Data is the new gold, but it doesn’t come pre-polished.
For example, let’s take user feedback. It’s raw, messy, and often overwhelming.
One minute you’re reading a glowing review, the next you’re hit with vague complaints that seem impossible to categorize. And yet, somewhere in all that chaos lies the insight that could redefine your strategy or fix a major problem.
This is the reality every researcher faces!
How to analyze qualitative data and turn it into meaningful, actionable takeaways? This is where content analysis comes in.
In this blog, we will explore the different types of content analysis in qualitative research and show you how to pick the right approach that could transform your data into a goldmine. We will also explore modern techniques for analyzing content that will make your research process smoother and faster.
What is qualitative content analysis?
Content analysis in qualitative data is the process of finding patterns, themes, and trends in textual data. No matter if the data is collected from surveys, interviews, or customer feedback, qualitative content analysis helps to get in-depth insights into what users actually want. It’s a systematic procedure to turn raw data into meaningful insights.
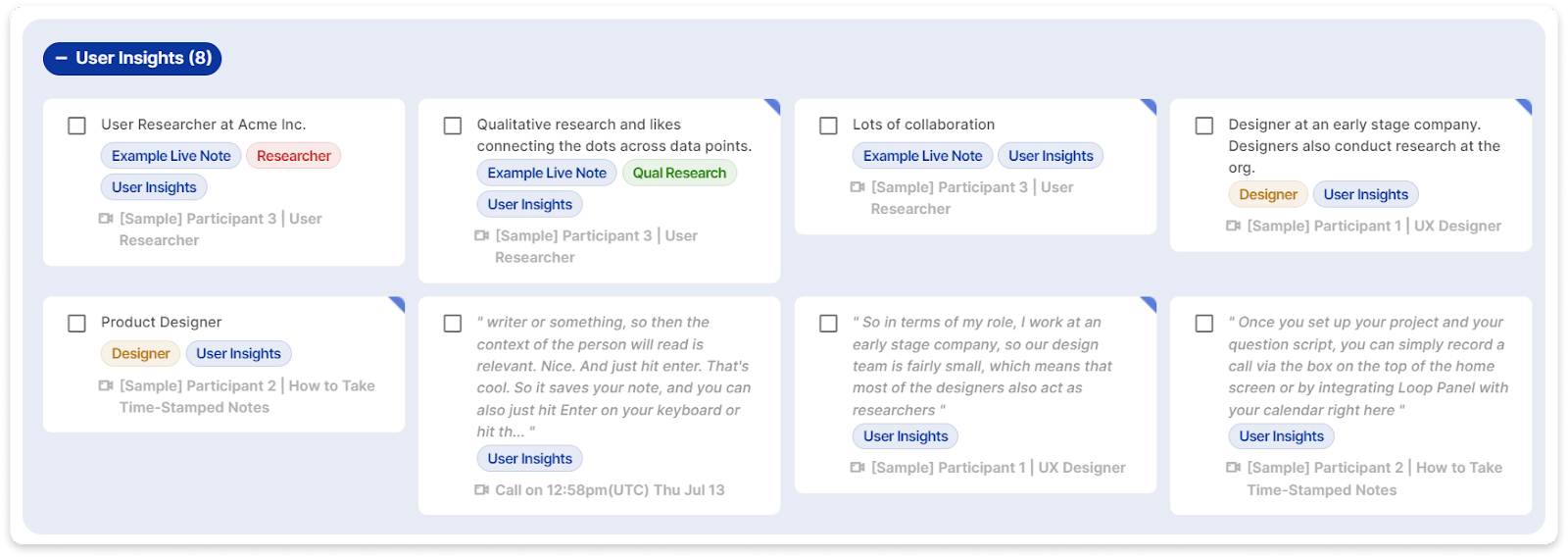
For example, a SaaS business can analyze customer feedback to understand the recurring problems with their onboarding process, measure how often certain concerns appear, and gain deeper insights into why users feel the way they do. This can help the business to tweak its user onboarding process to ensure a seamless experience for users.
Related read: 10 Steps For User Research Analysis
Types of content analysis in qualitative research
There are different qualitative content analysis approaches, each designed to make sense of text data in unique ways. These methods are as follows:
1. Conventional content analysis
Conventional content analysis follows a simple approach where researchers analyze the data without any preconceived category, framework, theme, or pattern.
