User research analysis is the heart of understanding your users. Think of it as detective work – you're looking for clues in what users say, do, and think to solve the mystery of their needs and behaviors.
What is user research analysis?
In user research analysis, researchers take all the information they’ve gathered – from interviews, surveys, usability tests, and more – and make sense of it. It’s not just summarizing what we heard or saw. We're digging deeper to find patterns, understand the reasons behind user actions, and uncover opportunities for improvement.
UX research analysis is also where we connect the dots between user behavior and product design, helping teams make informed decisions that truly meet user needs.
Why should researchers spend time on analysis?
Spending time on user research and analysis is like investing in a map before going on a journey. Sure, you could set off without one, but you're much more likely to reach your destination efficiently with a good map in hand.
Here's why analysis is so important.
- Uncover hidden patterns: Users often don't explicitly state their deepest needs or frustrations. Good analysis helps you spot trends that might not be immediately obvious.
- Validate or challenge assumptions: We all have ideas about how users think or behave. Analysis lets you test these assumptions against real data, often leading to surprising discoveries.
- Prioritize effectively: With limited time and resources, you need to know where to focus. Analysis helps you identify which user needs or pain points are most critical to address.
- Justify decisions: When you need to convince stakeholders to make changes, having solid, analyzed data to back up your recommendations is invaluable.
- Guide innovation: Sometimes, the gaps and frustrations you uncover through analysis can spark ideas for completely new features or products.
- Measure progress: By analyzing user research over time, you can track how changes to your product impact user satisfaction and behavior.
Remember, data without analysis is just noise. It's the careful examination and interpretation of that data that turns it into a powerful tool for improving user experience.
When should you do analysis in UX Research?
Before the research begins
It might seem odd to think about analysis before you've even started your research, but this is a crucial step that many researchers overlook. Here's what you should consider:
- Define your objectives: What exactly do you want to learn? Having clear goals will guide your analysis later.
- Choose your methods wisely: Different research methods will yield different types of data. Think about how you'll analyze the data when selecting your methods.
- Create an analysis plan: Outline how you'll organize and code your data. This forethought can save you a lot of time and confusion later.
- Prepare your tools: Whether you're using specialized software or just spreadsheets, set up your analysis framework in advance.
- Consider your team: If you're working with others, decide how you'll collaborate on the analysis process.
During the research
Analysis isn't just an end-stage activity. Ongoing analysis during your research can be incredibly valuable for the following reasons.
- Spot emerging patterns: As you collect data, you might notice themes starting to appear. Make note of these – they could guide further inquiry.
- Adjust your approach: If you're not getting the insights you need, ongoing analysis can help you tweak your research questions or methods mid-stream.
- Capture fresh insights: Sometimes, the most valuable insights come in the moment. Don't wait to start interpreting what you're seeing and hearing.
- Manage your data: Start organizing and tagging your data as you go. This will make your final analysis much smoother.
When the Research Is Done
This is typically the most intensive phase of analysis in UX research. Here’s how analysis plays a role in it.
- Code and categorize: Systematically tag and group your data to make patterns easier to spot.
- Look for patterns and themes: What common threads run through your data? What stands out as unique or unexpected?
- Generate insights: Move beyond just describing what you found to interpreting what it means for your users and your product.
- Test your conclusions: Challenge your own interpretations. Look for data that contradicts your findings as well as supports them.
- Create deliverables: Translate your analysis into reports, presentations, or other formats that will effectively communicate your insights to stakeholders.
Remember, good user research analysis isn't a one-time event. It's an ongoing process that spans the entire research journey, helping to ensure that the insights you generate are robust, meaningful, and actionable.
What are the methods of user analysis?
Qualitative vs. quantitative analysis
In the world of user research analysis, we have two main approaches: qualitative and quantitative. Each has its strengths, and often, the most powerful insights come from combining both.
Qualitative analysis
Qualitative analysis deals with non-numerical data – the stories, opinions, and experiences of your users. It's all about understanding the 'why' behind user behavior.
Methods include:
- Thematic analysis: Identifying patterns or themes in your data.
- Content analysis: Systematically categorizing and interpreting text data.
- Grounded theory: Developing theories based on the data you collect.
- Narrative analysis: Examining the stories users tell about their experiences.
Quantitative analysis
Quantitative analysis deals with numerical data. It answers questions like 'how many', 'how often', or 'how much'.
Methods include:
- Descriptive statistics: Summarizing data with measures like averages and percentages.
