AI content analysis is like having a tireless assistant who can read, watch, and listen to massive amounts of content, then distill it into actionable insights. It's revolutionizing how businesses understand their customers, markets, and competitors. But what exactly is it, and how can it help your business?
In the digital age, businesses face a common challenge—making sense of vast amounts of digital user information. From customer feedback to market research data, the sheer volume can be overwhelming. This is where AI content analysis comes in, transforming how companies understand their customers, markets, and competitors.
This article will explore the world of AI content analysis in depth. We'll look at what it is, how it works, and why it's becoming a crucial tool for businesses of all sizes. Whether you're an experienced UX researcher or just pottering around with qualitative data analysis, this guide will help you understand the potential of AI in analyzing all types of content.
What is content analysis?
Content analysis is a research method used to make sense of large amounts of information. It involves examining text, images, or videos to uncover patterns, themes, and meanings.
Imagine you have thousands of customer reviews. Content analysis helps you sift through them to find common themes, sentiments, and trends. It's not just about counting words - it's about understanding the meaning behind them.
There are two main types of content analysis:
- Quantitative content analysis: This focuses on numbers. How often do customers mention "user-friendly"? What percentage of reviews are positive?
- Qualitative content analysis: This digs deeper into meaning. What emotions are customers expressing? What stories are they telling about their experiences?
Traditionally, researchers did content analysis manually. They would read through documents, coding and categorizing information by hand. This method was time-consuming and often limited to smaller samples.
The process typically involves these steps:
- Define your research question
- Choose your content
- Create a coding scheme
- Code the content
- Analyze the results
For instance, if you're analyzing product reviews, you might code for positive and negative sentiments, specific features mentioned, and overall satisfaction ratings.
The rise of digital content has made content analysis more important than ever. With vast amounts of online data, businesses and researchers need efficient ways to make sense of it all.
This is where AI comes in. With the growth of artificial intelligence and machine learning, we can now analyze huge amounts of content quickly and accurately. This shift to automated content analysis using AI is transforming how we understand and use data.
Can AI do content analysis?
Yes, AI can do content analysis, and it's getting better at it every day. Here’s what it can do:
- Processing large volumes of data quickly: AI can analyze thousands of documents, images, or videos in a fraction of the time it would take a human.
- Identifying patterns and trends: AI is adept at spotting recurring themes or correlations that might be missed by human analysts.
- Categorizing content based on predefined criteria: Once trained, AI can sort content into categories with high accuracy.
- Performing sentiment analysis: AI can determine the emotional tone of text, which is useful for understanding customer feedback or social media reactions.
- Extracting key information: AI can pull out important data points or quotes from large texts.
For example, AI video content analysis can watch hours of footage and identify specific objects, actions, or even emotions. AI website content analysis can crawl through thousands of web pages, categorizing content and spotting SEO opportunities.
However, AI isn't perfect. It can struggle with:
- Understanding complex context: AI might miss nuances that require deeper understanding of cultural or social contexts.
- Interpreting sarcasm or humor: These often rely on subtle cues that AI can misinterpret.
- Making judgments that require ethical considerations: AI lacks the ability to make complex moral decisions.
- Dealing with ambiguity: When content is open to multiple interpretations, AI might struggle to choose the most appropriate one.
That's why the most effective content analysis often combines AI with human expertise. AI can do the heavy lifting of processing and initial analysis, while humans provide the final interpretation and decision-making.
As AI technology continues to advance, its capabilities in content analysis are expanding. Developments in natural language processing and machine learning are enabling AI to understand context and nuance better than ever before.
So, can AI do content analysis? Absolutely. But the real question is: How can we best use AI to enhance our content analysis capabilities? The answer lies in understanding both the strengths and limitations of AI, and using it as a powerful tool to augment human intelligence rather than replace it.
What is generative AI for content analysis?
Generative AI is a highly intelligent assistant who can read, understand, and generate content based on patterns it has learned.
Traditional AI content analysis tools excel at identifying patterns and trends in existing content. Generative AI takes this a step further. Here's what it can do:
- Summarize long pieces of content into concise, readable formats
- Generate new content based on learned patterns
- Create predictive models based on analyzed content
- Offer suggestions and recommendations based on its analysis
For example, let's say you're analyzing customer feedback about a new product. A generative AI tool might not only categorize the feedback but also suggest potential improvements or even draft responses to common issues.
Generative AI uses advanced machine learning techniques like natural language processing (NLP) and deep learning. These allow it to understand context, nuance, and even sentiment in ways that were previously only possible for humans.
Here's how generative AI typically works in content analysis:
- Data Input: The AI is fed large amounts of relevant content.
- Learning: It uses machine learning algorithms to understand patterns, structures, and meanings in the content.
- Generation: Based on what it has learned, the AI can create new content or insights.
- Refinement: Through feedback and further learning, the AI continually improves its output.
While generative AI is powerful, it's important to remember that it's not infallible. It learns from the data it's given, so the quality of its output depends on the quality of its input. That's why human oversight remains crucial in the process.
