User analysis shapes every successful product and service today, from the apps you use daily to the websites you browse. As research methodologies evolve and AI tools transform our capabilities, understanding how to harness user data analysis effectively has never been more crucial – or more exciting.
What is user analysis?
User analysis combines research methodologies and data analytics to deeply understand user behaviors, needs, and motivations. Unlike basic analytics that just track clicks and pageviews, proper user experience analysis creates a complete picture of who your users are and how they interact with your product.
Modern user data analysis involves multiple data streams – from direct user feedback through interviews and surveys to passive tracking of user interactions. For instance, a banking app might combine transaction data, customer service interactions, and user interviews to understand why customers abandon their mobile check deposit feature.
A thorough user analysis looks at both quantitative metrics (like conversion rates and engagement statistics) and qualitative insights (such as user feedback and observed behaviors). This dual approach helps teams understand not just what users do, but why they do it.
The methods for user experience analysis have evolved significantly with technological advances. Traditional methods like surveys and interviews now work alongside AI-powered tools that can process vast amounts of user data and identify patterns human analysts might miss.
Consider this user analysis example. An e-commerce platform noticed high cart abandonment rates. Through comprehensive analysis combining user session recordings, checkout funnel data, and customer interviews, they discovered mobile users struggled with a complex shipping calculator. This insight led to a streamlined mobile checkout process that boosted conversions by 25%.
Different types of user analysis
Behavioral analytics
Behavioral analytics forms the foundation of user analysis. This approach tracks and analyzes actual user actions within your product or service. It reveals navigation patterns, feature adoption rates, and common user journeys. Behavioral analysis helps identify where users succeed and where they encounter friction.
Attitudinal research
Attitudinal research digs deeper into user motivations and preferences. Through surveys, interviews, and feedback analysis, teams can understand user sentiment, satisfaction levels, and emotional responses to different features. This type of user data analysis often reveals surprising insights about why users choose certain features over others.
Contextual analysis
Contextual analysis examines how environmental factors influence user behavior. By studying users in their natural settings, researchers can understand external influences on product usage. For example, a food delivery app might discover that busy parents often order while multitasking, leading to simplified ordering flows.
Predictive analysis
Predictive analysis uses historical user data and machine learning to forecast future user behaviors. This advanced form of user experience analysis helps teams anticipate user needs and proactively address potential issues before they impact user satisfaction.
Segmentation analysis
Segmentation analysis divides users into meaningful groups based on behavior patterns, demographics, or other characteristics. This method helps teams tailor experiences for different user types and prioritize improvements for key user segments.
When to perform user analysis
User analysis shouldn't be a one-time event. The most successful companies weave it into their regular development cycle, creating a continuous feedback loop that drives improvement. The landscape of user behavior shifts constantly – what worked six months ago might not resonate today.
- The pre-launch phase demands extensive user analysis to validate assumptions and prevent costly mistakes. Smart companies start user research before writing a single line of code, using prototypes and concepts to gauge user interest and identify potential pitfalls.
- During active development, user analysis becomes your compass. Each sprint should incorporate user feedback and behavioral data, helping teams adjust course based on real user needs rather than assumptions. Companies that skip this step often find themselves rebuilding features that users never wanted in the first place.
- Post-launch analysis is equally crucial but often overlooked. The real test begins when users interact with your product in the wild. Regular analysis helps catch emerging issues, identify unexpected use cases, and spot opportunities for improvement that weren't visible during development.
Why is user analysis important for different teams?
Product teams rely on user analysis to make decisions that actually matter to users. Instead of building features based on hunches or the loudest voice in the room, they can prioritize improvements that data shows will have the biggest impact. A gaming company might discover through user analysis that players care more about quick load times than fancy graphics.
Design teams transform raw user data into intuitive interfaces. They use heat maps, user recordings, and feedback to create experiences that feel natural and effortless. The best designers combine user analysis with creativity – using data to inform decisions while maintaining innovative thinking.