- Inferential statistics: Drawing conclusions about a population based on a sample.
- Regression analysis: Examining relationships between variables.
- Cluster analysis: Grouping users based on similar characteristics.
In practice, most user research uses a mix of both qualitative and quantitative analysis. For example, you might use quantitative analysis to identify a trend in user behavior, then use qualitative analysis to understand the reasons behind that trend.
The key is to choose the right type of analysis for your research questions and to be aware of the strengths and limitations of each approach. By combining both, you can get a more complete picture of your users and their needs.
How to do user research analysis
Good user research analysis is both a science and an art. It requires systematic thinking and attention to detail, but also creativity and intuition. Let's dive into how to do it effectively.
Steps for analyzing research once it's done
- Organize your data: Start by getting all your data in one place. This might mean transcribing interviews, collating survey responses, or gathering usability test results. Create a system for naming and storing files that makes sense to you and your team.
- Read through everything: Before you start formal analysis, immerse yourself in the data. Read through everything at least once, maybe twice. This helps you get a feel for the overall picture and start noticing patterns.
- Code your data: Coding is the process of tagging important points or themes in your data. For example, if you're researching a food delivery app, you might use codes like "ordering process," "delivery experience," or "menu navigation." Be consistent with your codes and keep a codebook to track what each code means.
- Look for patterns: Now that your data is coded, start looking for patterns. What themes keep coming up? Are there common pain points or desires across multiple users? Are there any surprising findings that challenge your assumptions?
- Create personas or user journeys: Based on your patterns, you might create personas (fictional characters that represent user types) or user journeys (maps of the user's experience with your product). These help visualize your findings and make them more relatable to stakeholders.
- Check your biases: It's easy to see what you want to see in the data. Actively look for information that contradicts your expectations or hypotheses. Consider having team members review each other's analysis to catch potential biases.
- Quantify qualitative data: Even with qualitative data, it can be helpful to add some numbers. For example, "7 out of 10 users struggled with the checkout process" is more impactful than "Some users had trouble checking out."
- Synthesize your findings: Move beyond just describing what you found to interpreting what it means. What are the implications of your findings for your product or users?
- Prioritize your insights: Not all findings are equally important. Prioritize your insights based on factors like user impact, business goals, and feasibility of addressing the issue.
- Get a second opinion: Have someone else look at your data and conclusions. Fresh eyes can spot patterns you might have missed or challenge your interpretations.
Sharing key insights from user research
Once you've completed your analysis, it's time to share your insights with your team and stakeholders. This is a crucial step – even the best analysis is useless if it doesn't lead to action.
Findings vs Insights
It's important to understand the difference between findings and insights:
- A finding is a fact from your research. It's objective and directly observable from your data.
- An insight is what that fact means for your product or users. It requires interpretation and often points to opportunities or problems to solve.
For example:
- Finding: 70% of users click the 'Help' button within 5 minutes of using the app.
- Insight: Users find the app confusing and need more guidance early in their experience. We should focus on improving the app's intuitiveness and providing proactive help.
When presenting your research, include both findings and insights, but focus on the insights – they're what will drive action.
Next steps for user research analysis
Analysis doesn't end with the presentation of insights. Here's what typically comes next:
- Brainstorm solutions: Work with your design and product teams to generate ideas based on your insights. How can you address the user needs and pain points you've uncovered?
- Prioritize ideas: Not every insight can be acted on immediately. Work with stakeholders to decide which areas to focus on first, based on factors like user impact, business goals, and resource constraints.
- Create a roadmap: Develop a plan for implementing changes based on your research. This might involve design sprints, prototyping, or further research.
- Plan follow-up research: Think about what questions remain unanswered or what new questions your analysis has raised. Plan follow-up studies to dig deeper or to test potential solutions.
- Measure impact: Once changes are implemented based on your insights, plan how you'll measure their impact. This closes the loop and demonstrates the value of user research.
Remember, each round of research builds on the last, deepening your understanding of your users and continually improving your product.
What is an example of user research analysis?
Let's walk through a detailed example of user research analysis for a food delivery app. This will help illustrate how the process works in practice.
Research Scenario: You've conducted 20 in-depth interviews with users of your food delivery app, along with analyzing app usage data for 1000 users over the past month.
Step 1: Organize Data
- Transcribe all 20 interviews
- Collate app usage data into spreadsheets
- Create a central repository for all research materials
Step 2: Initial Review
Read through all transcripts and review the usage data to get a general sense of the information. You notice several users mentioning issues with delivery times and the app's search function.
