can also summarize transcripts, pulling out key points and themes from hours of conversation. That’s where AI transcript analysis really shines!
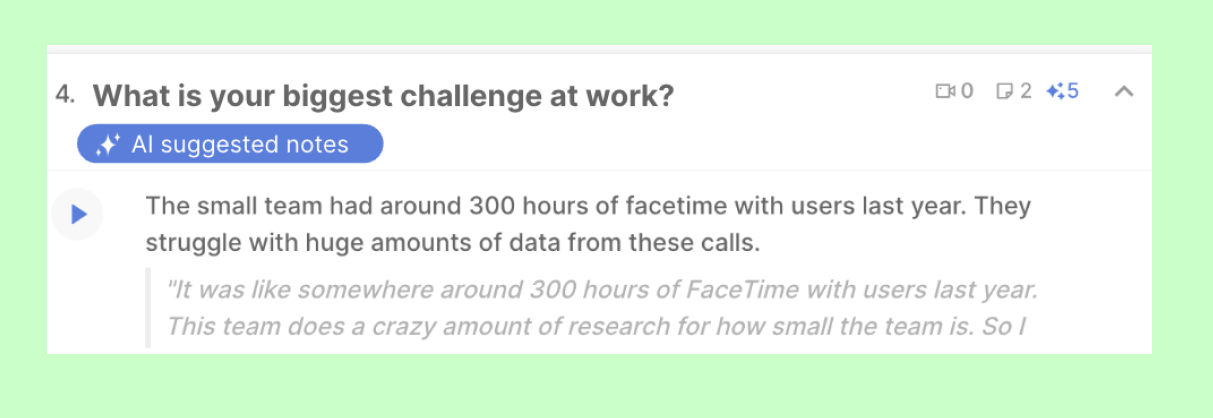
AI summarization tools use natural language processing (NLP) to understand the content of the transcript. They can identify main topics, recurring themes, and even sentiment. Instead of spending hours, this helps researchers quickly grasp the big picture without having to read every word. Researchers already swear by tools like Looppanel for making it 10x easier to go from data to research insights.
Which AI can analyze transcripts?
Several AI tools can analyze transcripts. User research repository tools like Looppanel offer automated notes, sentiment analysis and auto-tagging of data, along with identifying themes, creating word clouds and visualizing patterns in the data.
Some great AI tools to analyze transcripts are Looppanel, Dovetail, Condens, EnjoyHQ and Notably. We’ll review each tool, along with the features and pricing in the next section of the article.
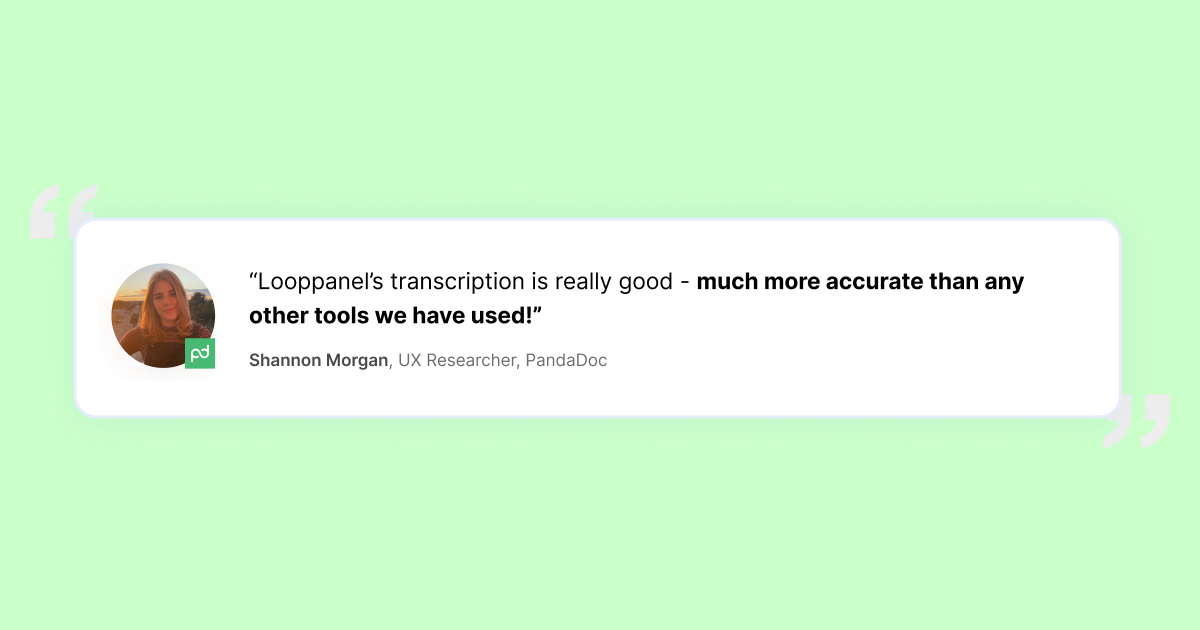
Even if you use extremely advanced AI tools for analysis, it's important to remember that it's just a tool to assist researchers, not replace them. The human touch is still crucial for interpreting results and drawing meaningful conclusions from the data. AI-powered tools can help with the first stage of data analysis, including identifying themes, sentiments and organizing data accordingly. The baton is then handed to human researchers, who can draw meaningful insights that’ll improve their product.