Types of AI Content Analysis
AI content analysis comes in various forms, each suited to different types of data. Let's explore the four main types:
AI Text Analysis
AI text analysis uses natural language processing (NLP) to understand and interpret written content. This could be anything from social media posts to customer reviews or long-form articles.
Can AI analyze an article? Yes, AI can analyze articles. It can summarize key points, identify themes, and even gauge the article's tone. For instance, AI might analyze a news article to determine if it's positive, negative, or neutral towards a topic.
How can AI analyze website content? Yes indeed! AI can crawl websites, examining text, metadata, and structure. It can assess readability, SEO optimization, and content relevance. AI website content analysis can help improve user experience and search engine rankings.
AI Video Analysis
Video content analysis AI is becoming increasingly sophisticated. It can process visual and audio elements in videos, offering insights that were once time-consuming to obtain manually.
Is there an AI that can analyse a video?
Yes, several AI tools can analyze videos. They can identify objects, recognize faces, track movement, and even understand context. This is useful in fields like security, sports analysis, and marketing.
Can ChatGPT analyse video content? As of now, ChatGPT itself can't directly analyze video content. However, it can work with text descriptions or transcripts of videos. For full video analysis, specialized AI video content analysis tools are needed.
AI Image Analysis
AI image analysis can identify objects, detect colors, recognize faces, and even interpret emotions in images. This type of analysis is useful in fields like medical imaging, social media monitoring, and quality control in manufacturing.
AI Audio Analysis
AI can analyze audio content, including speech recognition, sentiment analysis in spoken words, and even detecting emotions from voice tone. This is valuable for transcription services, call center analytics, and music classification.
These four types of AI content analysis often work together in automated content analysis software. For example, a tool might analyze a video by processing its visual elements, transcribing and analyzing the audio, and examining any text overlays.
How to do efficient AI content analysis: 7 steps
Let's break down the process of AI content analysis into seven steps:
1. Define Your Objective
Start by asking: What do you want to learn? Your goal guides everything that follows. Maybe you want to understand customer sentiment about a new product. Or perhaps you're looking to spot trends in your competitors' content. A clear objective helps you choose the right data and tools.
2. Collect and Prepare Content
Next, gather the content you'll analyze. This could be social media posts, customer reviews, or your own blog articles. Make sure your data is in a format your AI tool can use. You might need to clean up messy data or convert files to the right format.
3. Choose the Right AI Tools
Pick tools that fit your needs. Are you doing text analysis? Video content analysis? Or maybe you need cross-modal analysis that combines different types of data? Choose tools that can handle your content type and deliver the insights you need.
4. Run the Analysis
Now it's time to let the AI do its work. Your AI tool of choice will process your content, looking for patterns and insights based on your objectives. This step is mostly automated, but you might need to adjust settings or provide additional guidance to the AI.
5. Interpret the Results
Once the AI finishes its analysis, you'll get a set of results. These might be in the form of charts, summaries, or data points. Your job is to make sense of these results. What do they mean for your business? How do they answer your initial questions?
6. Validate and Refine
Don't take the AI's findings as gospel. Check the results against your knowledge and other data sources. If something seems off, you might need to refine your analysis. This could mean adjusting your AI settings, using a different tool, or adding more data.
7. Act on the Insights
Finally, use what you've learned to take action. Maybe you'll tweak your content strategy, adjust your product based on customer feedback, or start a new marketing campaign. The key is to turn your AI-powered insights into real-world improvements.
Remember, AI content analysis is a powerful tool, but it's not magic. It works best when combined with human expertise and judgment.
Benefits of AI content analysis
AI content analysis offers numerous benefits that can transform how businesses understand and use their data. Let's explore some of the key advantages:
1. Speed and Efficiency
AI can process vast amounts of content in a fraction of the time it would take humans. This speed allows businesses to analyze more data, more quickly, leading to faster insights and decision-making. For example, an AI system could analyze thousands of customer reviews overnight, providing valuable insights by morning.
2. Scalability
As your data grows, AI grows with it. Whether you're analyzing hundreds or millions of data points, AI can handle the load without breaking a sweat. This scalability makes AI content analysis particularly useful for large enterprises or fast-growing companies dealing with ever-increasing amounts of data.
3. Consistency
Unlike humans, AI doesn't get tired or distracted. It applies the same criteria consistently across all content, reducing bias and improving reliability. This consistency is crucial when analyzing large datasets where maintaining uniform standards is challenging for human analysts.
4. Pattern recognition
AI excels at spotting patterns that might be invisible to the human eye. This ability can lead to unexpected insights and discoveries in your data. For instance, AI might identify subtle correlations between customer satisfaction and specific product features that weren't previously apparent.
5. Multi-format Analysis
AI can analyze text, images, audio, and video, often simultaneously. This versatility allows for a more comprehensive understanding of your content landscape. For example, AI could analyze both the text and imagery of social media posts to gain a fuller understanding of brand perception.
6. Real-time Analysis
Many AI systems can perform analysis in real-time, allowing businesses to respond quickly to emerging trends or issues. This capability is particularly valuable in fast-moving industries or crisis management situations.