Step 3: Coding
Develop a coding scheme and apply it to your data. Some codes might include:
- ORDER: References to the ordering process
- DELIV: Comments about delivery experience
- SRCH: Mentions of the search function
- UX: General user experience comments
- CUST: Customer service interactions
Step 4: Pattern Identification
After coding, you start to see clear patterns:
- 15 out of 20 interviewees mentioned frustration with delivery times
- Usage data shows that 40% of users abandon their order after using the search function
- 12 interviewees praised the variety of restaurant options
Step 5: Deeper Analysis
Digging deeper into the delivery time issue, you find:
- Users in certain zip codes consistently report longer wait times
- These areas have fewer restaurant partners
- Usage data shows a 30% drop in repeat orders from these areas
Step 6: Insight Generation
Based on this analysis, you form several key insights:
- Delivery time issues in specific areas are significantly impacting user satisfaction and retention.
- The app's search function is causing friction in the user journey, leading to lost sales.
- The wide variety of restaurant options is a key strength that could be leveraged more.
Step 7: Recommendations
For each insight, you develop actionable recommendations:
- Expand restaurant partnerships in underserved areas to reduce delivery times.
- Conduct usability testing on the search function to identify and fix issues.
- Highlight restaurant variety in marketing materials and consider adding a "surprise me" feature to help users discover new options.
Step 8: Presentation
You create a presentation for stakeholders that includes:
- Key findings supported by data (e.g., "40% of users abandon orders after searching")
- Insights derived from these findings