Benefits of AI-Powered Interview Transcript Analysis
AI transcript analysis offers several key benefits.
- Speed: AI can process large amounts of text data much faster than humans. Tools like Looppanel can generate call transcripts, develop automated notes on them and tag the data all in mere minutes, compared to the hours that manual analysis might demand. By automating some of the more tedious aspects of transcript analysis, AI frees up researchers to focus on interpretation and insight generation.
- Consistency: AI applies the same rules and criteria across all your data. This can lead to more consistent analysis, especially when dealing with large datasets.
- Pattern recognition: AI can derive human insights from data (yet). But it excels at spotting patterns and connections. It can identify themes or trends that might not be immediately obvious to human researchers.
- Reduced bias: While AI isn't completely free from bias, it can help reduce some forms of human bias in analysis.
- Scalability: AI tools can handle large volumes of data without breaking a sweat, making it easier to scale up your research projects.
- Easier collaboration: Many AI tools allow multiple researchers to work on the same dataset, making collaboration easier.
Different Types of AI Interview Analysis
When it comes to analyzing interview data, a lot of what AI can do seems like magic. Tedious, long-drawn work like coding your data, and making notes can be sped through in mere minutes, with the right AI research assistant by your side!
Here are a few cool things that AI tools can do under interview analysis.
AI-powered transcription and note-taking
AI has revolutionized this process by offering speedy and accurate transcription across languages and accents. For example, Looppanel’s AI transcription boasts an accuracy rate above 90%!
AI transcription tools can also tell different speakers apart, add punctuation, and also pick out key points, create summaries.
Sentiment analysis and emotion detection
AI can do more than just write down words. It can also figure out how people feel.
Through sentiment analysis, AI tools can code interview responses based on whether the response contains positive or negative sentiments. Emotion detection goes even further. It can pick up on emotion from things like tone of voice, speaking speed and pauses.
This can add a lot of depth to the analysis, and uncover insights that might be missed just by reading the words.
Clustering and categorization
When you have lots of interviews, it can be hard to see the big picture. That's where clustering and categorization come in.
AI can group similar ideas or themes together, by looking for main themes that come up often. topics that are linked to each other and ideas that don't fit the usual pattern
This type of analysis can be a big time-saver. It helps researchers quickly see what's important in a large set of interviews.
Predictive analytics and user modeling
AI can do more than just look at past interviews. It can also make guesses about future behavior.
Predictive analytics uses patterns in the data to forecast what might happen. For example, it might predict how a user might react to a new product, what features people are likely to want next, or which users are at risk of leaving a service.
User modeling goes hand in hand with this. It creates a detailed picture of different types of users based on interview data.
Synthetic user generation
This is a newer and more advanced use of AI in interview analysis. It involves creating "fake" user responses based on real data. There’s a lot of reasonable skepticism about how this might not be ‘real UX’ as it doesn’t involve actual users, but the tech is quite fascinating!
How does it work? The AI learns from many real interviews. Then it can generate new, realistic responses. These aren't real people, but they act like them.
Synthetic user generation and interviews can be handy in running first stage tests on how users might react in new situations, generate more data for analysis, and protect privacy by using synthetic data instead of real user data.
Top Tools for AI Transcript Analysis
Let's dive deep into some top tools that can help you analyze transcripts more efficiently.
Which AI tool is best for an interview?
Let's start with your best option (in our extremely biased opinion, of course :P) Looppanel is an AI-powered research assistant, automating the tedious, manual parts of a researcher’s job.
Why we built Looppanel as a research assistant for audio and video
At Looppanel, we get that dealing with lots of call recordings can be a headache for researchers. So we built Looppanel to be more than just a transcription tool. It's not just a tool that turns speech into text – it's like a smart research buddy that does all the boring stuff for you, freeing you up to focus on the good stuff – finding insights.
Looppanel's approach to AI-based interview analysis
Looppanel's take on AI interview analysis is all about making your job easier. One of our best features? Looppanel organizes your notes based on your interview guide. You can easily jump between topics you're interested in, with all the relevant bits grouped together.
We've also tackled common annoyances in qualitative research. Our AI-powered transcription means you don't have to spend ages fixing transcripts or pay someone else to do it. And while it works great in English, we also offer transcription in languages like Hindi, German, French, Spanish, Portuguese, Dutch, and Italian.
As an AI transcript analysis tool, Looppanel can do the following:
- Generate high-quality transcripts across languages (with over 90% accuracy) in minutes
- Do sentiment analysis with color-coding for questions, positive, and negative responses
- Provide automatic, human-likes notes of your calls, organized by interview questions
- Automatic tag data into common themes and issues.