7. Cost-effective
While there's an initial investment, AI can significantly reduce the long-term costs associated with manual content analysis. By automating time-consuming tasks, businesses can allocate human resources more efficiently.
8. Predictive capabilities
Some AI systems can use historical data to make predictions about future trends or outcomes. This predictive analysis can help businesses anticipate market changes, customer needs, or potential issues before they arise.
9. Objective analysis
AI doesn't have personal biases or preconceptions that can influence analysis. This objectivity can lead to more accurate and unbiased insights, particularly when dealing with sensitive or controversial topics.
Whether you're in marketing, product development, customer service, or any other field that deals with large amounts of data, AI content analysis can provide valuable insights to drive your strategy and decision-making.
However, it's important to remember that while AI content analysis offers many benefits, it's not a magic solution. It's a tool that, when used effectively and in conjunction with human expertise, can significantly enhance your ability to understand and act on your data. The key is to understand both its capabilities and limitations, and to use it as part of a broader, strategic approach to data analysis and decision-making.
Potential challenges and limitations of AI in content analysis
While AI content analysis offers numerous benefits, it's not without its challenges and limitations. Understanding these is crucial for using AI effectively and responsibly in your content analysis efforts.
Data Quality Issues
AI systems are only as good as the data they're trained on. Poor quality data can lead to inaccurate or biased results. Ensuring clean, representative data is an ongoing challenge. For example, if an AI is trained on customer feedback that only represents a small segment of your customer base, its insights may not be applicable to your entire customer population.
Contextual Understanding
AI can struggle with nuanced language, sarcasm, or cultural references. It may misinterpret content that requires deeper contextual understanding. This limitation can be particularly problematic when analyzing social media content or informal communication, where context is often crucial to understanding the true meaning.
Bias in AI Systems
AI systems can inadvertently perpetuate or amplify biases present in their training data. This can lead to skewed analysis and unfair outcomes. For instance, if an AI system is trained on historical hiring data that reflects gender bias, it may perpetuate this bias in its analysis of job applications.
Lack of Creativity
While AI is great at pattern recognition, it may miss novel insights that require creative thinking or "out-of-the-box" perspectives. AI can tell you what is, but it may struggle to imagine what could be. This also underscores the continued importance of human creativity in the analysis process.
Privacy Concerns
AI content analysis often involves processing large amounts of data, which can raise privacy issues, especially with personal or sensitive information. Ensuring compliance with data protection regulations like GDPR can be challenging when using AI for content analysis.
Regulatory Compliance
As AI use grows, so does regulation around it. Keeping up with and complying to evolving AI regulations can be challenging. This is particularly true for businesses operating across multiple jurisdictions with different regulatory frameworks.
Explainability Issues
Some AI systems, particularly deep learning models, can be "black boxes," making it difficult to explain how they reached their conclusions. This lack of transparency can be problematic in situations where clear justification for decisions is required.
Integration Challenges
Incorporating AI content analysis into existing workflows and systems can be complex and time-consuming. It often requires changes to established processes and may face resistance from employees accustomed to traditional methods.
Ethical Considerations in AI Content Analysis
As AI content analysis becomes more widespread, it's crucial to address the ethical challenges it poses.
Data permission and privacy
Before using AI to analyze content, companies must get consent to use consumer information. This applies whether they collect data directly or buy it from others. Why does this matter? Many people worry about how companies might misuse their data. This concern can harm a brand's reputation and erode customer trust.
Bias in AI tools
AI tools learn from human-created content. If that content has biases, the AI might make unfair or incorrect conclusions. This is a significant ethical issue in AI content analysis.
To address this, organizations need to carefully check AI results before acting on them. Human oversight is key to spotting and correcting potential biases.
Transparency and explainability
When using AI for content analysis, it's important to be clear about how the AI works. Users should understand what the AI can and can't do. They should also know how it reaches its conclusions.
This transparency helps build trust. It also allows users to make informed decisions based on AI-generated insights.
Accountability
Who's responsible when AI content analysis goes wrong? Think of the recent furore caused by Figma’s new AI tool generating designs that were Apple app lookalikes, for example. As AI becomes more complex, companies using AI for content analysis should have clear policies on accountability.
Best Practices for Efficient AI Content Analysis
To get the most out of AI content analysis, here are some tried and tested practices to follow:
Define Clear Objectives
Before starting, know exactly what you want to achieve. Are you looking for trends in customer feedback? Or trying to spot inefficiencies in your content strategy? Clear goals help you choose the right data and tools.
Use High-Quality Data
The saying "garbage in, garbage out" applies to AI content analysis. Make sure your data is accurate, relevant, and diverse. This helps avoid biased or misleading results.
Choose the Right AI Tools
Not all AI tools are created equal. Pick ones that fit your specific needs. Consider factors like the type of content you're analyzing and the insights you're after.
Combine AI with Human Expertise
AI is powerful, but it's not perfect. Use it to enhance, not replace, human analysis. Human experts can provide context and catch nuances that AI might miss.
Interpret Results Carefully
Don't take AI insights at face value. Always interpret results in the context of your business and industry knowledge.