- Recommendations for next steps
- Visualizations like heat maps of problematic zip codes and user journey maps highlighting pain points
Step 9: Follow-up Research Plan
Based on the stakeholder discussion, you plan follow-up research:
- Usability testing focused on the search function
- Interviews with users in underserved areas to understand their needs better
- A survey to all users about potential new features like "surprise me"
Tools and software for research data analysis
In the world of user research analysis, having the right tools can make a big difference. Here are some popular options, along with their pricing and key features.
Looppanel
Looppanel is an AI-driven research assistant designed to take over the repetitive parts of research tasks.
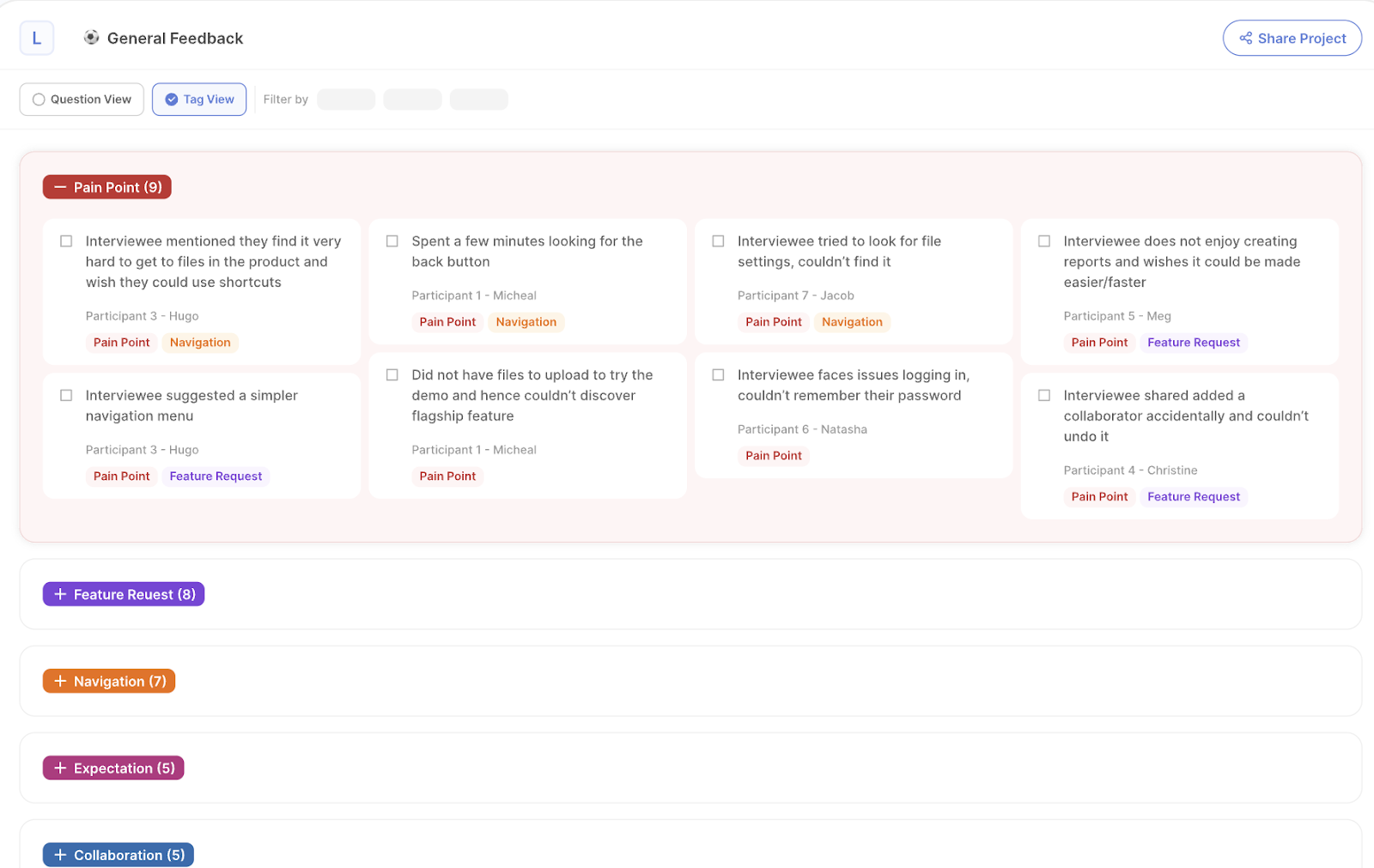
Key features include:
- Fast, accurate transcripts in multiple languages (over 90% accuracy).
- Sentiment analysis with color coding for positive, negative, and neutral responses.
- Auto-generated, human-like notes organized by interview questions.
- Automatic tagging of data into common themes and patterns.
- Powerful search across your workspace to quickly find any snippet or quote.
Looppanel organizes notes by matching them to specific interview questions. For example, if you ask, "Tell me about your role," it will sort relevant responses under that question.
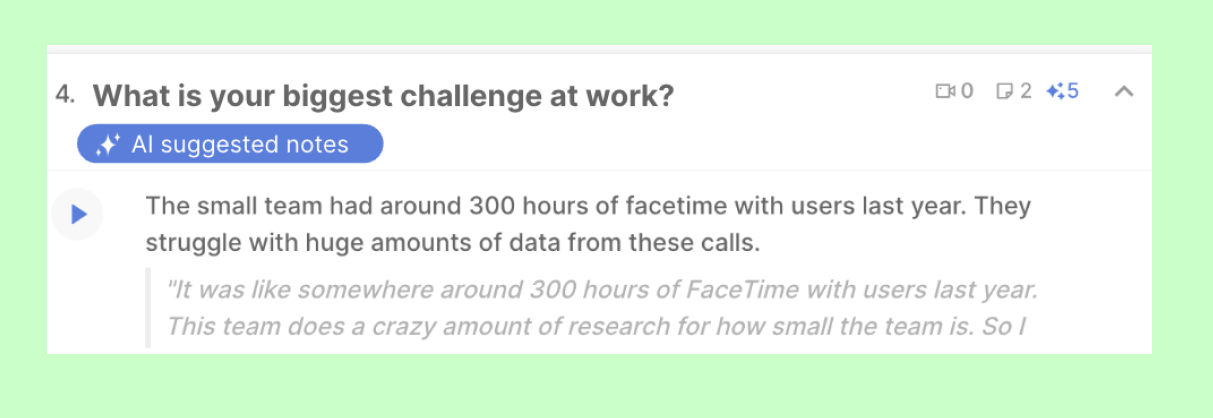
The ‘Analysis’ tab allows you to view notes across all calls, with AI suggesting tags for easier organization. You can also tag data manually, and Looppanel helps by creating affinity maps.
It’s simple to use—just upload your calls and discussion guide, and Looppanel does the rest.
Pricing: Starts at $30/month, with a free trial.
Marvin
Marvin is a research repository and analysis tool built for researchers.