Marketing teams leverage user insights to craft messages that truly resonate. Understanding user behavior patterns helps them target the right audiences with the right messages at the right time. A software company might learn that their users care more about time savings than technical features, completely reshaping their marketing approach.
Support teams use analysis to get ahead of user problems. By understanding common pain points and usage patterns, they can create better documentation, improve self-service options, and reduce ticket volumes. This proactive approach leads to happier users and more efficient support operations.
How to do user analysis in 6 steps
Step 1: Begin with personas
Start by creating detailed user personas based on real data, not assumptions. These personas should capture not just demographics but also goals, frustrations, and behavioral patterns. Modern analysis tools can help identify distinct user segments automatically, making persona creation more accurate than ever.
Step 2: User journey analysis—mapping out users
Map the complete user journey, from first contact to long-term engagement. Use analytics tools to track how users actually move through your product, not just how you think they should. Pay special attention to drop-off points and unexpected paths – they often reveal the most valuable insights.
Step 3: Product design, creation, and implementation
Transform insights into action by incorporating user analysis into your design process. Create prototypes based on user data, test them with real users, and iterate based on feedback. This cycle of design, test, and refine helps ensure your final product truly meets user needs.
Step 4: Set research goals and recruit users
Establish clear research objectives tied to business goals. Be specific about what you want to learn and why it matters. Here’s how you can build a research plan that actually works.
When recruiting users, look beyond convenience samples to find participants who truly represent your target audience. Recruitment tools like User Interviews and Respondent can help you find the right users quickly and efficiently.
Step 5: Conduct user research (interviews)
Conduct structured interviews that dig deep into user needs and behaviors. Record sessions and use AI-powered tools to transcribe and analyze conversations automatically. This approach helps you capture nuanced insights while maintaining efficiency. Remember to let users lead the conversation – sometimes the most valuable insights come from unexpected directions.
Step 6: Analyze and apply your findings
Start by organizing all your research materials in one place – recordings, transcripts, notes, and observations. This step alone can feel overwhelming, especially when dealing with hours of user interviews. Tools like Looppanel can streamline this process by automatically generating highly accurate transcripts and AI-powered notes, cutting down analysis time by up to 80%.

Next, look for patterns and recurring themes across your user data. While traditional methods involve manual coding and categorization, modern AI-powered platforms can identify themes automatically. Looppanel uses advanced AI to tag important moments and group similar insights, creating visual affinity maps that make pattern recognition intuitive and fast.
Your analysis should combine both qualitative and quantitative approaches.
- Qualitative analysis digs into the 'why' behind user behaviors. It examines user stories, emotions, and motivations. Modern tools can help process hundreds of pages of transcripts in minutes, identifying sentiment patterns and key themes that might take weeks to spot manually.
- Quantitative analysis tackles the numbers – usage patterns, feature adoption rates, and other measurable metrics. This data helps validate qualitative insights and provide concrete evidence for decision-making.
The end goal is to create a clear narrative that combines solid evidence with actionable recommendations. Modern analysis tools help you get there faster, but the human element – your expertise in interpreting and applying these insights – remains crucial for success.
How Looppanel speeds up user data analysis
Looppanel is a UX research analysis & repository solution for the modern UX team. Looppanel helps you analyze research data 10x faster, centralize feedback in one searchable hub, and surface insights in seconds.
Built for modern teams, Looppanel transforms scattered user interviews, reports, and more into a centralized insights hub where customer feedback becomes clear, actionable, and impossible to ignore.
If you’re running user analysis, here are some exciting ways Looppanel can help you out.
AI tagging
Traditional thematic analysis often means hours of manually sorting through transcripts and notes, trying to spot patterns. Looppanel's AI thematic tagging automatically identifies themes across your research files, instantly organizing insights into relevant categories. Even better, these themes are always traceable back to source quotes, maintaining research integrity while saving countless hours of manual work.

While the AI gets you 80% of the way there with automatic tagging, you maintain full control – easily editing or adjusting tags to match your specific research context. This human-in-the-loop approach combines efficiency with accuracy.