Ensure Ethical Use
Follow ethical guidelines in all your AI content analysis. This includes respecting privacy, avoiding bias, and being transparent about AI use.
Certainly. I'll write a section on the top 5 tools for AI content analysis based on the information provided, following the writing style you've described.
5 Top Tools for AI Content Analysis
Wondering which AI content analysis tools can supercharge your research? Let's explore five standout options that user researchers and businesses are using to gain deeper insights from their data.
1. Looppanel
Pricing: Starts at $30 per month, with a free trial available.
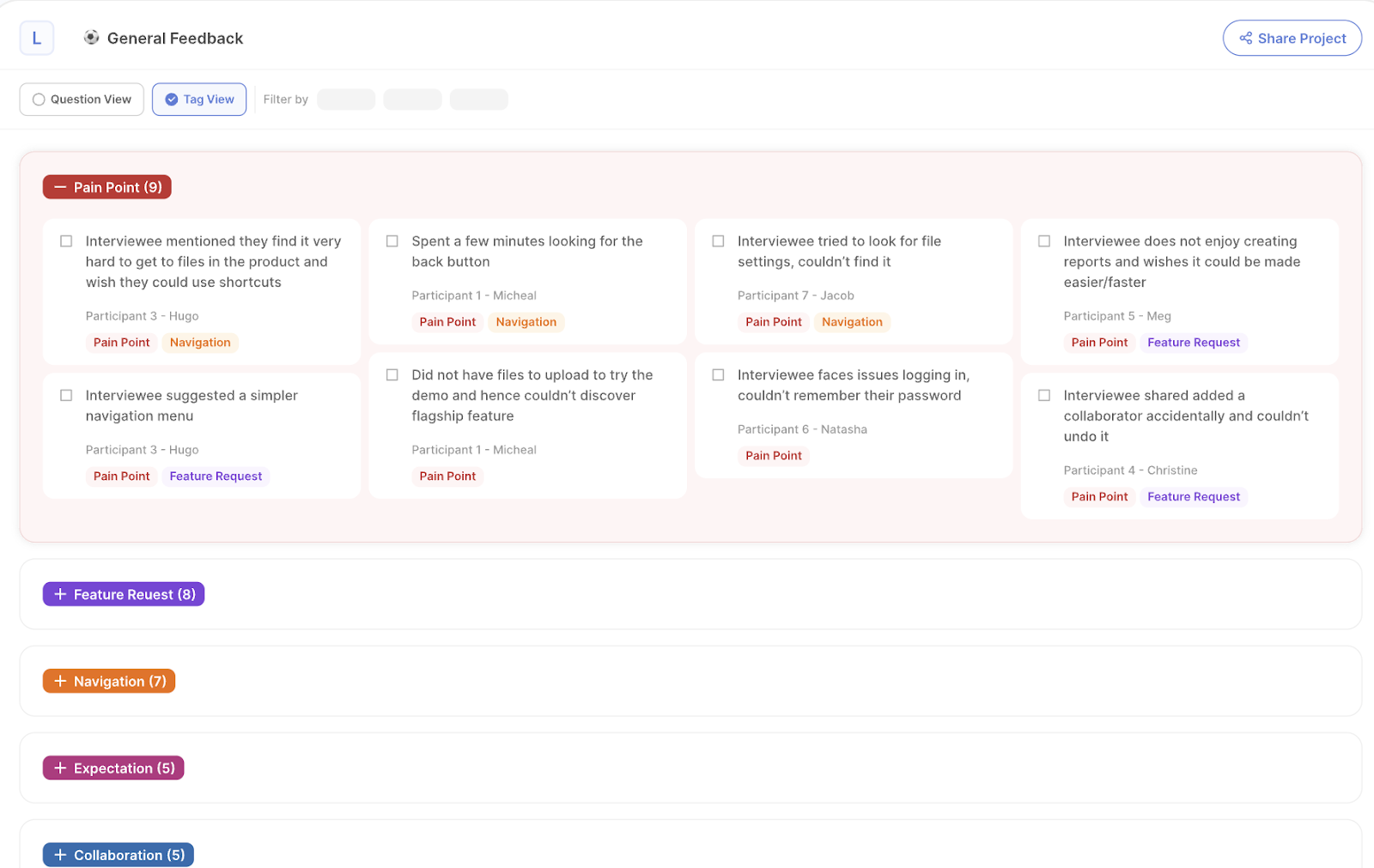
Looppanel is an AI-powered research assistant, automating the tedious, manual parts of a researcher’s job.
As an AI content analysis tool, Looppanel can do the following:
- Generate high-quality transcripts across languages (with over 90% accuracy) in minutes
- Do sentiment analysis with color-coding for questions, positive, and negative responses
- Provide automatic, human-likes notes of your calls, organized by interview questions
- Automatic tag data into common themes and issues.
- Do Google-like search across your workspace to find any data snippet or quote
Looppanel automatically generates notes from user interview call recordings and transcripts, and assigns them to relevant interview questions. For example, if you asked, "Tell me about your role," Looppanel will create notes about the participant's role and organize them under that question.