- Do Google-like search across your workspace to find any data snippet or quote
Looppanel automatically generates notes from your transcript and assigns them to relevant interview questions. For example, if you asked, "Tell me about your role," Looppanel will create notes about the participant's role and organize them under that question.

The 'Analysis' tab allows you to review these notes across every call in your project. The AI can also suggest tags for your data, making it easier to deal with small groups of data rather than countless sticky notes. You can also manually tag your data if you prefer to, and Looppanel can still help out by generating beautiful affinity maps once you’re done!
It’s also incredibly easy to use, no difficult learning curve to dread! Simply sign up, paste in your discussion guide, and upload your calls—Looppanel handles the rest.
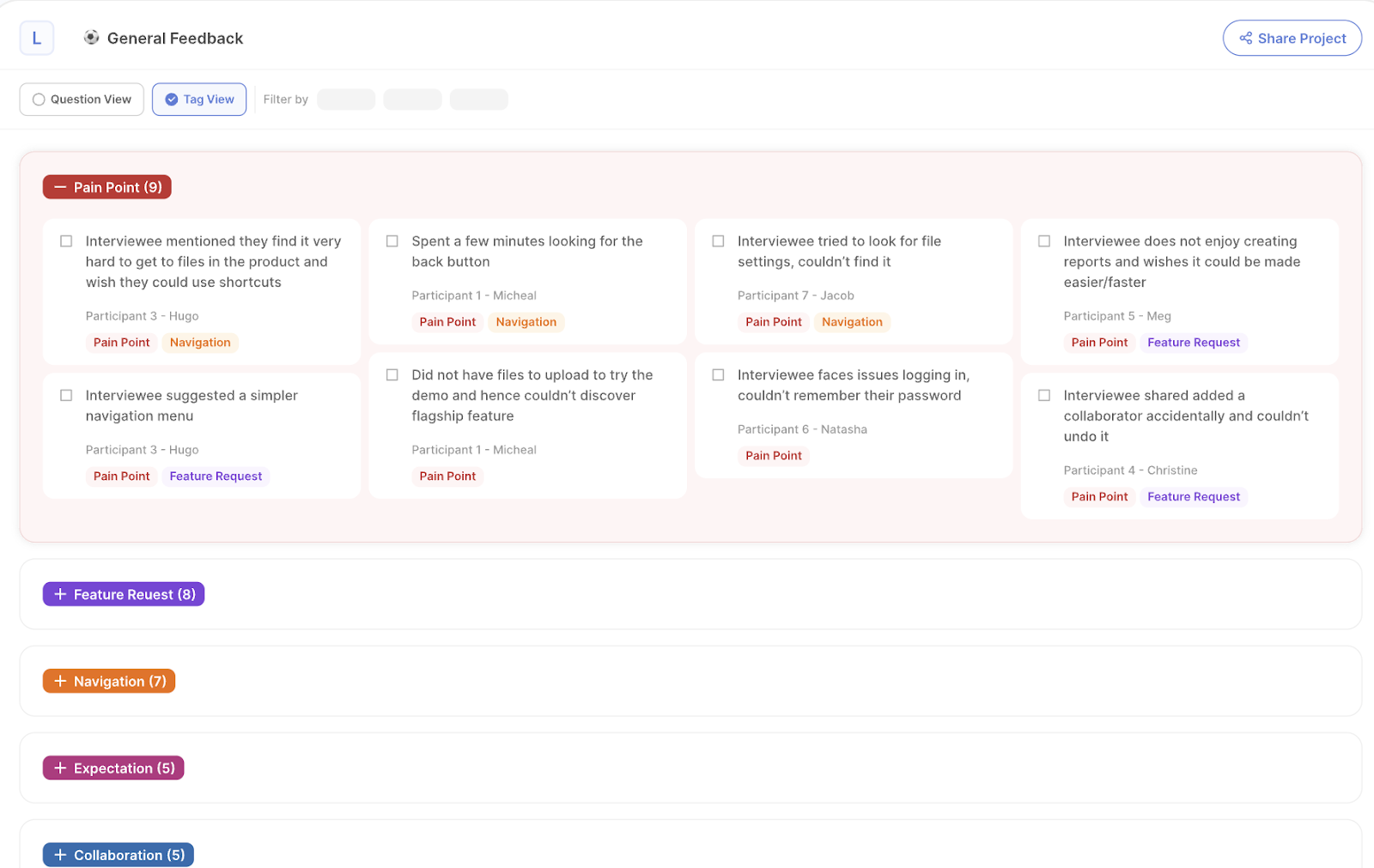
Pricing: Starts at $30 per month, with a free trial available.
ChatGPT

While not specifically built for research, ChatGPT can be a potent tool for transcript analysis, though it requires some adaptation.
Here are a few issues that come up with ChatGPT for AI transcript analysis:
- It can analyze text, but transcripts need to be broken into smaller chunks.
- There is a 4,096 character prompt limit, and requires careful prompt engineering for best results/
- It’s not really integrated into a research workflow/
- Free versions often have poorer quality than paid ones.