Instead of analyzing the data with assumptions, researchers allow patterns and themes to emerge organically from the data. This makes it a flexible and unbiased approach that helps derive new insights on unfamiliar topics.
This approach works best when researchers are analyzing a new concept without any prior research or when they want to derive fresh insights. It is extremely helpful when researchers want to go over and beyond what's explicitly stated and find out something fresh.
For example, let's say you just released a beta version of an app and collected some open-ended feedback from early users. You go through responses with no preconceived categorization in mind. Recurring themes come up organically like confusion with navigation, praise for a specific feature, and so on. These insights will then guide you on the areas of improvement. Now if you had set preconceived categories, then you might not be able to uncover all the issues.
2. Directed content analysis
Directed content analysis is based on existing theories, frameworks, or research that will guide the analysis.
Unlike traditional content analysis, this approach starts with pre-established categories or codes that are then applied to the data in order to confirm, modify, or extend existing ideas.
This is the best approach when there's a hypothesis or existing knowledge to be validated or extended. It's especially useful in areas with well-established theories where you want to do something new on top of prior research.
For example, a research team is using a new service to study customer satisfaction. They take the set of established models for customer experience and create categories such as "response time," "service quality," and "ease of use." They map all the feedback into these predefined categories as they analyze the responses from the surveys, identifying the gaps or trends that either prove or disprove the initial theories.
3. Summative content analysis
Summative content analysis is the process of analyzing the frequency of words or phrases to understand their meaning and context. It goes beyond simple counting by exploring how specific terms are used and the sentiment they convey.
This would be good for analyzing sentiment, tone, or underlying context in large datasets. It is especially good for studying trends in social media, reviews, or any other form of user-generated content.
For example, imagine a team analyzing comments on social media related to a product launch. The word frequencies for "love," "confusing," and "expensive" will let them not only identify the most frequent reactions but also make sense of the general sentiment. For example, frequent references to "confusing" together with "instructions" might hint at a need for clearer user guides.
Comparison of content analysis methods
Techniques for effective content analysis
The right technique can either make or break the content analysis process. Depending on the nature of your research, there are strengths and limitations to both manual and AI content analysis techniques. These are as follows:
1. Manual content analysis
Manual content analysis involves hand-coding and categorizing data, which are most frequently accomplished using spreadsheets or hand-written notes. This method provides the researcher with absolute control over the analysis. This approach also gives researchers the opportunity to immerse themselves in the data to observe specific nuances.
Pros
- Detailed understanding of data.
- Accuracy in spotting subtle patterns.
- Flexible for unique or small datasets.
- Promotes critical thinking and interpretation.
Cons
- Very time-consuming, especially for large datasets.
- Prone to human error or bias.
- Difficult to replicate consistently.
- It can be overwhelming for complex or extensive data.
2. Technology-driven tools

Technology-driven tools make it easier to analyze content with the help of AI-powered algorithms. They are designed to handle large data sets efficiently and generate insights with little manual effort.
AI tools for qualitative analysis provide services like transcription, sentiment analysis, tagging, and summarization and are very helpful for a researcher dealing with complex or large data.
Pros
- Processes large volumes of data fast.
- Reduces human effort and error.
- Provides consistent and replicable results.
- Identifies patterns and trends in minutes.
Cons
- It may lack contextual understanding.
- Limited flexibility for nuanced interpretation.
- Dependent on the quality of input data.
- Requires technical expertise for optimal use.
Looppanel is the ultimate tool for qualitative research, designed to ease and increase the productivity of your research work. With its powerful AI-driven features, including over 90%+ accurate transcriptions, automatic thematic tagging, and AI-generated executive summaries, it promises to save researchers hours of tedious tasks.
Rather than keeping researchers engaged in manual sorting and note-taking, Looppanel makes the most relevant findings emerge in a snap, hence making it a must-have for any team that intends to be ahead in the analysis process and drive meaningful insights faster.
Key features of Looppanel:
1. High-quality transcriptions: Obtain over 90%+ accuracy in transcriptions across 17 languages, ensuring a solid foundation for further analysis.

2. AI-powered note-taking: Automatically generates concise, readable notes organized by interview questions or themes, reducing review time by 80%.