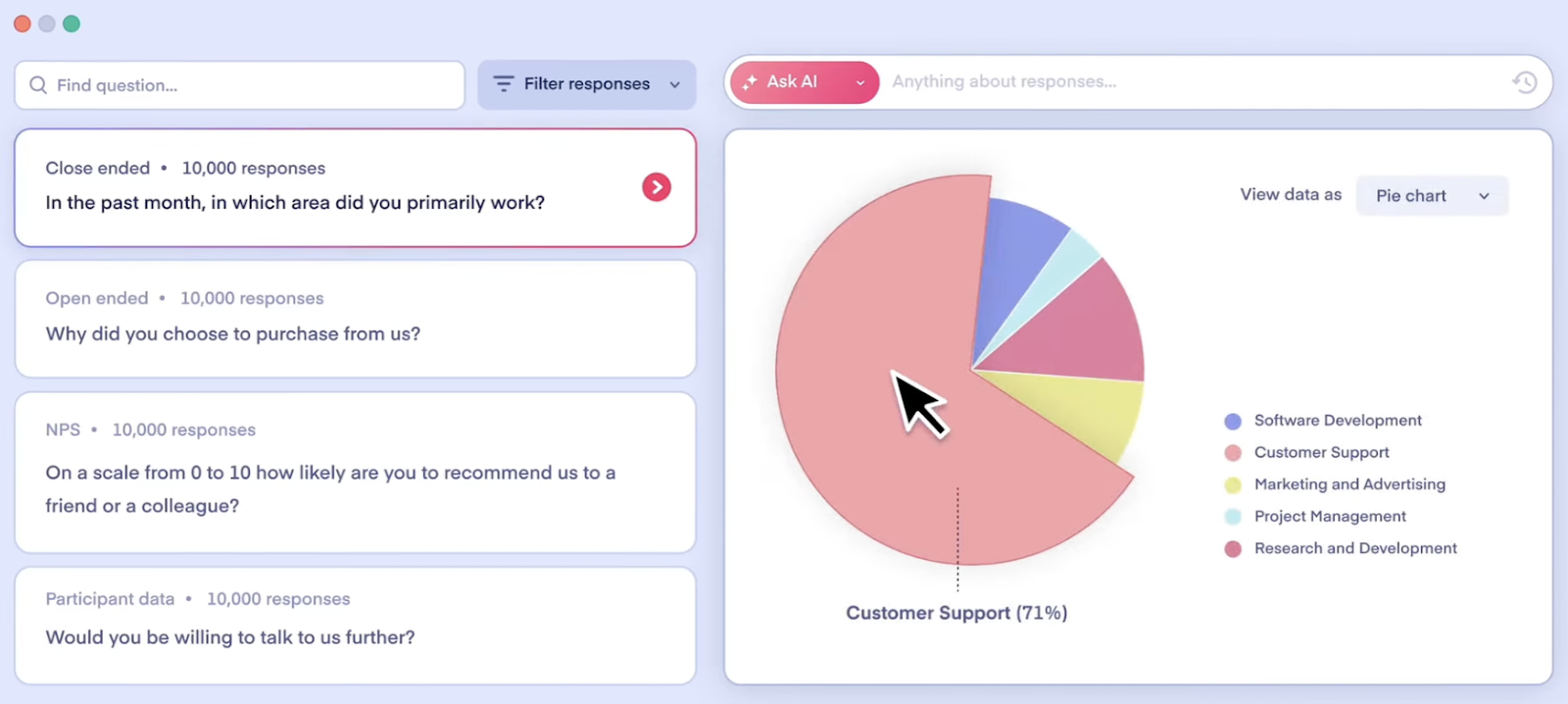
Marvin offers:
- Thematic analysis to automatically group key themes from qualitative data.
- Sentiment analysis to understand user emotions.
- Pattern recognition to identify trends across notes.
- Quick, AI-powered search to find quotes or answers from the entire research repository.
The "Ask AI" feature stands out, enabling easy searching, summarizing, and pattern identification across surveys and interviews using natural language. It also helps teams access insights from various projects.
Though survey uploads require manual input, Marvin provides robust analysis and visualization tools like charts and graphs to present findings.
Pricing: Free plan with limited features, paid plans start at $50/user/month.