If you still want to make the most of ChatGPT for analysis, follow these steps:
- Obtain a high-quality transcript (95%+ accurate)
- Break the conversation into chunks of about 2,500 characters each
- Create and refine prompts for your use case (e.g., "Summarize what Participant 1 is saying in 3-5 bullet points")
- Feed each chunk to ChatGPT with the relevant prompt
- Copy-paste the output to a Miro board or Excel sheet for final analysis
Remember to check the output after every prompt, as ChatGPT can sometimes hallucinate or generate inaccurate information.
Pricing: GPT-3.5 is free, while GPT-4 costs $20 per month. Note that GPT-3.5's quality is significantly lower than GPT-4.
Dovetail
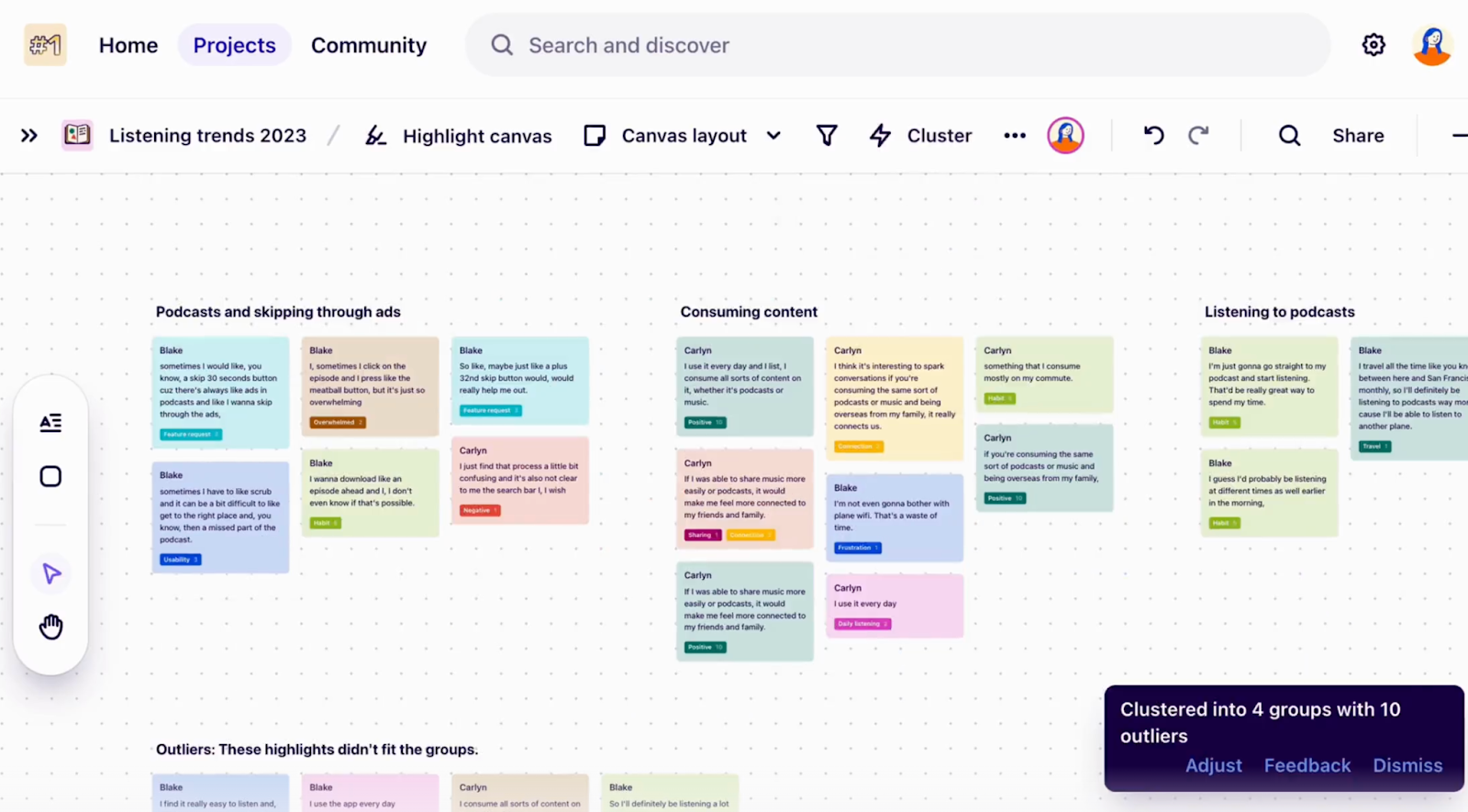
Dovetail is another research repository and analysis platform. While Dovetail still relies on manual tagging, it offers several powerful analysis features like:
- Transcription for user interview call recordings
- Multiple view options for your data, including Board, Table, Cluster, List, Grid
- Accurate, instant, timestamped summaries of video calls
- Magic search, using which you can search by topic, ask questions, and get summarized answers
- Automatic suggestions for key moments in video calls
- Analysis across different channels by helping you track themes in reviews, support tickets, feedback, and more
Dovetail users also appreciate the ability to directly tag video transcripts and quickly find and replay key moments from study sessions.