Executive AI-Summaries

Instead of spending days crafting the perfect report, Looppanel's AI Executive Summary feature generates shareable takeaways immediately after your study ends. These summaries include:
- Key findings supported by direct user quotes
- Clear, presentation-ready formatting
- Editable content for fine-tuning
Smart Search
Gone are the days of ctrl+F hunting through endless documents. Looppanel's smart search understands context and intent, letting you ask natural questions like "What frustrates users about our checkout process?" and getting comprehensive answers backed by actual user quotes and data points.
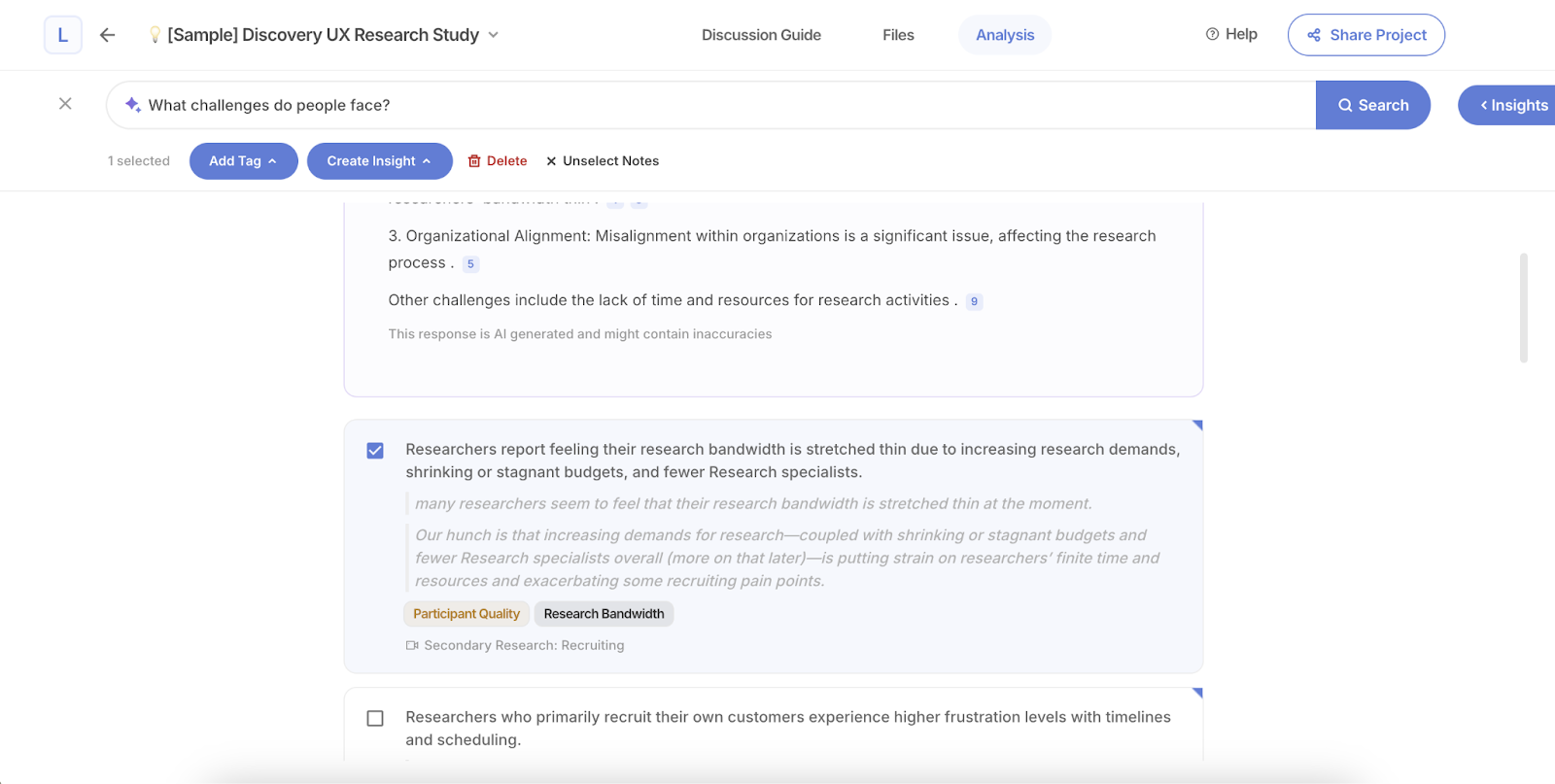
This capability works across your entire research repository, making it easy to:
- Find specific insights in seconds
- Connect patterns across multiple studies
- Support findings with direct evidence
Processing Qualitative Data at Scale
When faced with hundreds of open-ended survey responses or user reviews, manual analysis becomes nearly impossible. Looppanel's AI analysis processes vast amounts of qualitative data in minutes, delivering:
- Summarized insights from thousands of responses
- Citation counts for each finding
- Clear evidence trails for verification
- Actionable patterns and trends
Excited to see how Looppanel can make your research faster? Try it out now.