Dovetail
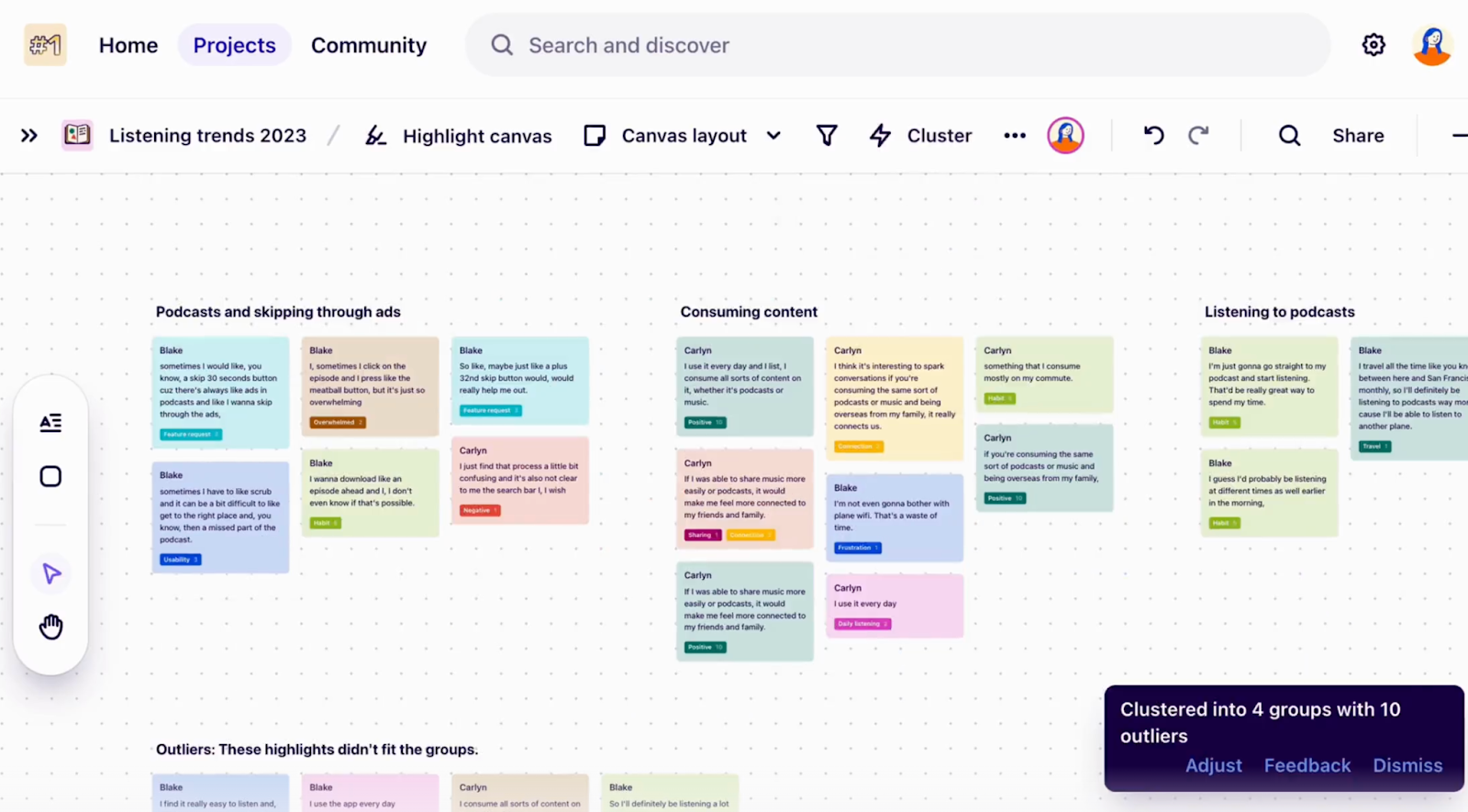
Dovetail is a research repository and analysis tool that still relies on manual tagging but comes with strong analysis capabilities:
- Transcription of user interviews.
- Multiple data views, such as Board, Cluster, and List.
- Instant, timestamped summaries of video calls.
- Magic search to find topics or get summarized answers.
- Automatic key moment suggestions in video calls.
- Multi-channel analysis for tracking themes in feedback, reviews, and tickets.
Users appreciate the ability to tag video transcripts and locate key moments instantly.
Pricing: Free plan with limited features, paid plans from $29/user/month (annual billing).
Condens Condens is considered a lighter version of Dovetail, offering a simpler and more intuitive interface but fewer advanced features.

AI-powered features in Condens include:
- AI-assisted tagging.
- Automatic bookmarks for key topics in long transcripts.
- Quick summaries of key highlights.
- AI-suggested tags to reveal connections and prevent duplicates.
- Transcription in 85+ languages, with timestamps and speaker recognition.
Condens helps manage large amounts of data and ensures no important insights are missed.