Pricing: Offers a free plan with limited features, paid plans start at $29/user/month, billed annually.
Condens

Condens is a research repository tool that’s often called “Dovetail lite”. It’s a simple, more user-friendly version of the other platform, although with less capabilities.
Condens also offers several AI-powered features to speed up your analysis including:
- AI-assisted tagging
- Auto-created bookmarks, which detects key topics in lengthy transcripts and notes
- Summarizing core messages from artifacts and highlights in seconds
- Suggestions provided based on existing tags to help uncover connections and trends
- Transcription in 85+ languages with time-stamps and speaker recognition
Condens' features help handle large amounts of data more efficiently and uncover connections that might be missed in manual review. The AI-suggested tags also help prevent the creation of duplicate tags and ensure none are overlooked.
Pricing: Offers a 15-day free trial, with the individual plan at $15 monthly.
EnjoyHQ
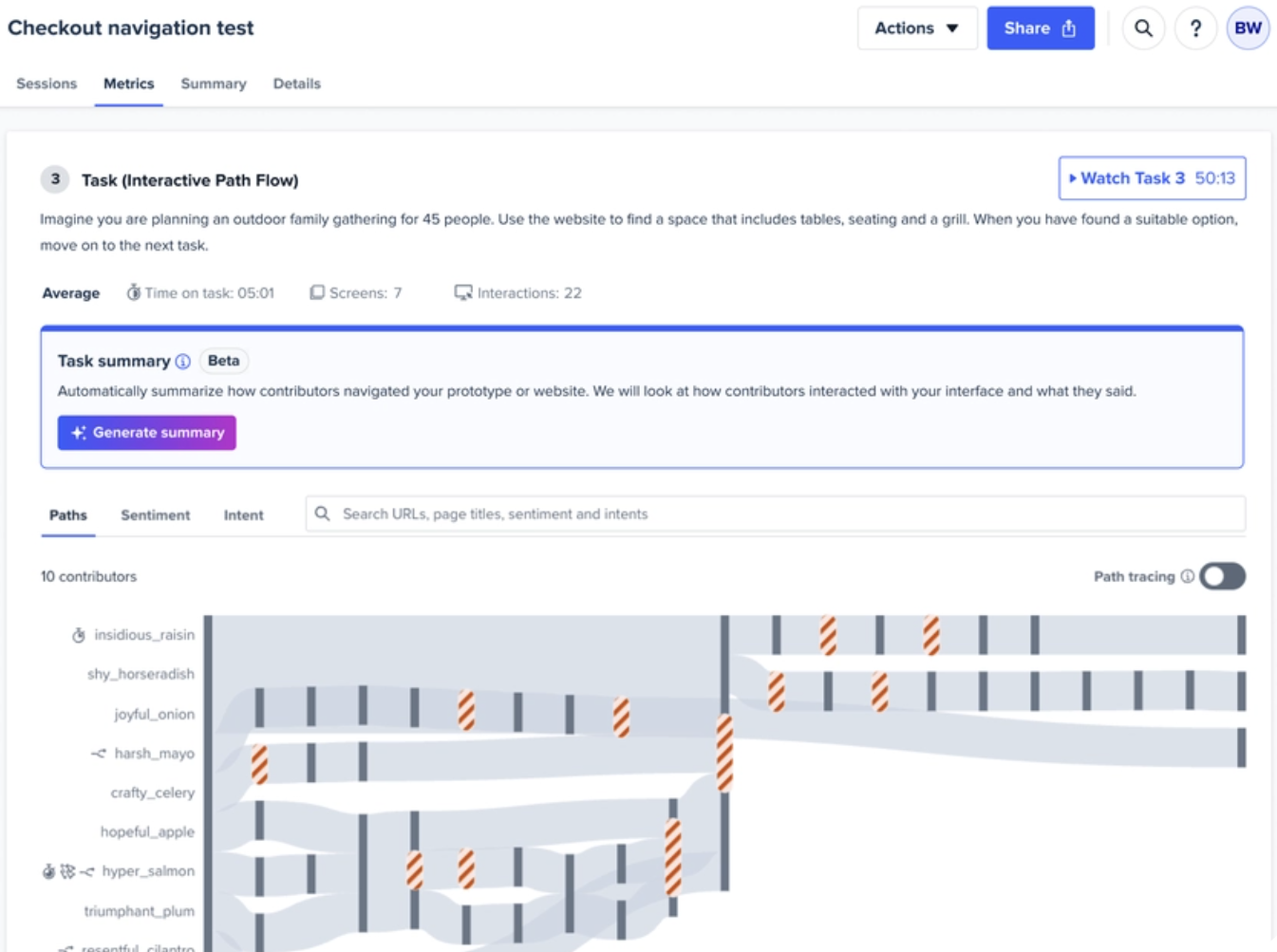
EnjoyHQ has been around for a while and was acquired by UserZoom (which has now been bought by UserTesting.com). Users have found it to be a challenging tool to learn and to use. Most teams don't end up using it for analysis at all, but just as a dumping ground for reports and data points.