The 'Analysis' tab allows you to review these notes across every call in your project. The AI can also suggest tags for your data, making it easier to deal with small groups of data rather than countless sticky notes. You can also manually tag your data if you prefer to, and Looppanel can still help out by generating beautiful affinity maps once you’re done!
It’s also incredibly easy to use, no difficult learning curve to dread! Simply sign up, paste in your discussion guide, and upload your content—Looppanel handles the rest.
2. Hey Marvin
Pricing: Offers a free plan with limited features, paid plans start at $50/user/month.
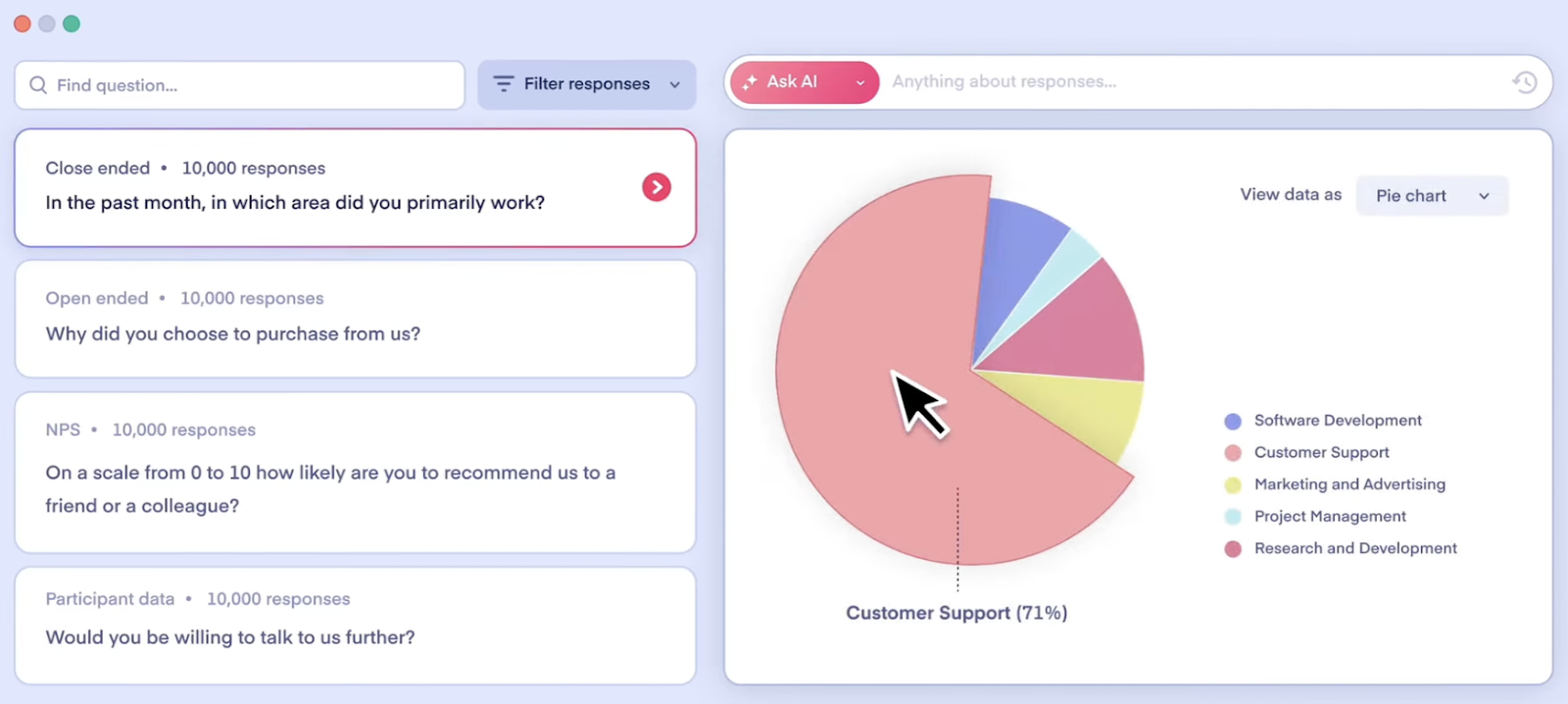
Marvin is a research repository and analysis tool that’s designed specifically for researchers.
Within AI content analysis, Marvin offers the following capabilities:
- Thematic analysis by automatically quantifying qualitative data and groups key themes
- Measures sentiment to understand user feelings
- Identifies key patterns and emerging trends across all notes
- Allows quick finding of answers and quotes across the entire research repository, with an Ask AI feature.
Marvin shines when you're dealing with surveys and interviews. It can sort responses into themes, though you'll need to upload data manually. Once your data's in, Marvin offers clear visuals to help you share findings with your team.
The Ask AI feature is particularly useful for summarizing, searching, and finding patterns in surveys and interviews.
3. Dovetail
Pricing: Offers a free plan with limited features, paid plans start at $29/user/month, billed annually.