Pricing: 15-day free trial, individual plans start at $15/month.
When choosing a tool for user research analysis, consider factors like:
- The size of your team and how you collaborate
- The types of research you conduct most often
- Your budget
- Integration with your existing tools and workflows
- The learning curve and available support
Remember, while these tools can greatly enhance your analysis process, they're not a replacement for critical thinking and interpretation. The best insights often come from combining tool capabilities with human expertise in user research analysis.
Challenges and common mistakes in analyzing data
User research analysis, while invaluable, comes with its own set of challenges. Being aware of these can help you navigate the process more effectively and avoid common pitfalls.
The problems of bias, subjectivity and objectivity
One of the biggest challenges in user research analysis is maintaining objectivity. It's all too easy to see what we want to see in the data, a phenomenon known as confirmation bias. Here's how this and other challenges can manifest:
Confirmation Bias
This is the tendency to focus on information that confirms our preexisting beliefs. For example, if you believe users struggle with your app's navigation, you might pay more attention to comments that support this view while downplaying contradictory feedback. To combat this:
- Actively look for evidence that contradicts your hypotheses
- Have team members review each other's analysis
- Use structured analysis methods that force you to consider all data equally
Selection Bias
This occurs when your sample doesn't accurately represent your user base. For instance, if you only interview power users, you might miss the struggles of newcomers. To avoid this:
- Ensure your research participants represent a diverse range of users
- Be clear about the limitations of your sample when reporting findings
- Use multiple research methods to get a more complete picture
Interpretation Bias
This happens when we impose our own meanings onto user statements or behaviors, rather than truly understanding the user's perspective. To mitigate this:
- Use direct quotes from users to support your interpretations
- Practice reflective listening during interviews to check your understanding
- Consider alternative explanations for user behavior
Recency Bias
This is the tendency to place more importance on the most recent data or experiences. To counter this:
- Systematically review all your data, not just the most recent
- Use tools to help organize and weigh all data equally
- Conduct longitudinal studies to track changes over time
Quantifying Qualitative Data
While it can be tempting to turn qualitative data into numbers (e.g., "80% of users said..."), this can be misleading with small sample sizes. Instead:
- Use phrases like "most users" or "a few users" for qualitative data
- Only use percentages when you have a statistically significant sample size
- Combine qualitative insights with quantitative data when possible
Overconfidence in Insights
Sometimes, researchers might present tentative findings as definitive insights. To avoid this:
- Be clear about the strength of evidence behind each insight
- Indicate where further research might be needed
- Be open to revising insights as new data comes in
Remember, some subjectivity in user research analysis is unavoidable – after all, we're interpreting human behavior and experiences. The key is to be aware of our biases, use methods to minimize their impact, and be transparent about the limitations of our analysis.
Ways and methods for good analysis
To conduct effective user research analysis and overcome the challenges mentioned above, consider these methods and best practices.
Triangulation
Use multiple methods or data sources to verify your findings. For example, combine interview data with usability test results and analytics data.
This approach helps overcome the limitations of any single method and provides a more comprehensive view of user behavior and needs.
Peer debriefing
Regularly discuss your analysis process and findings with colleagues. This can be formal (like scheduled review sessions) or informal (quick chats about interesting findings).
Other perspectives can help spot biases, generate new insights, and ensure the analysis is on the right track.
Member checking
Share your interpretations with research participants to see if they resonate. This doesn't mean participants have the final say, but their feedback can be valuable.
This helps ensure you're accurately representing user perspectives and can uncover misinterpretations early.
Negative case analysis
Actively seek out data that doesn't fit your emerging patterns or contradicts your hypotheses. This helps guard against confirmation bias and can lead to more nuanced, accurate insights.
Audit trail
Keep detailed records of your analysis process, including your coding scheme, how you arrived at your insights, and any major decisions made during analysis.
This promotes transparency, allows others to review your process, and helps you reflect on and improve your methods over time.
Iterative analysis
Don't wait until all data is collected to start analyzing. Begin analysis early and refine your approach as you go.
This allows you to adjust your research questions or methods if needed and can help manage large amounts of data.
Collaborative analysis
Involve team members in the analysis process, perhaps through group coding sessions or insight generation workshops. Multiple perspectives can lead to richer insights and help overcome individual biases.
Quantitative + Qualitative
Whenever possible, combine quantitative data (like usage statistics) with qualitative insights (like user quotes). This provides a more complete picture, balancing the 'what' (quantitative) with the 'why' (qualitative).