Although manual tagging is painful, EnjoyHQ has the ability to set tagging rules (E.g., all text mentioning "integration" should be tagged accordingly). This helps with large volumes of tagging, like when you're dealing with support tickets.
Here are some of EnjoyHQ’s features that help with AI transcript analysis:
- AI Insight summary and AI themes for surveys to instantly identify key insights from verbal tasks or open-ended survey questions.
- Video insights from analyzing real-world interactions, facial expressions, and nuanced insights with an embedded video player
- Interactive visualizations including click paths, sentiment analysis, and keyword maps
- Automatic detection of user friction, by identifying points where users encounter issues or express frustration.
Pricing: Offers a free plan with limited features, contact the EnjoyHQ team to know more about pricing for paid plans.
Notably
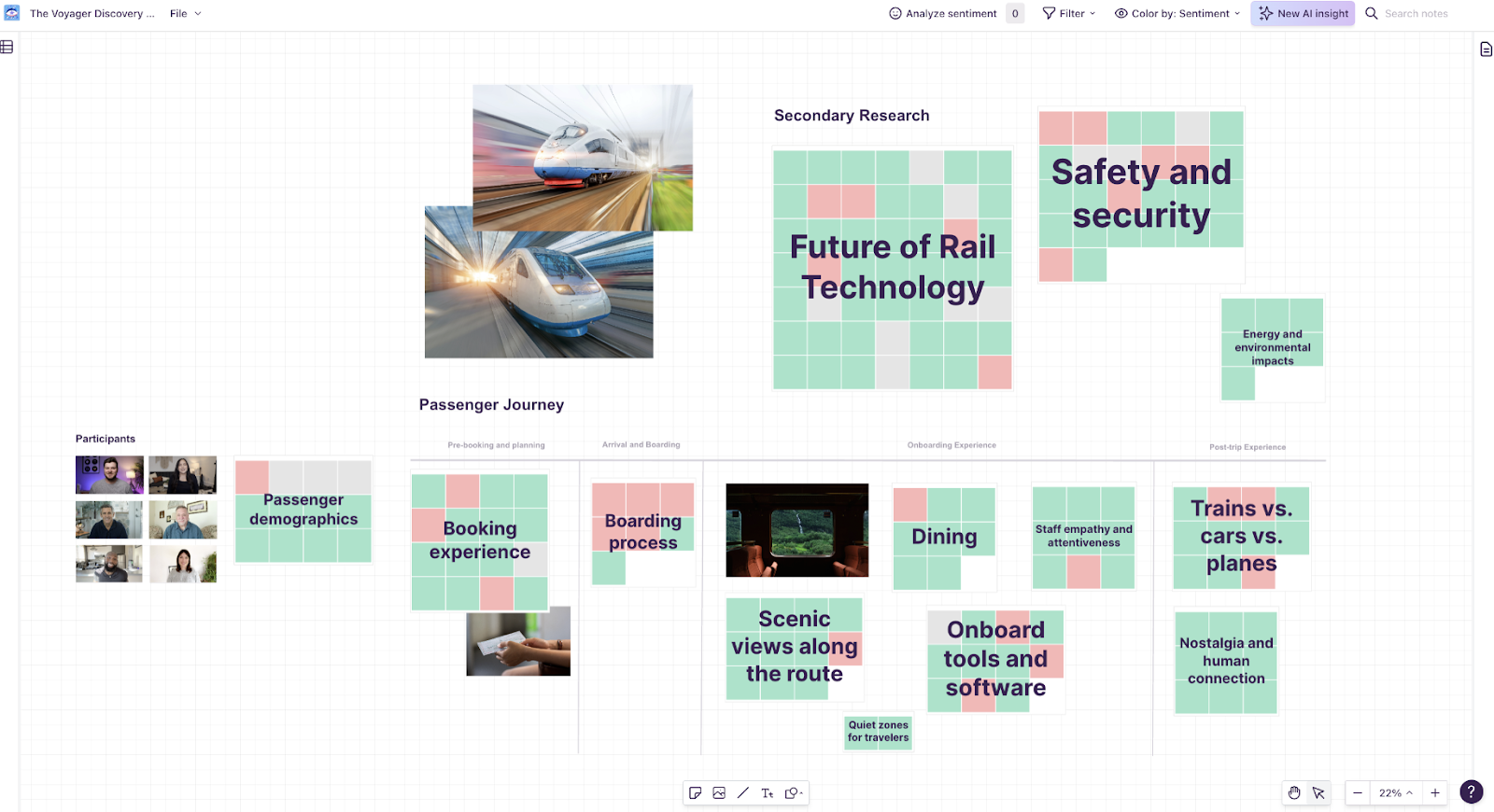
Notably is a research analysis and note-taker software for researchers working with qualitative data like interviews, surveys, and notes. It was originally built as a note-taking tool for students and professionals, and has since expanded to offer more features like transcription of audio recordings, AI summary templates for sharing research insights,and tagging.