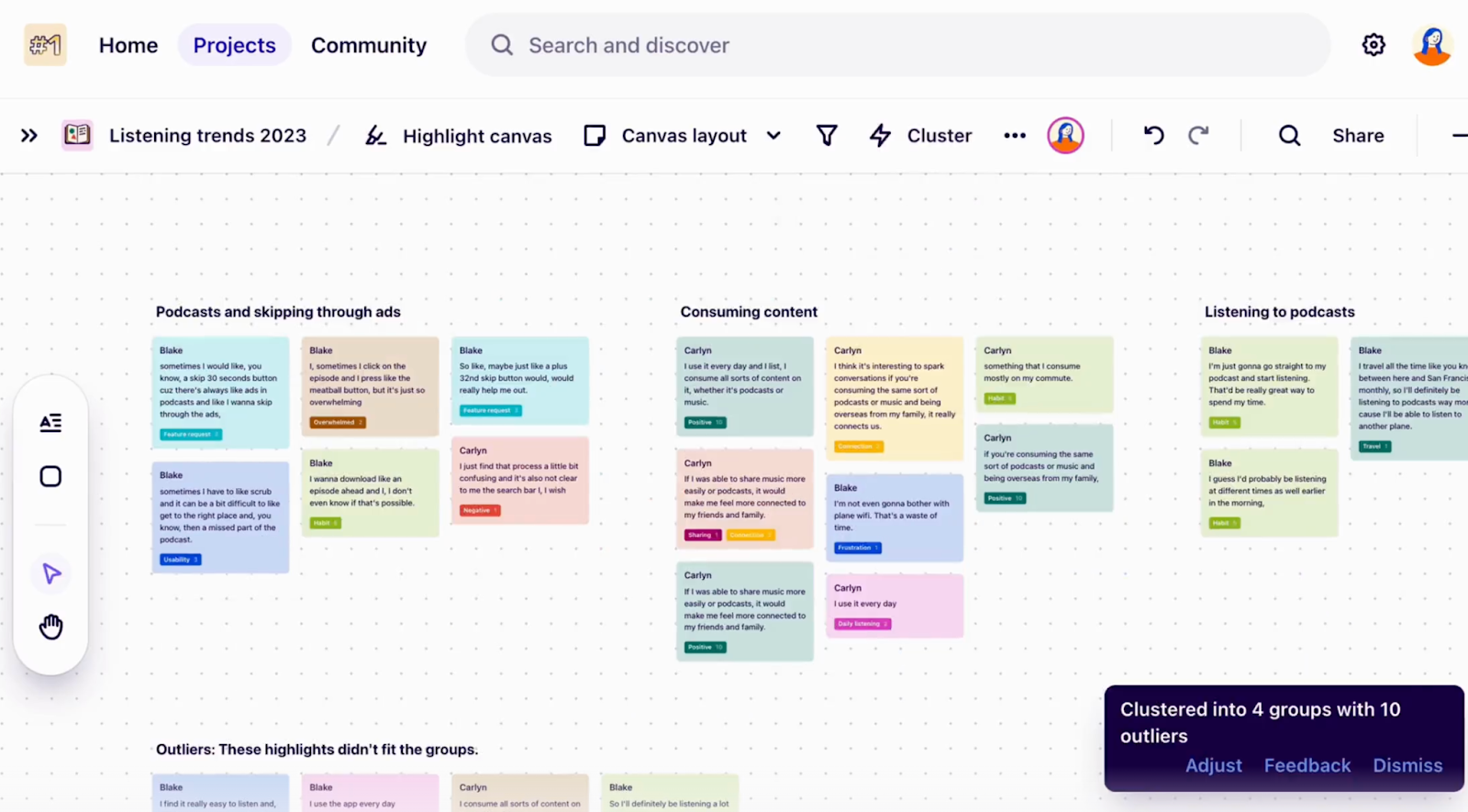
Dovetail is another research repository and analysis platform. While Dovetail still relies on manual tagging, it offers several powerful content analysis features like:
- Auto-transcription: It turns your video calls into text.
- Flexible views: See your data as a board, table, cluster, list, or grid.
- Video summaries: Get quick, timestamped summaries of your calls.
- Smart search: Ask questions and get summarized answers from your data.
- Multi-channel analysis: Track themes across reviews, support tickets, and more.
Dovetail users also appreciate the ability to directly tag video transcripts and quickly find and replay key moments from study sessions.