Visual mapping
Use techniques like affinity diagramming or journey mapping to visualize patterns and relationships in your data. Visual representations can help spot patterns that might not be obvious in raw data and can be powerful tools for communicating insights.
Scenario-based analysis
Analyze your data in the context of specific user scenarios or tasks. This helps ensure your insights are grounded in real user needs and behaviors, rather than abstract generalizations.
By employing these methods, you can enhance the rigor and reliability of your user research analysis, leading to more actionable and impactful insights.
Frequently Asked Questions (FAQs)
Is user research the same as UX research?
User research and UX research are often used interchangeably, and they do have a lot of overlap. However, there can be subtle differences:
User research is a broad term that can apply to any type of research focused on understanding users, their needs, behaviors, and motivations. This could be for products, services, or even physical spaces. UX research specifically focuses on understanding user experiences with digital products or services. It's a subset of user research that's particularly concerned with how users interact with and feel about specific user interfaces and digital experiences.
In practice, many professionals use these terms interchangeably, especially in the context of digital product development. The core skills and methods are largely the same for both.
What is the difference between user research analysis and synthesis?
While these terms are closely related and often used together, they refer to different parts of the research process:
- Analysis is the process of breaking down data into its component parts to understand it better. It involves organizing, coding, and examining data to identify patterns and trends.
- Synthesis is the process of bringing different pieces of information together to form a coherent whole. It's about connecting the dots between different findings to generate insights and create a bigger picture understanding.
In user research, you typically analyze first (breaking down the data), then synthesize (bringing the pieces back together to form insights). Both are crucial for turning raw data into actionable insights.
What does a UX research analyst do?
A UX research analyst is responsible for planning, conducting, and analyzing user research to inform product design decisions. Their typical tasks include:
- Planning research studies: Defining research questions, choosing appropriate methods, and creating study plans.
- Conducting research: This might involve running usability tests, conducting interviews, creating and analyzing surveys, or performing contextual inquiries.
- Analyzing data: Using various methods to examine both qualitative and quantitative data to identify patterns and trends.
- Communicating findings: Creating reports, presentations, or other deliverables to share insights with stakeholders.
- Collaborating with design and product teams: Working closely with designers and product managers to ensure user insights inform product decisions.
What is the difference between user research and data analyst?
While there's some overlap, these roles have distinct focuses:
User Researcher:
- Focuses on understanding user behavior, needs, and motivations
- Often deals with qualitative data (interviews, usability tests)
- Generates insights to inform product design and strategy
- Typically has skills in research design, interviewing, and behavioral analysis
Data Analyst:
- Focuses on analyzing large sets of quantitative data
- Deals primarily with numerical data and statistics
- Identifies trends and patterns in user behavior at a larger scale
- Typically has skills in statistical analysis, data visualization, and often programming
In some organizations, these roles might collaborate. For example, a data analyst might provide insights from usage data that a user researcher then investigates further through qualitative methods.
How do you analyze data from user research?
Analyzing data from user research involves several steps:
- Organize your data: Transcribe interviews, collate survey responses, etc.
- Familiarize yourself with the data: Read through everything to get a general sense of the information.
- Code your data: Tag important points or themes in your data.
- Identify patterns: Look for common themes or trends across your data.
- Generate insights: Interpret what these patterns mean for your users and product.
- Validate your findings: Check your interpretations against the raw data and with team members.
- Prioritize insights: Determine which findings are most important or actionable.
- Create deliverables: Develop reports, presentations, or other ways to share your insights.
The specific methods you use will depend on whether you're dealing with qualitative data (like interview transcripts) or quantitative data (like survey responses or usage statistics).
How do you write a UX analysis?
Writing a UX analysis report typically involves the following steps:
- Start with an executive summary: Provide a brief overview of your key findings and recommendations.
- Introduce the research: Explain the goals of the research, the methods used, and who participated.
- Present your findings: Share what you discovered, organized by themes or user journeys. Use a mix of quantitative data, qualitative insights, and user quotes.
- Provide insights: Explain what your findings mean for the user experience and the product.
- Make recommendations: Based on your insights, suggest actionable next steps or areas for improvement.
- Include supporting evidence: Add relevant charts, user quotes, or journey maps to illustrate your points.
- Conclude with next steps: Suggest areas for further research or immediate actions to take.
Remember to tailor your report to your audience. Executives might want a high-level summary, while the design team might appreciate more detailed findings and recommendations.