Ever wondered how researchers make sense of hundreds of interview transcripts, feedback forms, or survey responses? Cue content analysis—-a powerful method that helps researchers uncover patterns and meaning in qualitative data. In this guide, we'll break down what content analysis is, how it works, and when to use it.
What is qualitative content analysis?
Qualitative content analysis is a research method that helps find patterns and themes in text data. Think of it as a systematic way to organize and understand large amounts of written information - whether that's interview transcripts, open-ended survey responses, or documents.
For example, if you're analyzing customer feedback about a new product feature, content analysis helps you identify common themes in user comments, track how often certain issues come up, and understand the context behind user reactions.
What is the primary goal of content analysis in qualitative research?
The main goal of content analysis in qualitative research is to turn raw text data into meaningful insights. It helps researchers:
- Find patterns in data
- Understand context and meaning
- Draw reliable conclusions
- Support findings with evidence
When to use content analysis
Content analysis works best when you need to:
- Make sense of large amounts of text data
- Find patterns across multiple documents
- Support findings with specific evidence
- Track changes over time
- Compare different sources of information
What is qualitative content analysis example?
Understanding qualitative content analysis becomes clearer through examples.
In the product development world, UX researchers often analyze customer feedback from multiple channels - app reviews, support tickets, and user interviews. Let’s imagine—a research team at a food delivery app uses content analysis to understand why users were abandoning their orders. They examine thousands of customer comments, coding them for themes like "pricing concerns," "delivery timing," and "app usability." This systematic analysis reveal that confusing delivery fee calculations were the primary pain point, leading to specific app improvements.
In healthcare research, content analysis can help improve patient care. For instance, imagine that a hospital analyzed patient feedback forms to enhance their emergency room experience. Researchers coded written comments for themes like "wait time," "staff communication," and "facility comfort." They could discover that while wait times were a common complaint, poor communication about expected wait duration caused more frustration than the wait itself. This insight can lead to implementing real-time wait updates via text messages.
Social media researchers provide another compelling use case for qualitative content analysis. A retail brand for instance, analyzes customer comments across their social media platforms during a rebranding campaign. They code posts for sentiments, specific feedback about logo changes, and commentary about brand values. This analysis might reveal that while customers disliked the new logo colors, they strongly supported the brand's shift toward sustainability, leading to a modified design that better balanced both aspects.
What are the three types of qualitative content analysis?
Conventional content analysis
Conventional content analysis works best when researchers want to describe a phenomenon without preexisting theories. Researchers read through the data multiple times, allowing categories to emerge naturally. This approach works well for exploratory research where little is known about the topic.
Directed content analysis
Directed content analysis starts with existing theories or research findings. Researchers use these as a guide for initial coding, but remain open to new categories and themes. This method helps validate or extend theoretical frameworks while maintaining flexibility.
Summative content analysis
Summative content analysis begins by identifying and counting specific words or content, then expands to include interpretation of the underlying context. This approach helps researchers understand both the frequency and meaning of certain terms or themes in their data.
Thematic Analysis vs. Qualitative Content Analysis vs. Discourse Analysis
What is content and discourse analysis in qualitative research?
While content analysis focuses on systematic categorization and interpretation of text data, thematic analysis takes a more flexible approach to finding patterns. Thematic analysis allows for more interpretive freedom but might sacrifice some of the systematic rigor of content analysis. Discourse analysis examines how language constructs social reality.
What is an example of context and discourse analysis?
A content and discourse analysis qualitative research example might look at how company emails reflect and shape organizational culture. While content analysis focuses on what's being said, discourse analysis explores how language choices reflect power relationships and social contexts.
How do you write a content analysis in qualitative research?
Planning your analysis
The foundation of good content analysis starts with thorough planning. Begin by clearly defining your research questions and what you want to learn from your data. If you're studying customer feedback about a new product feature, your question might be "What aspects of the feature are causing user friction?" This guides every subsequent decision in your analysis process.