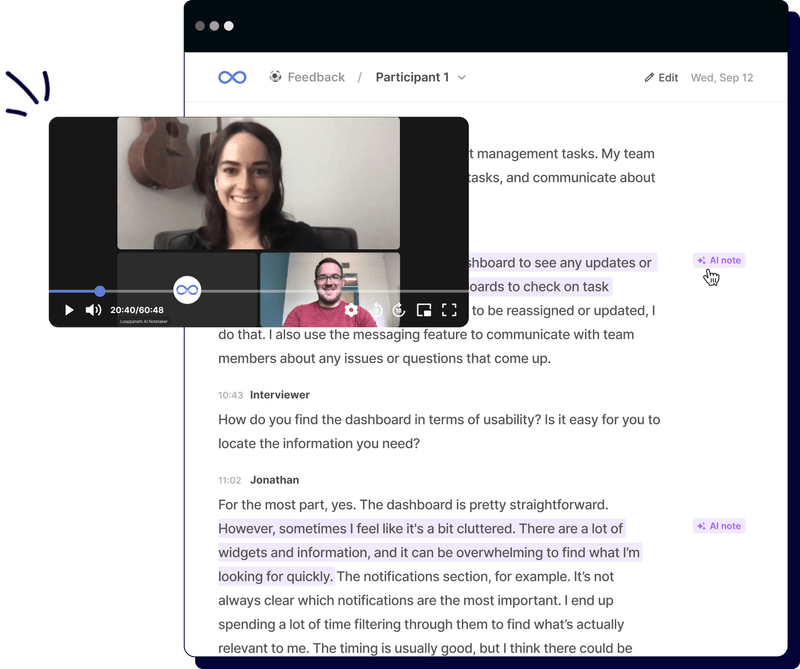
3. Thematic tagging: Looppanel helps with the process of thematic analysis by identifying and grouping themes across data, saving hours of manual effort and allowing researchers to refine as needed.
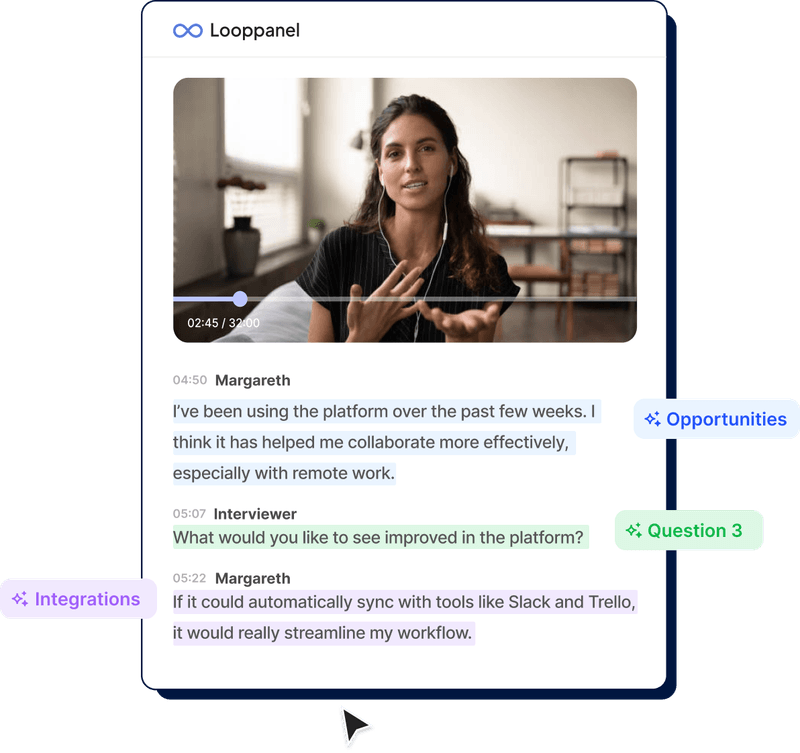
4. AI executive summaries: Instantly share insights through editable, presentation-ready reports with citations and supporting quotes, thereby enhancing communication with team members.

5. Smart search functionality: Find answers and raw data in seconds with intuitive, context-aware queries, complete with source citations for transparency.