4. Notably
Pricing: Pro plan starts at $25 monthly
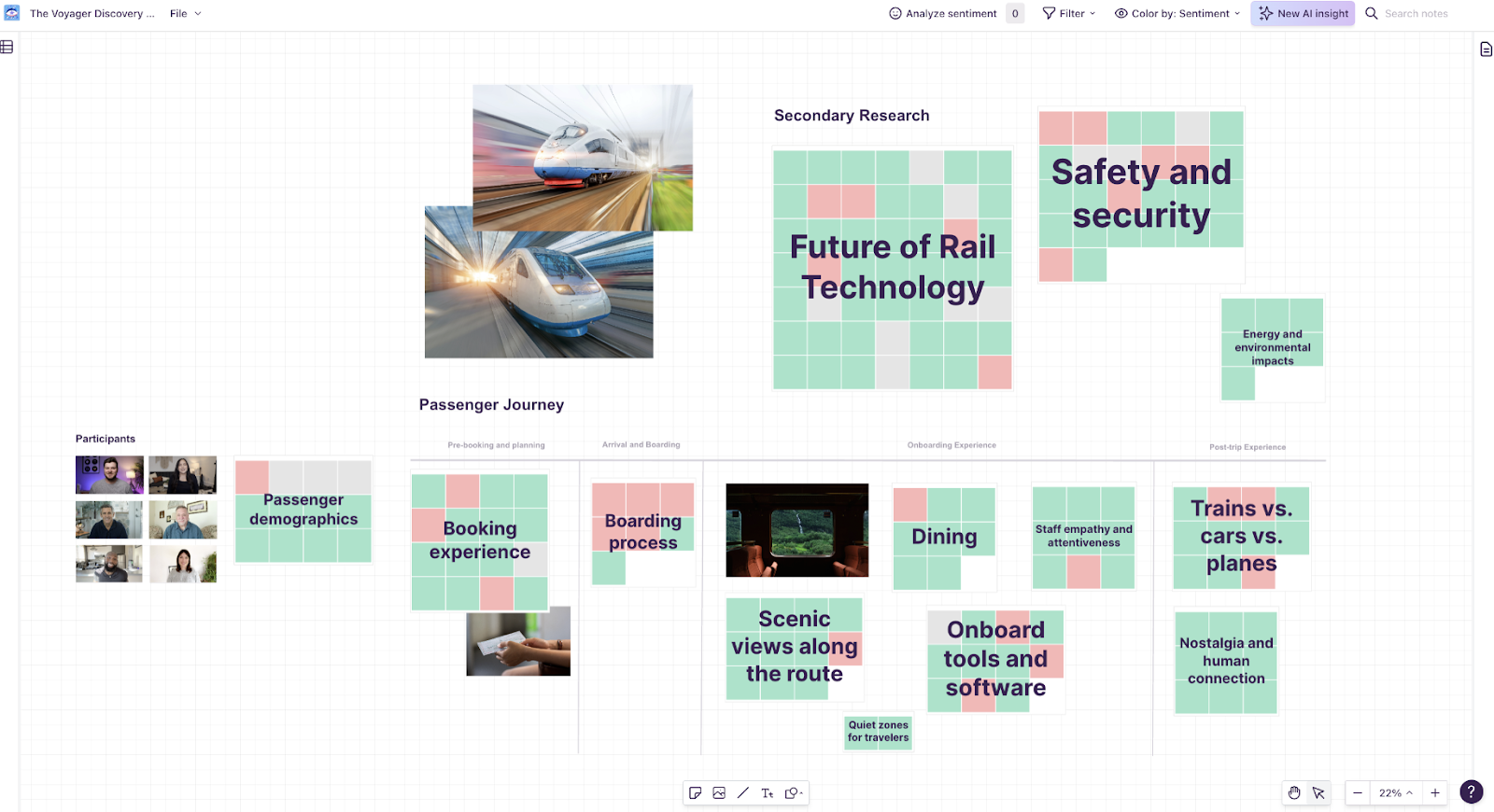
Notably is a research analysis and note-taker software for researchers working with qualitative data like interviews, surveys, and notes. It was originally built as a note-taking tool for students and professionals, and has since expanded to offer more features like transcription of audio recordings, AI summary templates for sharing research insights,and tagging.
Notably offers a range of AI-powered features for content analysis including:
- Video transcription, turning recordings into transcripts (up to 5 hours free)
- Transcript summaries, extract important parts of conversations into organized notes and takeaways
- Suggested AI-powered tags. AI learns and improves tag suggestions the more you use them
- Sentiment analysis across highlights from an entire study
- Generative Images from insight content or text input
- Custom AI templates for summaries and reports to stakeholders
Notably is great for researchers working with qualitative data like interviews and surveys.