Preparing your data
Data preparation involves more than just collecting materials. You need to organize your data in a way that makes analysis manageable. This means transcribing interviews accurately, cleaning up messy text data, and ensuring all materials are in a consistent format. Create a clear system for naming and storing files to avoid confusion later.
Creating your coding framework
Developing a coding framework is like creating a map for your analysis journey. Start by reading through a sample of your data to get familiar with its content. Note recurring themes or patterns that emerge. These initial observations help you create preliminary codes that capture important aspects of your data. Your framework should be flexible enough to evolve as you discover new patterns.
The coding process
The actual coding process requires patience and attention to detail. Read through your data line by line, applying codes that capture the meaning of each segment. You might start with broad categories and then create subcategories as you notice finer distinctions. Keep detailed notes about why you assigned specific codes - these notes become valuable when interpreting your findings later.
Ensuring reliability
Reliability in coding doesn't happen by accident. Regularly check your coding consistency by recoding some materials after a few days and comparing results. If you're working with a team, have multiple people code the same content and discuss any differences. This process, called intercoder reliability checking, helps ensure your analysis remains consistent and trustworthy.
Finding patterns and relationships
Once your initial coding is complete, look for patterns and relationships in your coded data. What themes appear most frequently? How do different codes relate to each other? Create visual maps or diagrams to help you see these relationships more clearly. This step transforms your coded data into meaningful insights.
Writing up your findings
Writing up your analysis requires clear organization and plenty of supporting evidence. Start with your most significant findings and use specific examples from your data to support each point. Explain not just what you found, but why it matters and how it connects to your research questions. Include enough detail about your process so others can understand how you reached your conclusions.
Reflecting and revising
The final step involves stepping back and reflecting on your analysis. Are there alternative explanations for your findings? Have you considered all relevant contexts? This reflection often leads to valuable refinements in your analysis. Remember that content analysis is often iterative - you might need to revisit earlier steps as new insights emerge.
The advantages and disadvantages of content analysis
Advantages:
- Flexible and adaptable
- Can handle large amounts of data
- Provides systematic approach
- Generates reliable insights