Notably offers a range of AI-powered features for transcript analysis including:
- Video transcription, turning recordings into transcripts (up to 5 hours free)
- Transcript summaries, extract important parts of conversations into organized notes and takeaways
- Suggested AI-powered tags. AI learns and improves tag suggestions the more you use them
- Sentiment analysis across highlights from an entire study
- Generative Images from insight content or text input
- Custom AI templates for summaries and reports to stakeholders
Pricing: Pro plan starts at $25 monthly
How to select the right AI tool for interview analysis
When choosing an AI tool for interview analysis, there's no one-size-fits-all solution. The right tool depends on your specific needs, budget, and research goals.
Start by listing your must-have features. Then look at tools that meet those needs. Don't be afraid to try out a few different options before deciding.
As you explore different tools, keep in mind that the field of AI is always changing. New tools and features are coming out all the time. Stay curious and be ready to adapt as the technology evolves.
Here are some key factors to think about:
- Accuracy and reliability
The most important factor is how well the tool works. You need to trust its results.
For transcription tools, check their accuracy rate. Most good tools are 90-95% accurate, but this can vary based on audio quality and accents.
For analysis tools, look at how well it identifies themes, if it can handle different types of language (formal, casual, technical) and how consistent its results are.
It's a good idea to test a tool with some of your own data before committing to it.
- Language support
If you work with interviews in different languages, make sure the tool can handle them.
Consider: which languages does the tool support? How well does it handle accents or dialects? Can it deal with interviews that mix languages?
Some tools are better at certain languages than others. If you often work in a specific language, look for a tool that specializes in it.
- Customization and flexibility
Every research project is different. You need a tool that can adapt to your needs.
Look for features like custom dictionaries for specialized terms, ability to create your own categories or tags, options to adjust sensitivity for sentiment analysis
The more you can customize the tool, the better it will fit your specific research needs.
It’s also important to pick the right pricing plan that fits your team size and stakeholder management requirements. Certain tools like Condens and Looppanel also allow for unlimited stakeholders to share insights with, while others like Dovetail only allows only limited number of viewers within smaller team plans.
- Cost and scalability
AI tools can be expensive, especially for large projects. Think about:
- Does the tool charge per minute of audio, per user, or a flat fee?
- Are there limits on how much you can use it?
- Do you need to pay extra for certain features?
Also consider scalability. A tool that works well for a few interviews might struggle with hundreds. Make sure the tool can grow with your needs.
- Ease of use
A powerful tool is no good if it's too hard to use. Consider: how intuitive is the interface? Is there good documentation or tutorials? How steep is the learning curve?
Remember, you might need to train others to use the tool. A simpler tool might be better if many people need to use it.
- Data privacy and security
Interview data is often sensitive. You need to be sure it's protected.
Where is the data stored and who has access to it? Is the data encrypted? Does the tool comply with regulations like GDPR?
If you work with very sensitive data, you might need a tool that can be installed on your own servers.
- Integration Capabilities
Research rarely happens in isolation. Your AI tool should work well with your other tools.
Can it export data in formats you need? Does it integrate with your analysis software? Can it work with your existing workflow?
Good integration can save a lot of time and reduce errors from moving data between tools.
Challenges and Limitations of AI Tools
While AI analysis tools have revolutionized qualitative research, they're not without their hitches. Let's dive into some key limitations you should keep in mind when using AI for interview analysis.
- AI is your assistant, not a replacement
First things first: AI is here to help, not to take your job. Think of it as a super-smart intern, not a seasoned researcher. It can speed up coding and spot patterns, but it can't replace your years of experience and intuition.
ChatGPT is great at crunching data, but lack the nuanced understanding that comes from being in the trenches of your industry. AI tools can give you a great starting point, but don't take their output as the final word. Always double-check AI-generated data and be ready to add your own insights.
- AI lacks context
Here's the thing: AI tools are like book-smart students who've never left the library. They lack real-world context. They don't know about your company's history, your product's quirks, or your industry's unspoken rules.
This means AI might miss obvious points that you, with your years of experience, would catch in a heartbeat. It's why we at Looppanel make sure you can always trace and edit AI output. You need to be able to check where a note came from, tweak a cluster, or add your own takeaways.
- AI needs supervision
Imagine dumping an entire project's worth of data into AI and expecting fully-formed, ready-to-present insights. Sounds great, right? Well, pump the brakes. AI isn't that smart (yet).
You can't just feed it everything and expect magic. AI needs guidance and supervision. It's great at spotting patterns, but it needs you to make sense of those patterns. It's like having a really smart metal detector – it can find the buried treasure, but you need to figure out what that treasure means.
- AI struggles with the ‘why’s
AI is like a super-observant friend who notices everything but doesn't always get the deeper meaning. It can tell you what users are doing, but it struggles with the "why" behind those actions.