5. MAXQDA
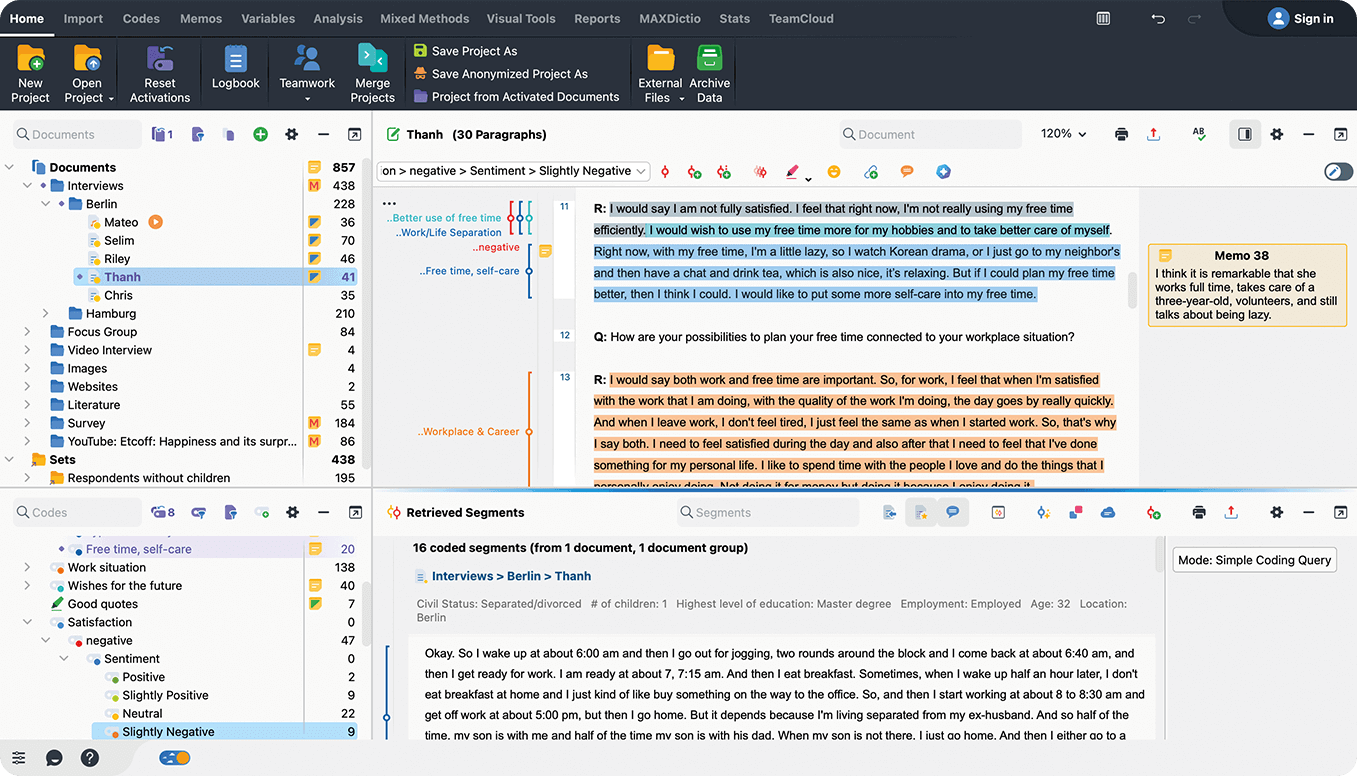
MAXQDA is the heavyweight champion of qualitative and mixed methods research. It's more complex than the others, but is ideal for academics and scientists who need deep, detailed analysis.
Here are some of the things MAXQDA can do:
- AI Assist: Chat with your data, get summaries, and even definitions of tricky terms.
- Quantitative analysis: Dive into word frequencies and dictionary-based analysis.
- Visual mapping: Create concept maps to show relationships in your data.
- Comprehensive workspace: Organize your thoughts, data, and conclusions in one place.
The Future of AI in Content Analysis
Let's explore the exciting developments on the horizon and their potential impact on various industries.
Evolving Job Landscape
AI's growing capabilities in content analysis will reshape the job market. Routine tasks like data cleaning and basic categorization will likely become automated. This might lead to a dip in traditional content analyst roles. But don't worry - it's not all bad news.
New opportunities will emerge. We'll need more people to develop and fine-tune AI algorithms. Jobs focusing on interpreting AI insights and applying them to business strategies will grow. The key will be adapting our skills to work alongside AI, not compete with it.
Smarter Language Understanding
Current AI can analyze text, but it often misses subtle nuances that humans easily grasp. The future promises AI with improved natural language understanding. These systems will pick up on context, tone, and even cultural references more like we do.
Imagine an AI that can detect sarcasm in customer reviews or understand regional slang in social media posts. This level of comprehension will lead to more accurate sentiment analysis and richer insights from text data.
Multi-Modal Magic
One of the most exciting trends is multi-modal or cross-modal analysis. Instead of looking at text, images, audio, and video separately, future AI will analyze them together. This holistic approach will give us a more complete picture of the content we're studying.
For example, when analyzing a video ad, the AI might consider:
- The script (text)
- Visual elements and brand colors (image)
- Background music and voice tone (audio)
- Audience reactions (video)
By combining insights from all these elements, we'll get a deeper understanding of the ad's effectiveness and impact.
Peeking Inside the Black Box
Many current AI systems are "black boxes" - we input data and get results, but we don't always know how the AI reached its conclusions. This lack of transparency can be a problem, especially in fields like healthcare or finance where decisions need to be explainable.
The future of AI content analysis will likely include more "explainable AI" models. These systems will provide clearer reasoning for their conclusions. This transparency will build trust and allow us to better validate and refine AI insights.
Preparing for the Future
So, how can we prepare for this AI-powered future? Here are some tips:
- Stay curious: Keep learning about AI developments. You don't need to become a programmer, but understanding the basics will help you work effectively with AI tools.
- Develop complementary skills: Focus on skills that AI can't easily replicate, like creative problem-solving, strategic thinking, and emotional intelligence.
- Embrace ethical AI: As you use or develop AI systems, always consider the ethical implications. Strive for fairness, transparency, and respect for privacy.
Experiment: Don't be afraid to try new AI tools as they emerge. Hands-on experience will help you understand their potential and limitations.