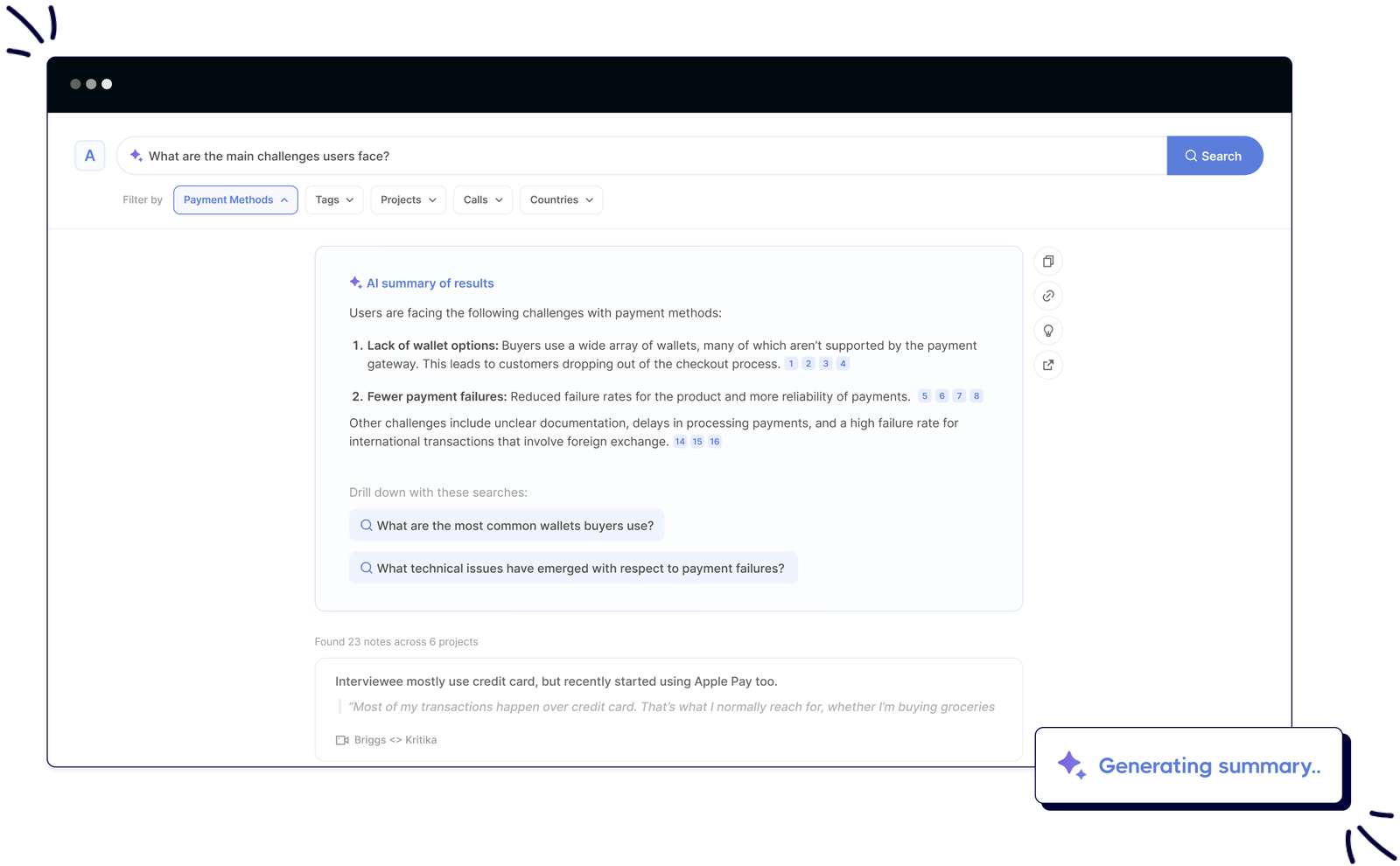
Conclusion
Content analysis makes it easy to summarize a large amount of data by finding the patterns and trends in qualitative data. Whether it's user feedback, interview transcripts, or even social media comments, the key point is to turn that raw data into meaningful insights.
Now, if you’re looking for a tool that simplifies this process and speeds up your analysis, Looppanel is the perfect solution. With its AI-driven features like automatic tagging, smart search, and 90%+ accurate transcription, it makes content analysis faster and more efficient. Want to see it in action?
Book a demo with Looppanel today and experience how it can transform your research workflow.
Frequently asked questions
1. What is content analysis in qualitative research?
Content analysis helps to identify patterns and trends across textual data, enhancing decision-making and strategy.
2. How do I conduct content analysis?
Steps include data collection, coding, categorization, and interpretation of the information collected in order to identify critical themes and trends within your data.
3. How to interpret content analysis results?
Review coded data, identify emerging themes, and analyze patterns to draw insights that can inform decisions and strategies.
4. What is the difference between thematic analysis and content analysis?
Read this blog to understand the differences between Thematic Analysis vs. Content Analysis