- Supports both quantitative and qualitative analysis
Disadvantages:
- Time-consuming
- Can be subjective
- Requires careful planning
- May miss context
- Needs skilled coders
How Looppanel’s AI can help with content analysis in qualitative research
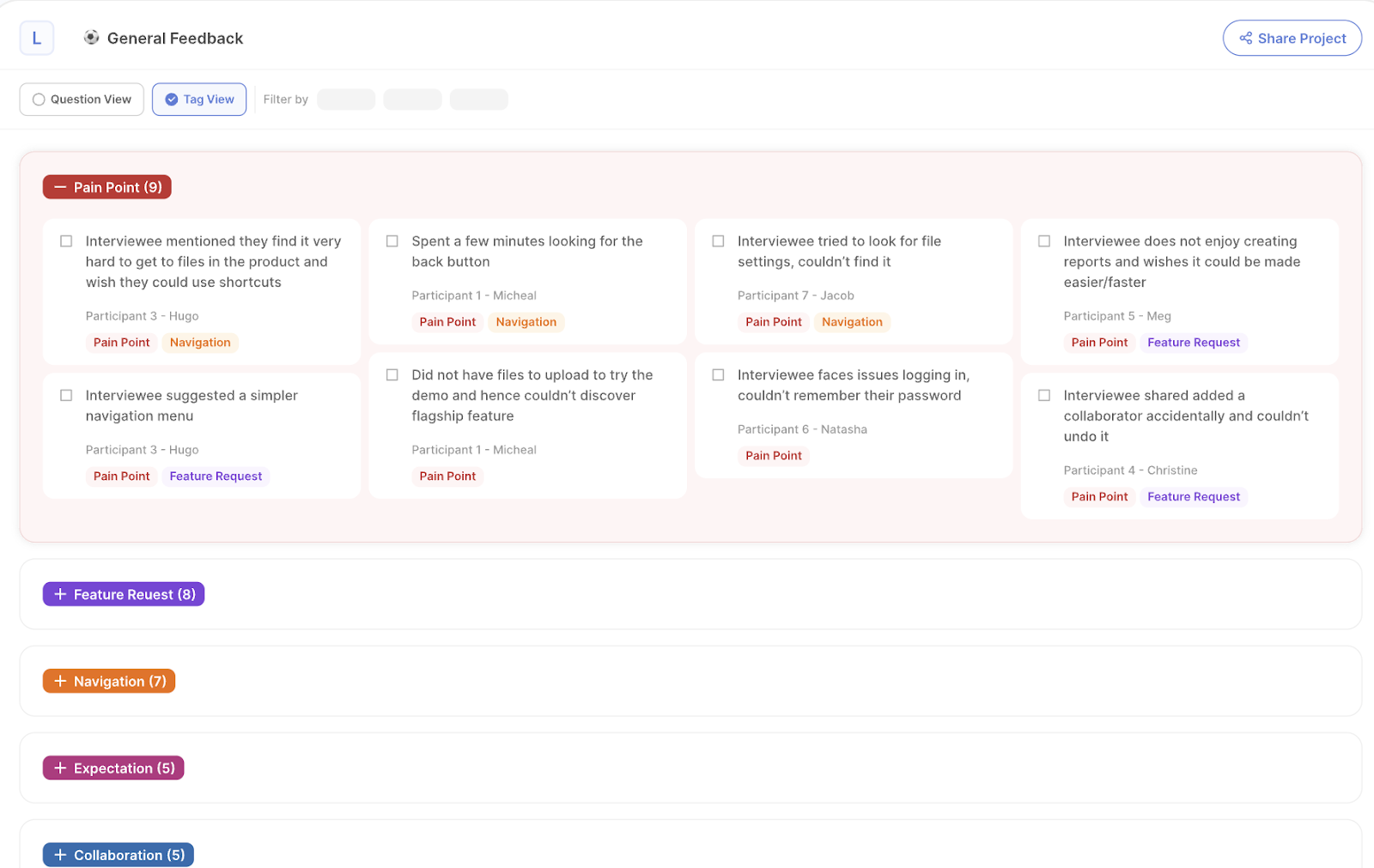
Content analysis has traditionally been a time-intensive process, often taking researchers weeks to process interview transcripts and find meaningful patterns. But AI tools are changing the game, and Looppanel is one of the best among them (in our completely unbiased opinion).
Looppanel is a UX research analysis & repository solution for the modern UX team. Looppanel helps you analyze research data 10x faster, centralize feedback in one searchable hub, and surface insights in seconds.
Think of it as having a super-smart research assistant who handles the tedious parts of analysis, letting you focus on finding deeper meanings and connections. The AI spots initial patterns, but you bring your expertise to interpret what those patterns mean for your product or service.
Curious to know more? Try it out here.
Frequently Asked Questions (FAQs)
What is content analysis designed to do in qualitative research methods?
Content analysis in qualitative research is designed to systematically uncover meaningful patterns in text data. Think of it as a structured way to find the story hidden in mountains of qualitative data. Researchers use this method to identify themes, track sentiment changes, and understand relationships between different aspects of their data. As a qualitative content analysis example, imagine analyzing customer service chat logs to understand not just what problems users report, but how those problems affect their overall experience with the product. The method helps researchers move beyond counting occurrences to understanding the deeper meaning and context behind communications.
What is an example of a content analysis?
Let's look at several real-world applications. A content analysis in qualitative research example might involve studying how teachers give feedback on student essays. Researchers might analyze written comments to understand patterns in how teachers communicate areas for improvement. Another example of content analysis in qualitative research could be analyzing company internal communications during a major organizational change. A content and discourse analysis qualitative research example might examine both what information is shared and how language choices reflect changing power dynamics. In healthcare, a content analysis example qualitative research might involve studying patient discharge instructions to understand how medical information is communicated and what aspects might cause confusion for patients. Each of these examples shows how content analysis helps researchers understand not just what is being said, but its broader meaning and implications.