Human behavior is a complex cocktail of personal quirks, cultural norms, and situational factors. AI can spot the patterns in user behavior, but decoding the reasons behind those patterns? That's still your territory. Use AI to observe and highlight themes, but leave the deep analysis and organizational impact to your human brain.
- AI’s output depends on the input quality
Remember, AI is only as good as the data you feed it. If your data is incomplete, inaccurate, or biased, your AI output will be too. It's like trying to bake a cake with half the ingredients – it just won't turn out right.
Make sure you're giving your AI tools complete and accurate data. The more comprehensive and high-quality your input, the more reliable and useful your AI-generated insights will be.
- Data security concerns
When you're using AI tools for qualitative data analysis, you need to be extra vigilant about data security and compliance.
Before signing up for any AI tool, do your homework. Check their terms and conditions, especially around data security. Look for things like:
- Data encryption and secure storage
- Use of summarization models that don't use your data for training
- GDPR compliance for data privacy
For example, at Looppanel here are some of the security measures we take:
🔐 Data is encrypted and stored securely
❌ We only use summarization models that do not use your data for training purposes
🛡️ GDPR compliance for data privacy
If you have questions about Looppanel’s data security measures or what you should watch out for in terms of security, you can always reach out to us at support@looppanel.com.
The human touch is irreplaceable
Remember, in the world of qualitative research, the goal isn't just to collect data – it's to understand people. And understanding people, with all their complexity and quirkiness, is still a uniquely human skill.
Redditors’ opinion on “Are there existing AI tools for coding/theming interview transcripts?”
This question is a frequent discussion point in the researcher Reddit communities.
When it comes to AI tools for coding and theming interview transcripts, Redditors have diverse opinions. Some researchers express skepticism about using AI for this task. One Redditor voiced concern, saying, "Coding might be the last corner of humanity in the research process," highlighting fears that AI might remove the crucial human element from qualitative analysis. They argue that while AI can help with data collection, the real value lies in processing, analysis, and synthesis – areas where they believe AI still falls short, especially in understanding nuanced sentiments.
However, other Redditors are more optimistic about AI's potential in this area. Some mention tools like Looppanel, which uses AI for auto-tagging and generating notes from transcripts. These users suggest that AI can streamline the thematic coding process, though they emphasize it should augment rather than replace human analysis. One Redditor shared success with using AI for thematic analysis in a semi-manual way. Yet, they also point out current limitations, such as restrictions on input size for some AI models and the need for specialized tools to handle large datasets effectively. Overall, while there's recognition of AI's growing capabilities in coding and theming transcripts, most Redditors agree that human oversight and interpretation remain essential in qualitative research.
Conclusion
AI is changing the game in qualitative research. Tools like Looppanel and Condens are making it possible to analyze mountains of data in record time. They're spotting patterns we might miss, summarizing hours of interviews in minutes, and helping us visualize our findings in new ways.
But here's the kicker: these tools are just that – tools. They're incredibly powerful, sure, but they're not magic wands that'll do your job for you. They're more like super-smart assistants, ready to crunch data and spot patterns, but still needing your expertise to make sense of it all.
The key to using AI effectively in qualitative research is to understand its strengths and limitations. Use it to handle the time-consuming tasks of transcription, initial coding, and pattern recognition. Let it help you organize and visualize your data. But don't expect it to replace your analytical skills, your industry knowledge, or your human intuition.
As you explore these AI tools, remember to:
- Always double-check AI-generated insights
- Provide context where AI lacks it
- Use AI for observation, not deep analysis
- Feed it high-quality, comprehensive data
- Stay vigilant about data security
The future of qualitative research isn't about AI replacing researchers. It's about researchers who know how to leverage AI into becoming supercharged versions of themselves. By combining the speed and pattern-recognition capabilities of AI with human creativity and contextual understanding, we can unlock insights faster and more effectively than ever before.
Frequently Asked Questions (FAQs)
What is the AI test for an interview?
When people talk about an "AI test for an interview," they might mean a couple of different things. In the job world, it could be AI tools that screen candidates before human interviews. These might look at written answers, video interviews, or even how someone plays a game.
In research, an AI test for an interview might mean using AI to check how good an interview was. AI interview analysis tools can look at things like how clear the questions were, how in-depth the answers were, or how well the conversation flowed. Some advanced tools can even give tips on how to interview better based on what's worked well before.
How can AI enhance academic research?
AI can give academic research a real boost in several ways. For literature reviews, AI can quickly sift through tons of published work, finding relevant studies and summarizing key points. This can save researchers a lot of time and help ensure they haven't missed anything important.
When it comes to collecting and analyzing data, AI interview analysis tools can help design surveys, clean up data, and run statistics. For qualitative research, AI can help turn interviews into text, code data, and find themes. AI can even help with writing, structuring papers, and suggesting citations. But remember, while AI is a great helper, it can't replace the critical thinking and insights that human researchers bring to their work.