Frequently Asked Questions (FAQs)
What is user data analysis?
User data analysis is the systematic examination of how people interact with your product or service. It goes beyond basic metrics to uncover behavioral patterns, pain points, and opportunities for improvement. Modern user data analysis combines traditional research methods with AI-powered tools to process vast amounts of user data quickly and effectively.
Think of user analysis as solving a complex puzzle – each data point adds to the complete picture of your users' needs, behaviors, and motivations. Today's user experience analysis tools can process thousands of data points in minutes, giving researchers unprecedented insight into user behavior.
What does a user experience analyst do?
A user experience analyst combines data analysis with human insight to improve product usability and satisfaction. They conduct user needs analysis, run usability tests, analyze behavioral data, and translate findings into actionable recommendations. Their work bridges the gap between user intent analysis and product development.
How do you write a user analysis?
Writing effective user analysis starts with clear research objectives and methodical data collection. Start by defining your goals, gathering both qualitative and quantitative data, and organizing findings into clear themes. A good user analysis example might combine interview insights, usage metrics, and behavioral patterns to tell a compelling story about user needs.
What are the 4 types of Data Analysis?
The four fundamental types are descriptive (what happened), diagnostic (why it happened), predictive (what might happen), and prescriptive (what should be done). Modern user experience analysis often combines all four types to create comprehensive insights about user behavior and needs.
What is user need analysis?
User needs analysis is a structured approach to understanding what users want to accomplish and why. This process combines direct user feedback with behavioral data to identify both explicit and implicit needs. Good user analysis includes thorough investigation of user needs through interviews, surveys, and observational research.
What is user role analysis?
User role analysis examines how different user types interact with your product. This specialized form of user analysis helps teams understand various user personas and their specific needs. It's particularly valuable for products serving multiple user types with different goals.
What is user data analytics?
User data analytics combines quantitative metrics with qualitative insights to understand user behavior comprehensively. Modern user data analysis tools can process vast amounts of data from multiple sources, identifying patterns that might be invisible to human observers alone.
What is data analysis in UX?
UX data analysis focuses on understanding how users interact with and experience your product. This specialized form of user experience analysis combines behavioral metrics, usability testing results, and user feedback to improve product design and functionality.
What is an example of user experience analysis?
A practical user analysis example might be analyzing how users navigate an e-commerce checkout process. By combining heatmap data, user interviews, and conversion metrics, analysts can identify friction points and opportunities for improvement. This kind of user intent analysis helps teams make data-driven design decisions.
What are the 5 elements of user experience?
The five elements are strategy (product objectives), scope (functional requirements), structure (information architecture), skeleton (interface design), and surface (visual design). Each element builds upon the others to create a complete user experience analysis framework.
What are the 4 C's of user experience?
The four C's – content, context, users (consumers), and competition – form a framework for comprehensive user needs analysis. This framework helps teams consider all aspects that influence user experience, from content quality to competitive landscape.